Sparse Representation Classifier Guided Grassmann Reconstruction Metric Learning With Applications to Image Set Analysis.
IEEE Transactions on Multimedia(2023)
摘要
Modeling a sequence of video frames as a linear subspace on Grassmann manifold has recently become increasingly attractive in multiple computer vision applications. The success of such algorithms largely depends on a good distance measure, and learning an appropriate metric on Grassmann manifold remains a key challenge. Existing works address this by learning a discriminative mapping from the original Grassmann manifold to Hilbert space or a lower-dimensional, more discriminative Grassmann manifold. However, these approaches always highly rely on nearest neighbor matching of samples on the projected space, which is sensitive to noises and errors. Different from them, this paper proposes a Grassmann Reconstruction Metric Learning (GRML) algorithm guided by sparse representation-based classifier (SRC) for image set classification. SRC selects the coefficients associated with each class to reconstruct training samples, and then we employ it as a criterion to direct the design of a discriminant metric on Grassmann manifold. Specifically, GRML attempts to jointly maximize the inter-class reconstruction residual and minimize the intra-class reconstruction residual in the lower but more discriminative Grassmann manifold. To further explore the intrinsic geometry distance, we present a Grassmann Reconstruction Multiple Kernel Metric Learning (GRMKML) algorithm, which aims to jointly learn a metric and the corresponding kernel from a family of kernels for Grassmann manifold. Extensive experiments on eight benchmark datasets demonstrate that the proposed algorithms perform favorably against the state-of-the-art methods.
更多查看译文
关键词
Grassmann manifold,image set classification,multiple kernel learning,sparse representation-based classifier
AI 理解论文
溯源树
样例
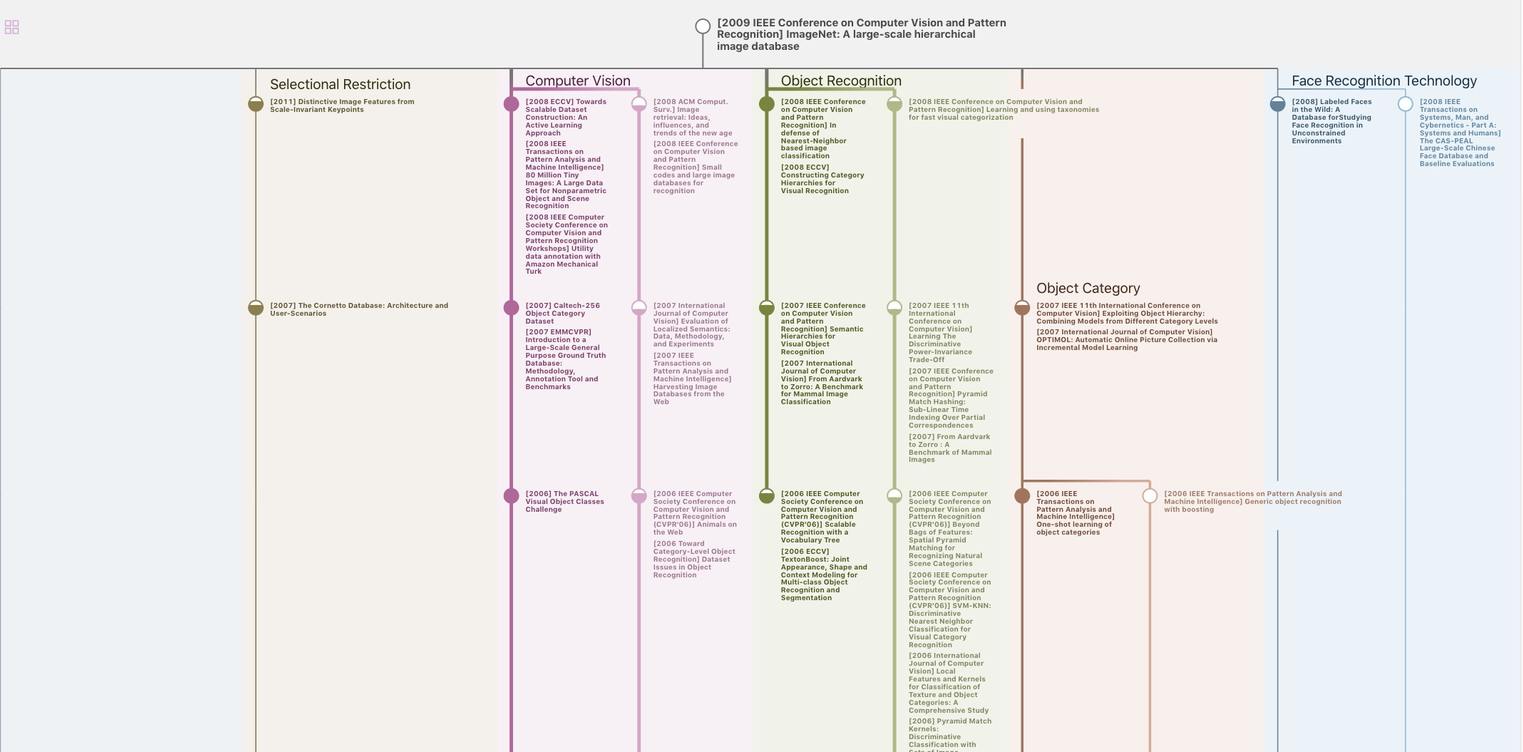
生成溯源树,研究论文发展脉络
Chat Paper
正在生成论文摘要