Pixel-level Correspondence for Self-Supervised Learning from Video
CoRR(2022)
Abstract
While self-supervised learning has enabled effective representation learning in the absence of labels, for vision, video remains a relatively untapped source of supervision. To address this, we propose Pixel-level Correspondence (PiCo), a method for dense contrastive learning from video. By tracking points with optical flow, we obtain a correspondence map which can be used to match local features at different points in time. We validate PiCo on standard benchmarks, outperforming self-supervised baselines on multiple dense prediction tasks, without compromising performance on image classification.
MoreTranslated text
Key words
correspondence,learning,video,pixel-level,self-supervised
AI Read Science
Must-Reading Tree
Example
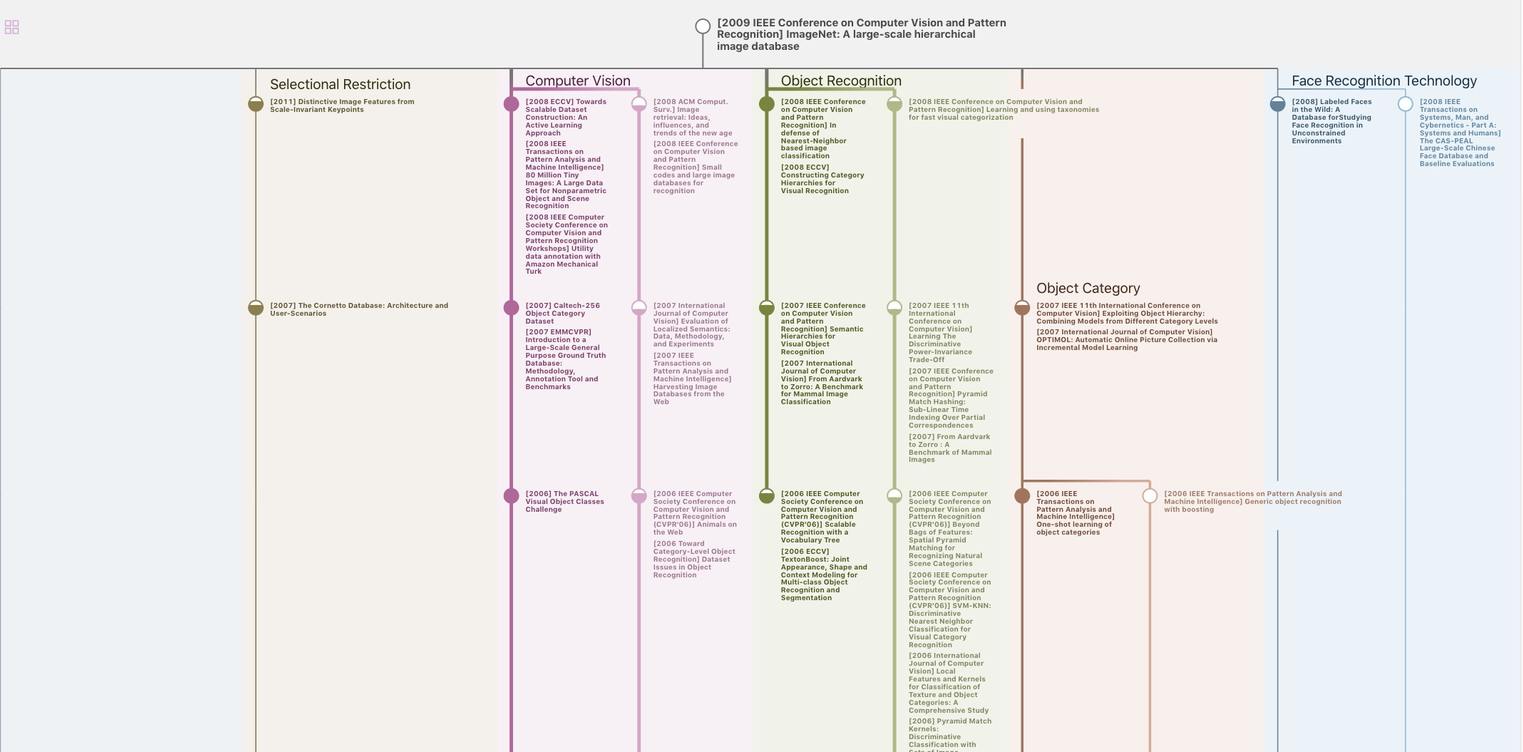
Generate MRT to find the research sequence of this paper
Chat Paper
Summary is being generated by the instructions you defined