Multi-Objective Optimization of Building Environmental Performance: An Integrated Parametric Design Method Based on Machine Learning Approaches
SSRN Electronic Journal(2022)
摘要
Reducing energy consumption while providing a high-quality environment for building occupants has become an important target worthy of consideration in the pre-design stage. A reasonable design can achieve both better performance and energy conservation. Parametric design tools show potential to integrate performance simulation and control elements into the early design stage. The large number of design scheme iterations, however, increases the computational load and simulation time, hampering the search for optimized solutions. This paper proposes an integration of parametric design and optimization methods with performance simulation, machine learning, and algorithmic generation. Architectural schemes were modeled parametrically, and numerous iterations were generated systematically and imported into neural networks. Generative Adversarial Networks (GANs) were used to predict environmental performance based on the simulation results. Then, multi-object optimization can be achieved through the fast evolution of the genetic algorithm binding with the database. The test case used in this paper demonstrates that this approach can solve the optimization problem with less time and computational cost, and it provides architects with a fast and easily implemented tool to optimize design strategies based on specific environmental objectives.
更多查看译文
关键词
building performance simulation,machine learning,multi-objective optimization,parametric design,genetic algorithm
AI 理解论文
溯源树
样例
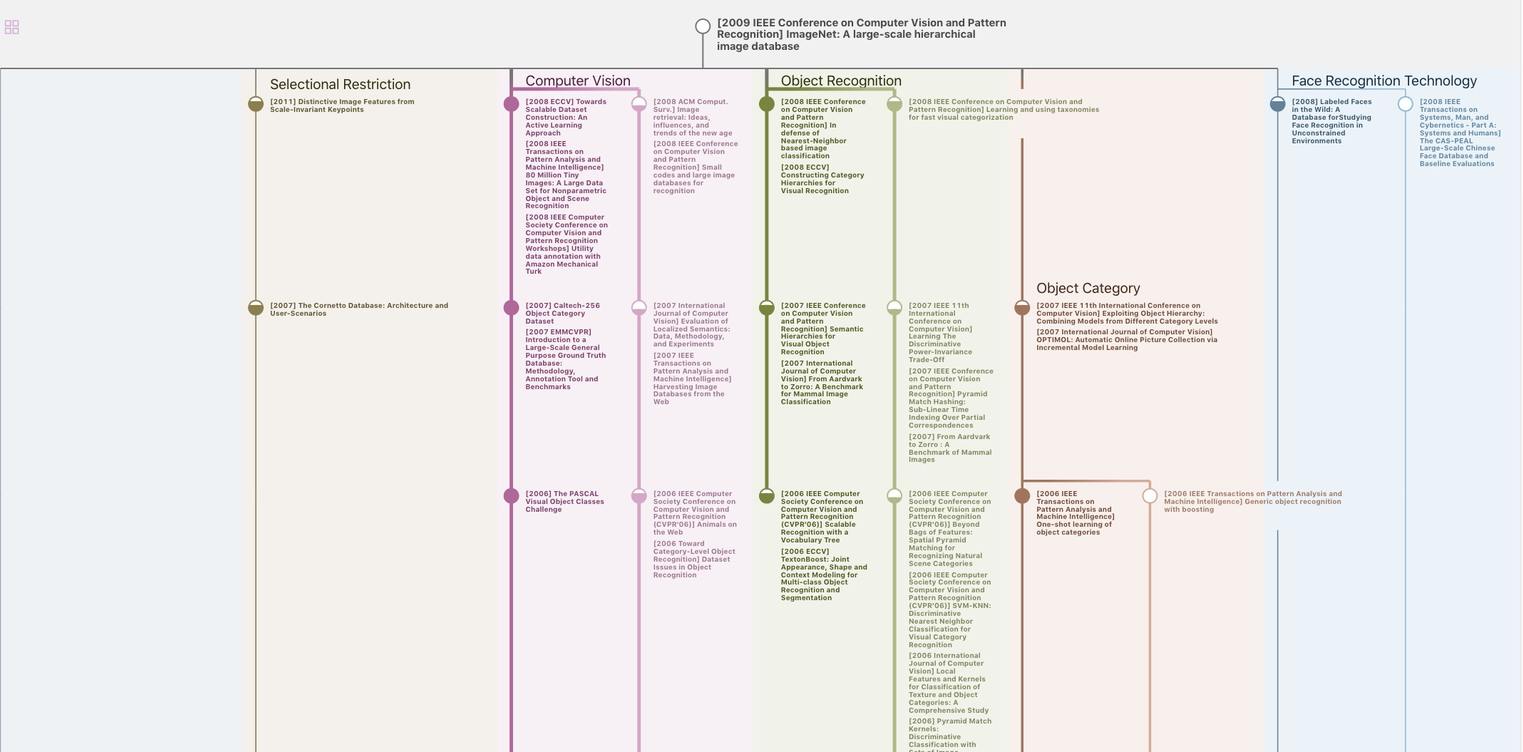
生成溯源树,研究论文发展脉络
Chat Paper
正在生成论文摘要