Computer Vision and Multi-Object Tracking for Traffic Measurement from Campus Monitoring Cameras
Computing in Civil Engineering 2021(2022)
摘要
Conventional methods of traffic monitoring primarily rely on sensors, on-board GPS, and human observation, which are generally resource-intensive, slow, and subject to implementation limits. Computer vision (particularly convolutional neural networks) is a more flexible, convenient, and cost-effective alternative to extract traffic information from traffic videos. This paper presents a methodology to extract traffic volume counts from cameras on Texas A&M University (TAMU) campus. Particularly, an object detector model, namely YOLOv5 (You Only Look Once), is integrated with an object tracking algorithm (Deep-SORT) to track vehicles in camera views. These tracks are projected using homography transformation from the local coordinate system of the camera onto an orthogonal map (world coordinates) along with information such as unique vehicle ID, type, position, and time stamp. This data is used to count vehicles that cross the pre-defined study zones (lanes) on different directions. A total of 60 samples (each 1 minute long) recorded during a 24-h period on TAMU campus are manually annotated and used to benchmark model predictions, resulting in a traffic volume count accuracy ranging from 68% to 93%. Moreover, the best input pixel resolution is determined to be 1,280 x 1,280 by measuring the average precision for each class of interest.
更多查看译文
AI 理解论文
溯源树
样例
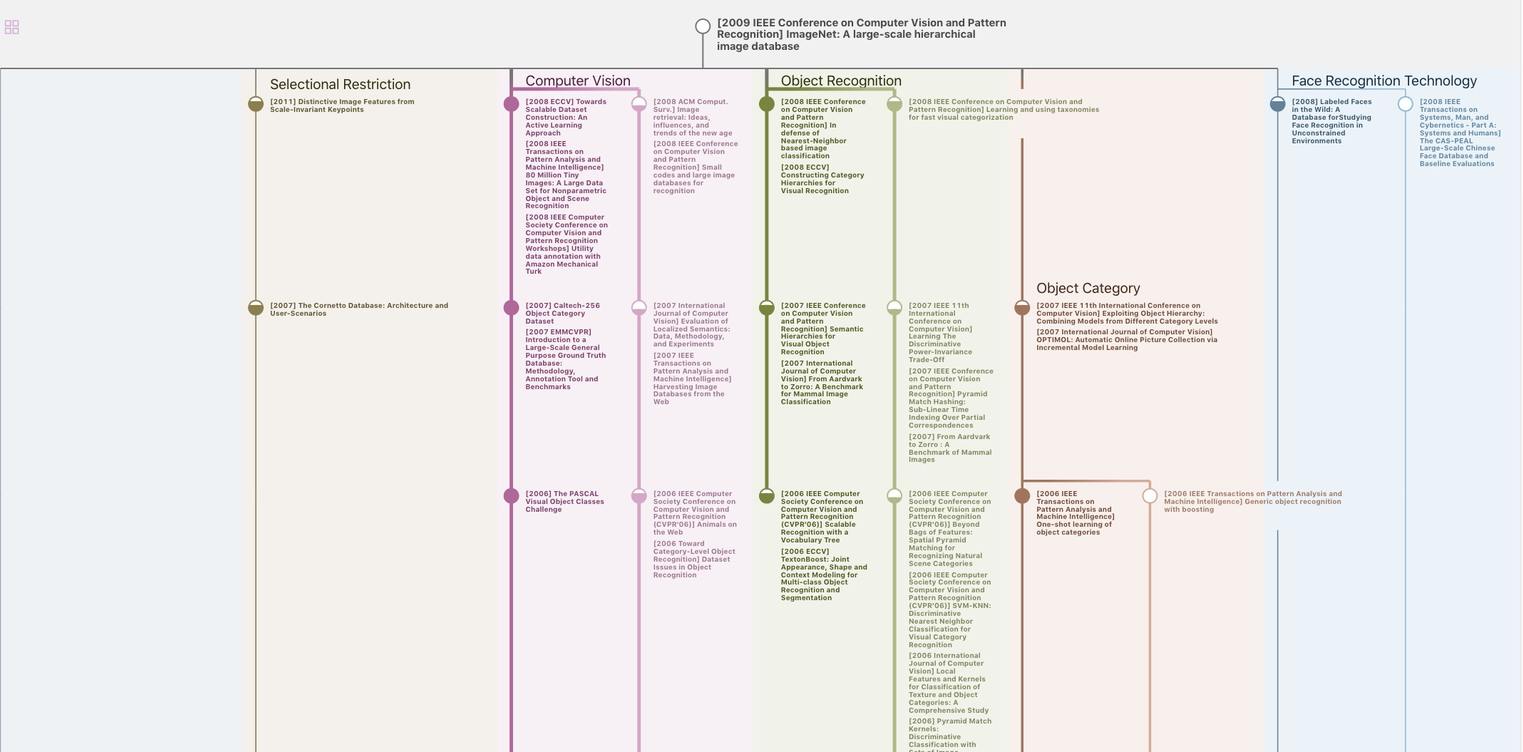
生成溯源树,研究论文发展脉络
Chat Paper
正在生成论文摘要