A Robust Computerized Adaptive Testing Approach in Educational Question Retrieval
SIGIR '22: Proceedings of the 45th International ACM SIGIR Conference on Research and Development in Information Retrieval(2022)
摘要
Computerized Adaptive Testing (CAT) is a promising testing mode in personalized online education (e.g., GRE), which aims at measuring student's proficiency accurately and reducing test length. The "adaptive" is reflected in its selection algorithm that can retrieve best-suited questions for student based on his/her estimated proficiency at each test step. Although there are many sophisticated selection algorithms for improving CAT's effectiveness, they are restricted and perturbed by the accuracy of current proficiency estimate, thus lacking robustness. To this end, we investigate a general method to enhance the robustness of existing algorithms by leveraging student's "multi-facet" nature during tests. Specifically, we present a generic optimization criterion Robust Adaptive Testing (RAT) for proficiency estimation via fusing multiple estimates at each step, which maintains a multi-facet description of student's potential proficiency. We further provide theoretical analyses of such estimator's desirable statistical properties: asymptotic unbiasedness, efficiency, and consistency. Extensive experiments on perturbed synthetic data and three real-world datasets show that selection algorithms in our RAT framework are robust and yield substantial improvements.
更多查看译文
关键词
computerized adaptive testing, educational resource search, educational measurement, cognitive diagnosis
AI 理解论文
溯源树
样例
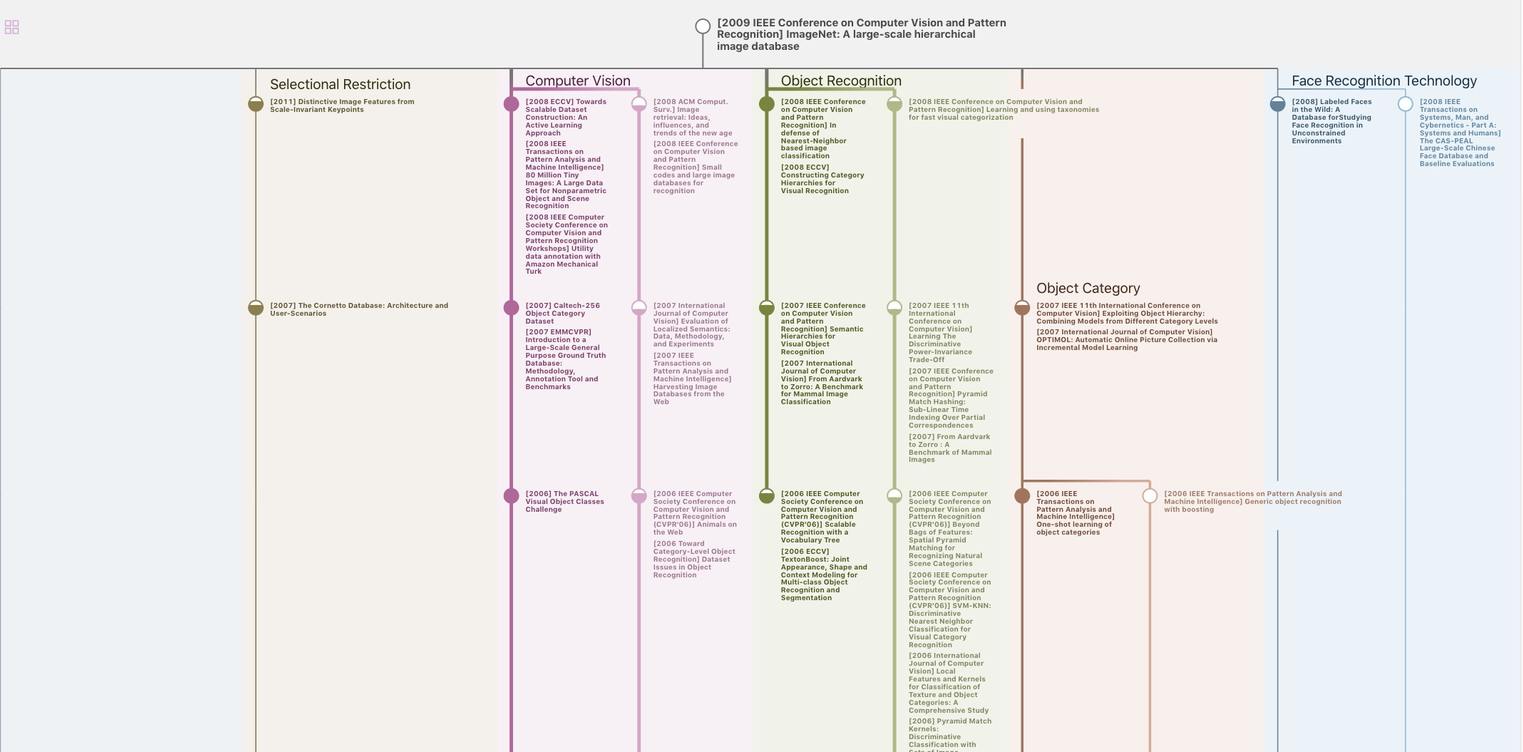
生成溯源树,研究论文发展脉络
Chat Paper
正在生成论文摘要