Research on improved ant colony optimization for traveling salesman problem
MATHEMATICAL BIOSCIENCES AND ENGINEERING(2022)
摘要
As one of the most popular combinatorial optimization problems, Traveling Salesman Problem (TSP) has attracted lots of attention from academia since it was proposed. Numerous meta heuristics and heuristics have been proposed and used to solve the TSP. Although Ant Colony Optimization (ACO) is a natural TSP solving algorithm, in the process of solving it, there are also some shortcomings such as slow convergence speed and prone to fall into local optimum. Therefore, this paper proposes an improved ant colony optimization based on graph convolutional network: Graph Convolutional Network Improved Ant Colony Optimization (GCNIACO). The graph convolutional network is introduced to generate a better solution, and the better solution is converted into the pheromone on the initial path of the ACO. Thereby, the guiding effect of the pheromone concentration for the ants at the beginning of the algorithm is enhanced. In the meantime, through adaptive dynamic adjustment of the pheromone volatility factor and the introduction of the 3-opt algorithm, the algorithm???s ability to jump out of the local optimum is enhanced. Finally, GCNIACO is simulated on TSP datasets and engineering application example. Comparing the optimization results with other classical algorithms, it is verified that the graph convolutional network improved ant colony optimization has better performance in obtaining the optimal solution.
更多查看译文
关键词
traveling salesman problem, ant colony optimization, graph convolutional network, dynamic pheromone volatility factor, 3-opt algorithm
AI 理解论文
溯源树
样例
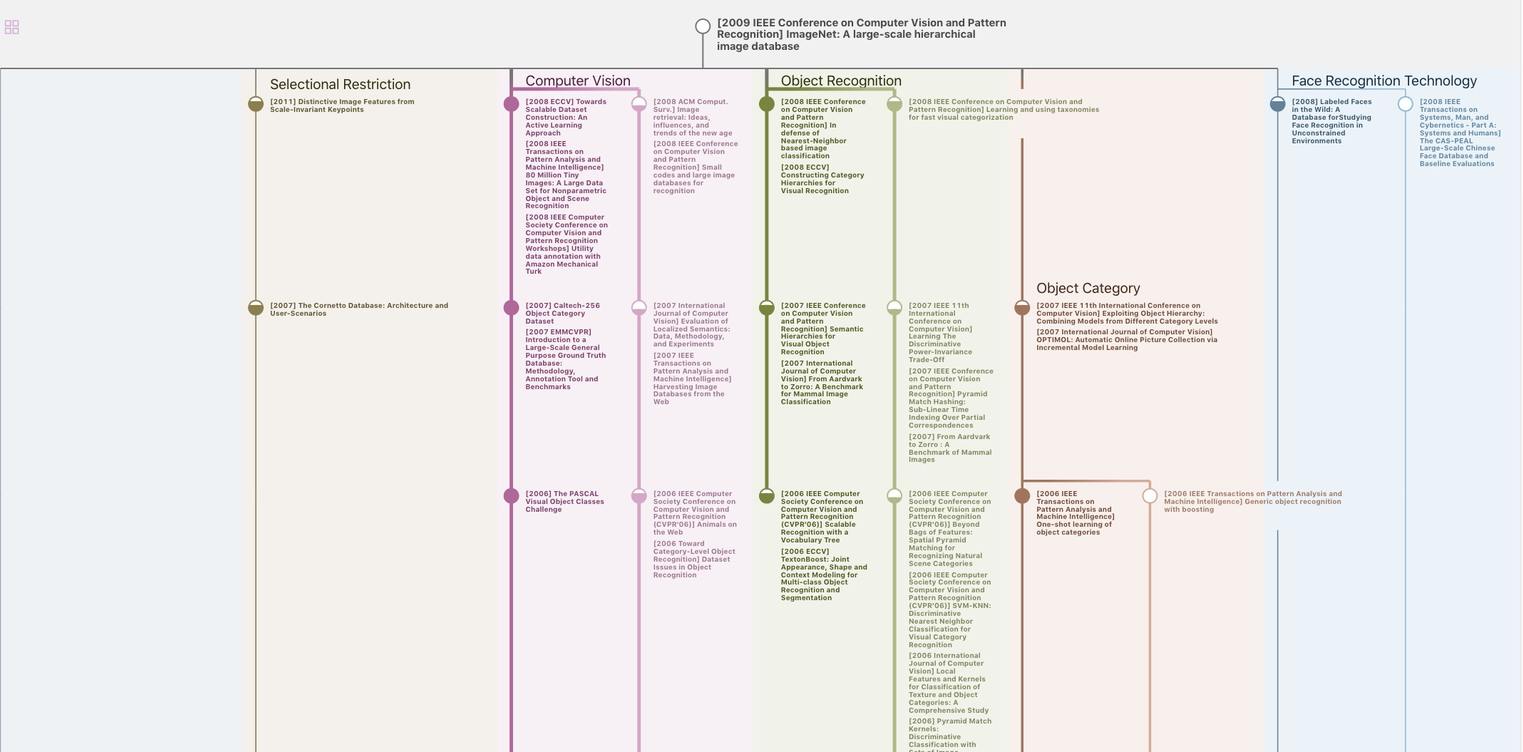
生成溯源树,研究论文发展脉络
Chat Paper
正在生成论文摘要