Learning-to-augment incorporated noise-robust deep CNN for detection of COVID-19 in noisy X-ray images
Journal of Computational Science(2022)
摘要
Deep convolutional neural networks (CNNs) are used for the detection of COVID-19 in X-ray images. The detection performance of deep CNNs may be reduced by noisy X-ray images. To improve the robustness of a deep CNN against impulse noise, we propose a novel CNN approach using adaptive convolution, with the aim to ameliorate COVID-19 detection in noisy X-ray images without requiring any preprocessing for noise removal. This approach includes an impulse noise-map layer, an adaptive resizing layer, and an adaptive convolution layer to the conventional CNN framework. We also used a learning-to-augment strategy using noisy X-ray images to improve the generalization of a deep CNN. We have collected a dataset of 2093 chest X-ray images including COVID-19 (452 images), non-COVID pneumonia (621 images), and healthy ones (1020 images). The architecture of pre-trained networks such as SqueezeNet, GoogleNet, MobileNetv2, ResNet18, ResNet50, ShuffleNet, and EfficientNetb0 has been modified to increase their robustness to impulse noise. Validation on the noisy X-ray images using the proposed noise-robust layers and learning-to-augment strategy-incorporated ResNet50 showed 2% better classification accuracy compared with state-of-the-art method.
更多查看译文
关键词
Noise,Adaptive resize,Adaptive convolution,Data augmentation,COVID-19 classification
AI 理解论文
溯源树
样例
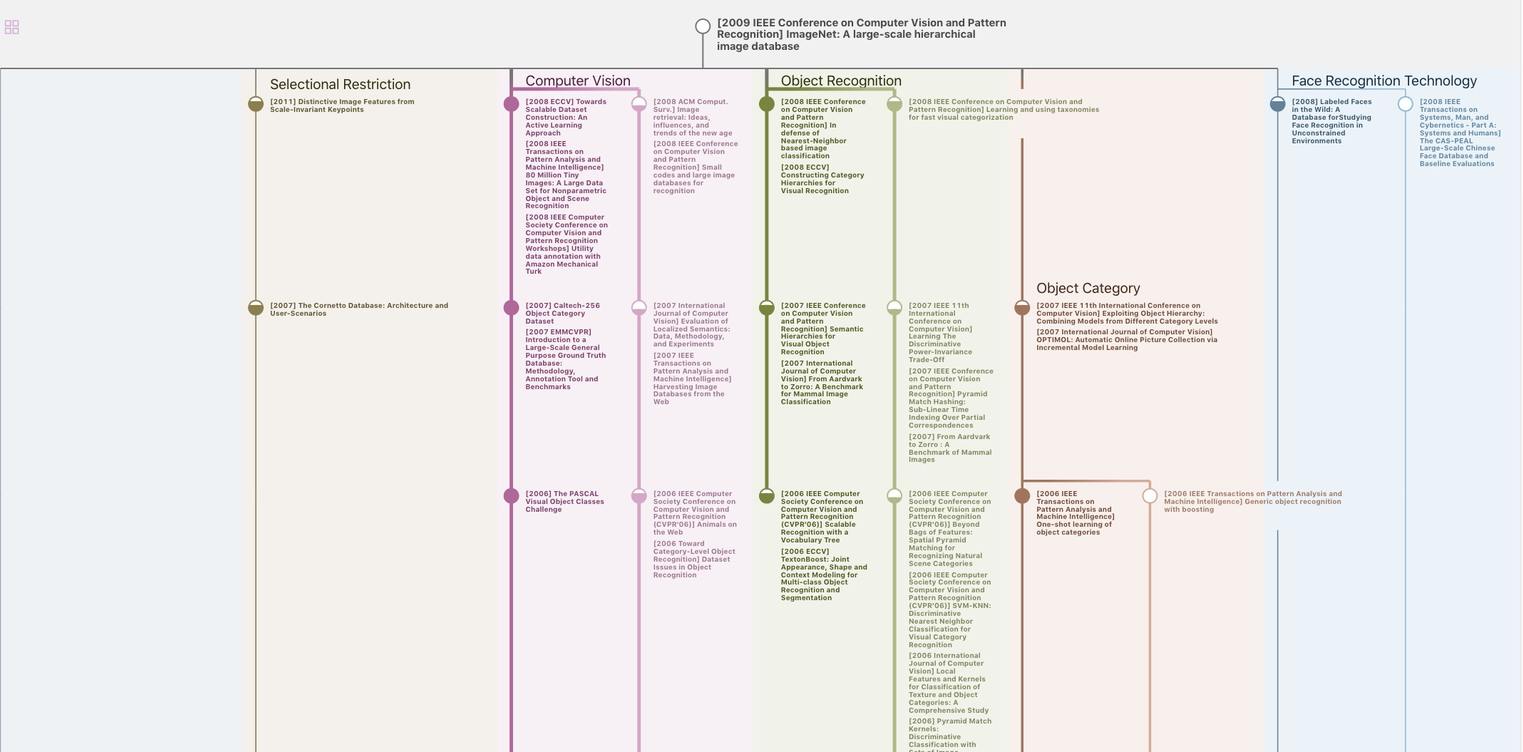
生成溯源树,研究论文发展脉络
Chat Paper
正在生成论文摘要