A Classical Machine Learning Model Scheduling in Industrial Wireless Sensor Networks
Journal of Hunan University Natural Sciences(2022)
摘要
Time synchronization is a primary issue in industrial wireless sensor networks (IWSNs). It helps to optimize the connection and preserve battery consumption, and thus increase the network lifetime. This study aims to identify the most effective factors that decrease the battery consumption and monitor the critical targets in wireless sensor networks (WSNs) through addressing the coverage and connectivity aware scheduling of sensor nodes (SNs). On the other hand, this paper aims to get a scheduling algorithm for industrial wireless sensor networks of SNs by using classical machine learning in the proposed model like support vector machine, decision tree, and RProp (resilient back-propagation) algorithms. In this paper, classical machine learning methods are applied for testing the extracted features and the affected degree for network configurations. An extensive simulation run showed high accuracy for machine learning measurements and extracted the most affected features that play a big role in the sensor node scheduling in industrial wireless sensor networks. For testing, we used the KNIME (KoNstanz Information MinEr ) model that gives a result with high accuracy. The SVM (Support Vector Mashine), Decision Tree, and RProp classifiers give an accuracy of 92.489%, 97.979%, and 98.335%, respectively.
更多查看译文
AI 理解论文
溯源树
样例
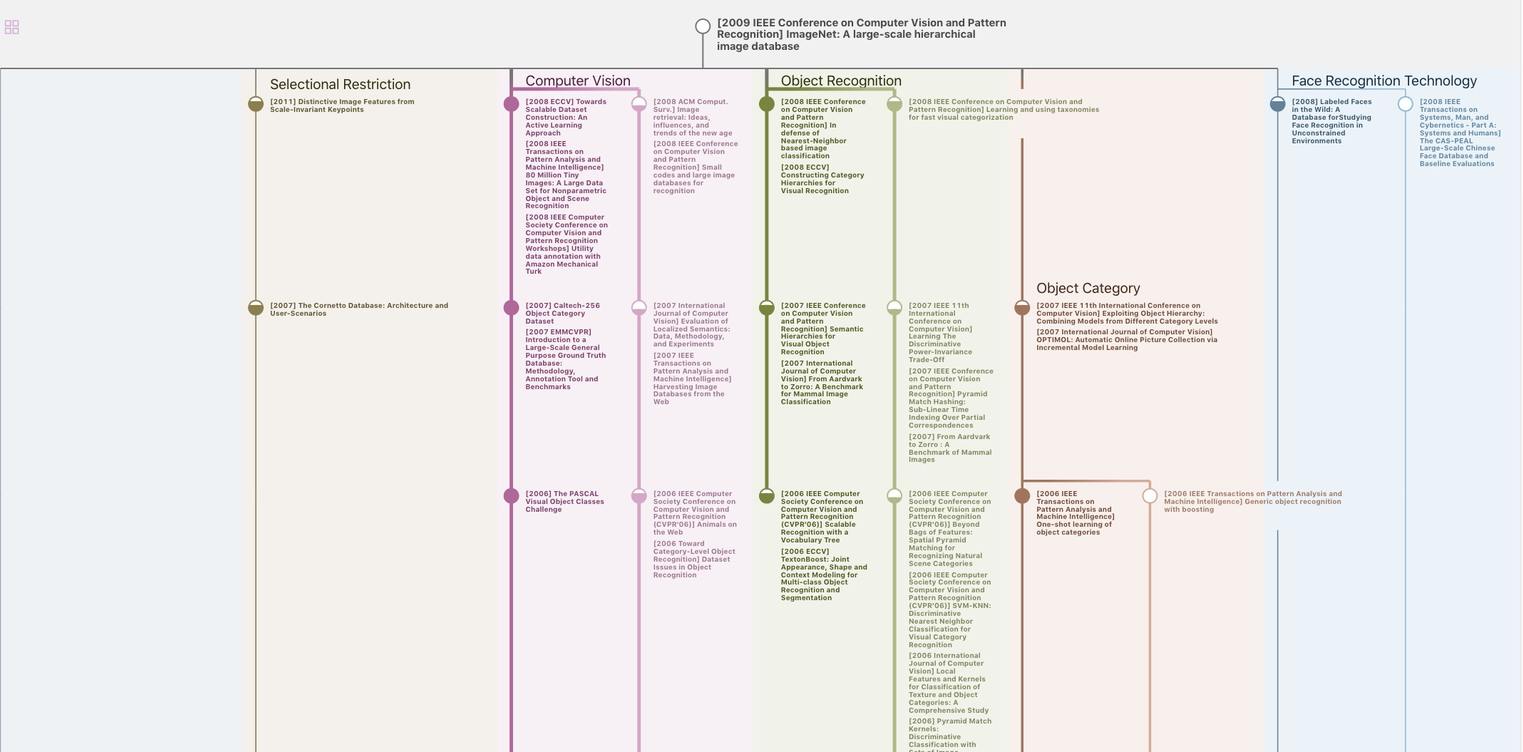
生成溯源树,研究论文发展脉络
Chat Paper
正在生成论文摘要