Enhancing Distributed In-Situ CNN Inference in the Internet of Things
IEEE Internet of Things Journal(2022)
摘要
Convolutional neural networks (CNNS) enable machines to view the world as humans and become increasing prevalent for Internet of Things (IoT) applications. Instead of streaming the raw data to the cloud and executing CNN inference remotely, it would be very attractive to use local IoT devices to process as it enables IoT applications with independent decision-making ability. Since a single IoT device can hardly match the requirements of the CNN inference, especially for time-sensitive and high-accuracy tasks, the distributed
in-situ
CNN inference becomes a potential solution. However, because of the inherently tightly coupled structure of existing CNN models, it is difficult to distribute the inference efficiently. In this article, we enhance the distributed
in-situ
CNN inference in the IoT. We fundamentally reduce the communication overhead of distributed CNN inference by designing new loosely coupled structure (LCS). Experimental results demonstrate that LCS achieves the leading performance compared with other popular structures. Next, based on the LCS, we customize the partitioning method to reduce the synchronization points and design the decentralized asynchronous method to optimize communication in each synchronization point. To evaluate the effectiveness, we build a prototype system. When the number of IoT devices increases from 1 to 4, our system accelerates by up to
$3.85\times $
and reduces the memory footprint in each device by 70% with achieving a competitive accuracy and significantly outperforming other approaches.
更多查看译文
关键词
Distributed inference,in-situ processing,intelligent Internet of Things (IoT)
AI 理解论文
溯源树
样例
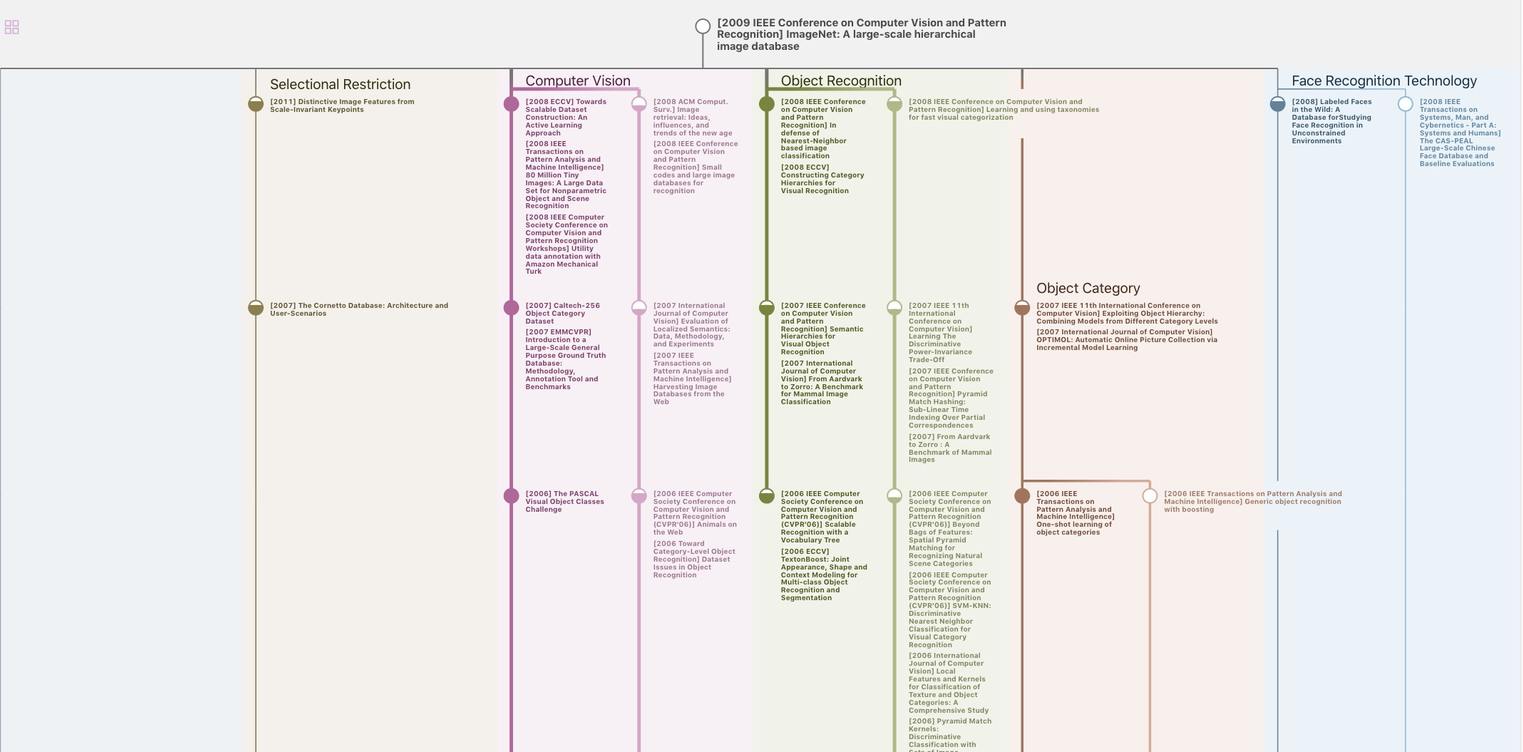
生成溯源树,研究论文发展脉络
Chat Paper
正在生成论文摘要