Client-Side Optimization Strategies for Communication-Efficient Federated Learning
IEEE Communications Magazine(2022)
摘要
Federated learning (FL) is a swiftly evolving field within machine learning for collaboratively training models at the network edge in a privacy-preserving fashion, without training data leaving the devices where it was generated. The privacy-preserving nature of FL shows great promise for applications with sensitive data such as healthcare, finance, and social media. However, there are barriers to real-world FL at the wireless network edge, stemming from massive wireless parallelism and the high communication costs of model transmission. The communication cost of FL is heavily impacted by the heterogeneous distribution of data across clients, and some cutting-edge works attempt to address this problem using novel client-side optimization strategies. In this article, we provide a tutorial on model training in FL, and survey the recent developments in client-side optimization and how they relate to the communication properties of FL. We then perform a set of comparison experiments on a representative subset of these strategies, gaining insights into their communication-convergence trade-offs. Finally, we highlight challenges to client-side optimization and provide suggestions for future developments in FL at the wireless edge.
更多查看译文
关键词
communication-efficient federated learning,machine learning,collaboratively training models,privacy-preserving fashion,training data,privacy-preserving nature,sensitive data,real-world FL,wireless network edge,massive wireless parallelism,high communication costs,model transmission,communication cost,cutting-edge works,novel client-side optimization strategies,model training,communication properties,communication-convergence trade-offs,wireless edge
AI 理解论文
溯源树
样例
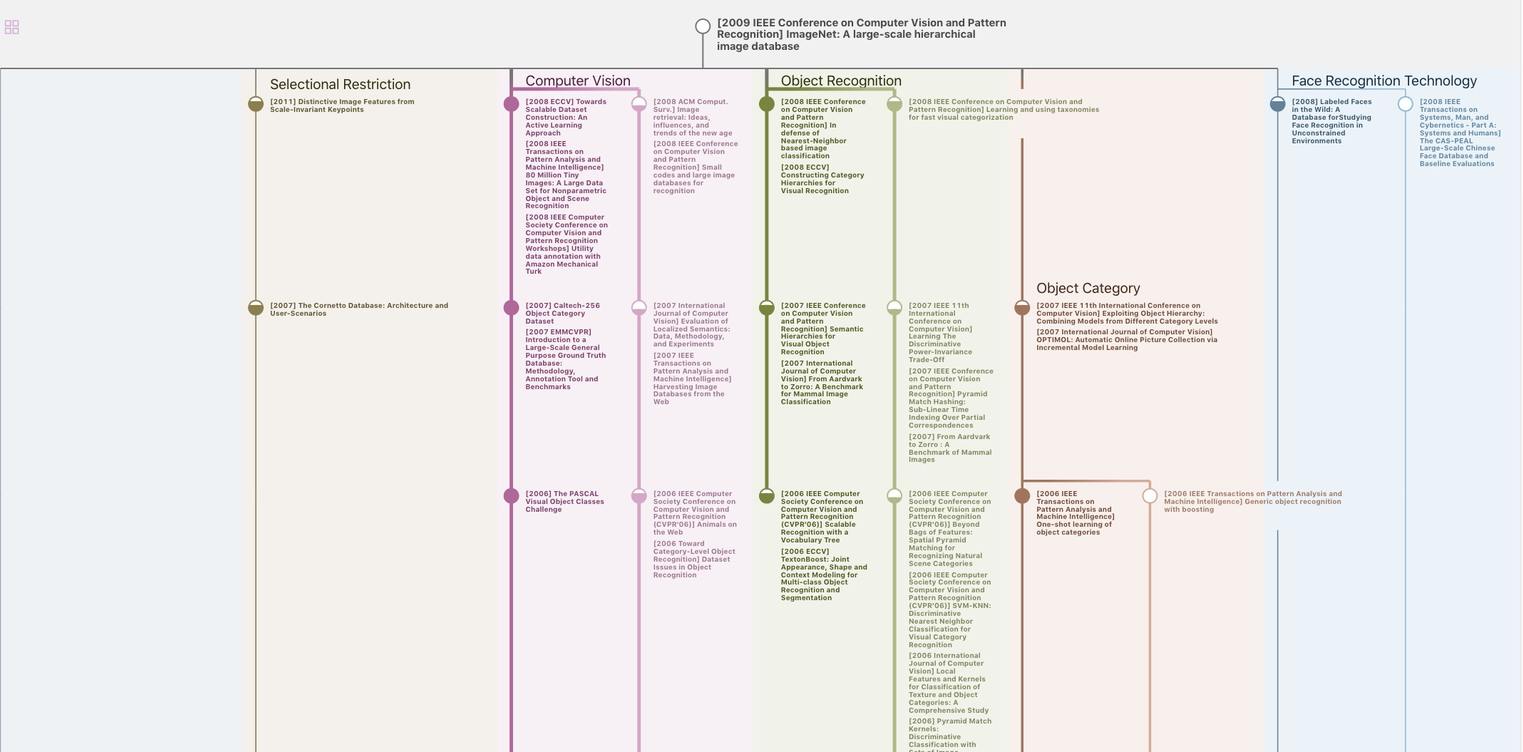
生成溯源树,研究论文发展脉络
Chat Paper
正在生成论文摘要