A law of adversarial risk, interpolation, and label noise
ICLR 2023(2022)
摘要
In supervised learning, it has been shown that label noise in the data can be interpolated without penalties on test accuracy. We show that interpolating label noise induces adversarial vulnerability, and prove the first theorem showing the relationship between label noise and adversarial risk for any data distribution. Our results are almost tight if we do not make any assumptions on the inductive bias of the learning algorithm. We then investigate how different components of this problem affect this result including properties of the distribution. We also discuss non-uniform label noise distributions; and prove a new theorem showing uniform label noise induces nearly as large an adversarial risk as the worst poisoning with the same noise rate. Then, we provide theoretical and empirical evidence that uniform label noise is more harmful than typical real-world label noise. Finally, we show how inductive biases amplify the effect of label noise and argue the need for future work in this direction.
更多查看译文
关键词
label noise,adversarial robustness,lower bound,robust machine learning
AI 理解论文
溯源树
样例
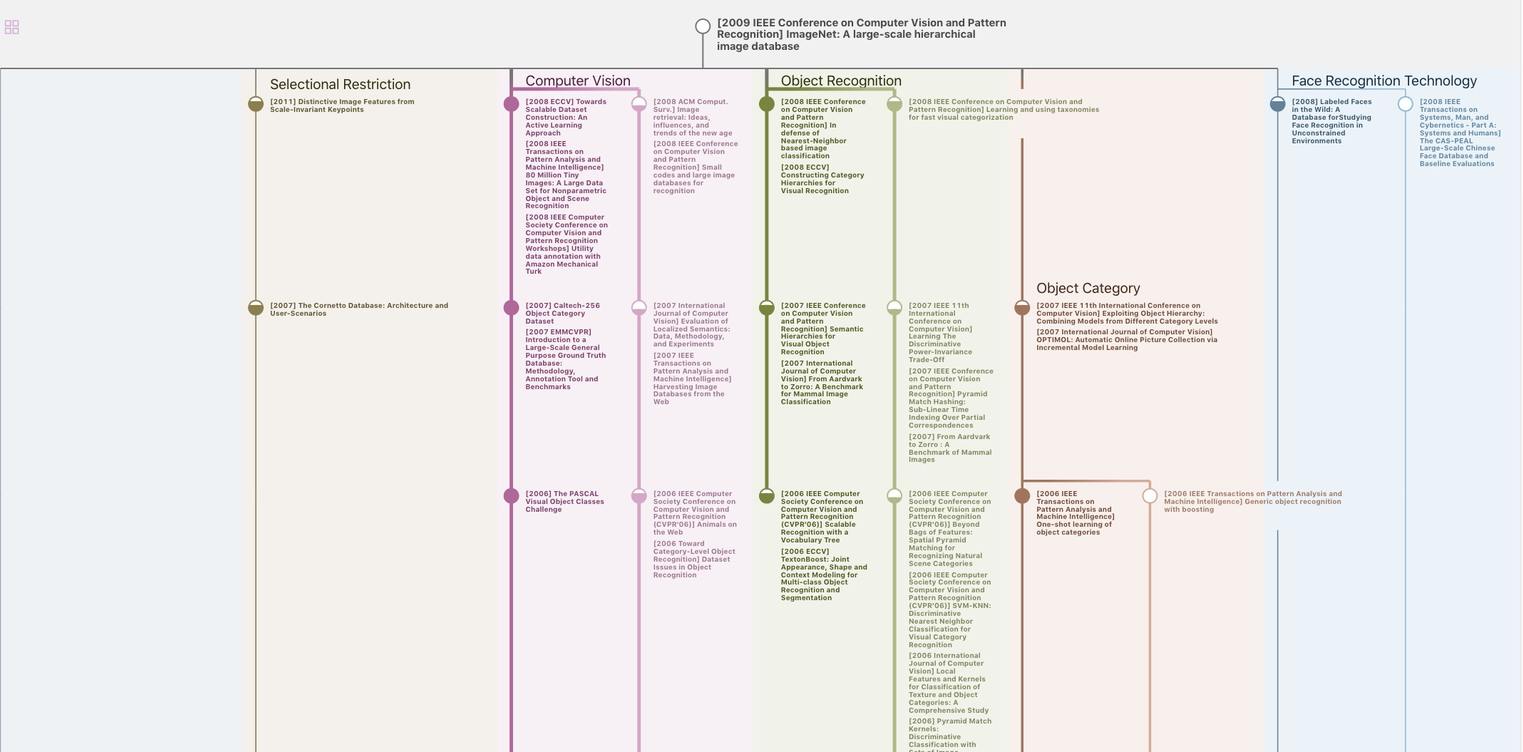
生成溯源树,研究论文发展脉络
Chat Paper
正在生成论文摘要