MO463: Machine Learning-Based Prediction of Mortality and Risk Factors in Patients With Chronic Kidney Disease Developed With Data From 10000 Patients Over 11 Years
Nephrology Dialysis Transplantation(2022)
摘要
Abstract BACKGROUND AND AIMS Around the globe, over 850 million patients suffer from chronic kidney disease (CKD). These have associated with high mortality rates, in particular when undergoing renal replacement therapies (RRT) such as dialysis, reaching up to 10% a year, and therefore, are considered of a fragile status. CKD is also associated with cardiovascular complications that can cause mutual aggravation. Available clinical guidelines identify certain risk factors and predictive models, but those have not been tested and validated successfully for renal patients and consequently, there is for the identification of predictive factors and the prediction of mortality. This is caused by the limitations of current methodologies and statistics: current models simplify complex relationships by assuming a linear relation between risk factors and certain events, and so, there is a need for a new approach. Over the last years, a rise in Artificial Intelligence and Machine Learning has been seen, presenting an alternative for the first time. This project aimed to study the performance of different ML algorithms for the prediction of mortality and the identification of risk factors for CKD patients. METHOD Design: Retrospective analysis of a historical cohort from the Register of Renal Patients of Catalonia (RMRC) and the Catalan Agency for Health Quality and Evaluation. Group of 10 473 patients with CKD stages from first to RRT. Follow-up of 11 years, from January 2010 to December 2020. Inclusion criteria: ˃18 years. Training of an Extreme Gradient Boosting model, and comparison with other algorithms for the prediction of mortality at different times, using different follow-up periods for each patient. Methodology: Variables: i) Age, gender, body mass index, time for death (9), ii) Diagnoses (ICD-9/10) (26); iii) Laboratory variables (37) iv) All pharmacological treatments (46). For all executions, data was balanced using the SMOTETomek technique. Analysis: RESULTS The patient sample presented a mean of 68.2 ± 12.9 years and 65.8% were female and 34.2% male. Different follow-up and time windows were tested and the best results were obtained when using a 2-year period follow-up and a 4-year mortality prediction. The Area Under the Curve values obtained for each model were: XBGClassifier (0.89), LGBM Classifier (0.90), CatBoost Classifier (0.91). The 10 variables with major relevance according to the XBGClassifier (54.65% of the total weight of the 71 variables) and in this order, are cardiopathy, advanced chronic kidney disease, vasculopathy, age, neoplasia, transplant, digestive pathology, estimated glomerular filtration rate, high blood pressure. The results presented in Figure and Table correspond to the mean obtained for the 5-folds of the Cross-Validation. CONCLUSION Machine Learning techniques suppose an alternative to classical statistical methods, with a high predictive capacity for mortality. The possibility of generating algorithms with real-world data can allow the individualization of the mortality risk as well as the predictive factors.
更多查看译文
AI 理解论文
溯源树
样例
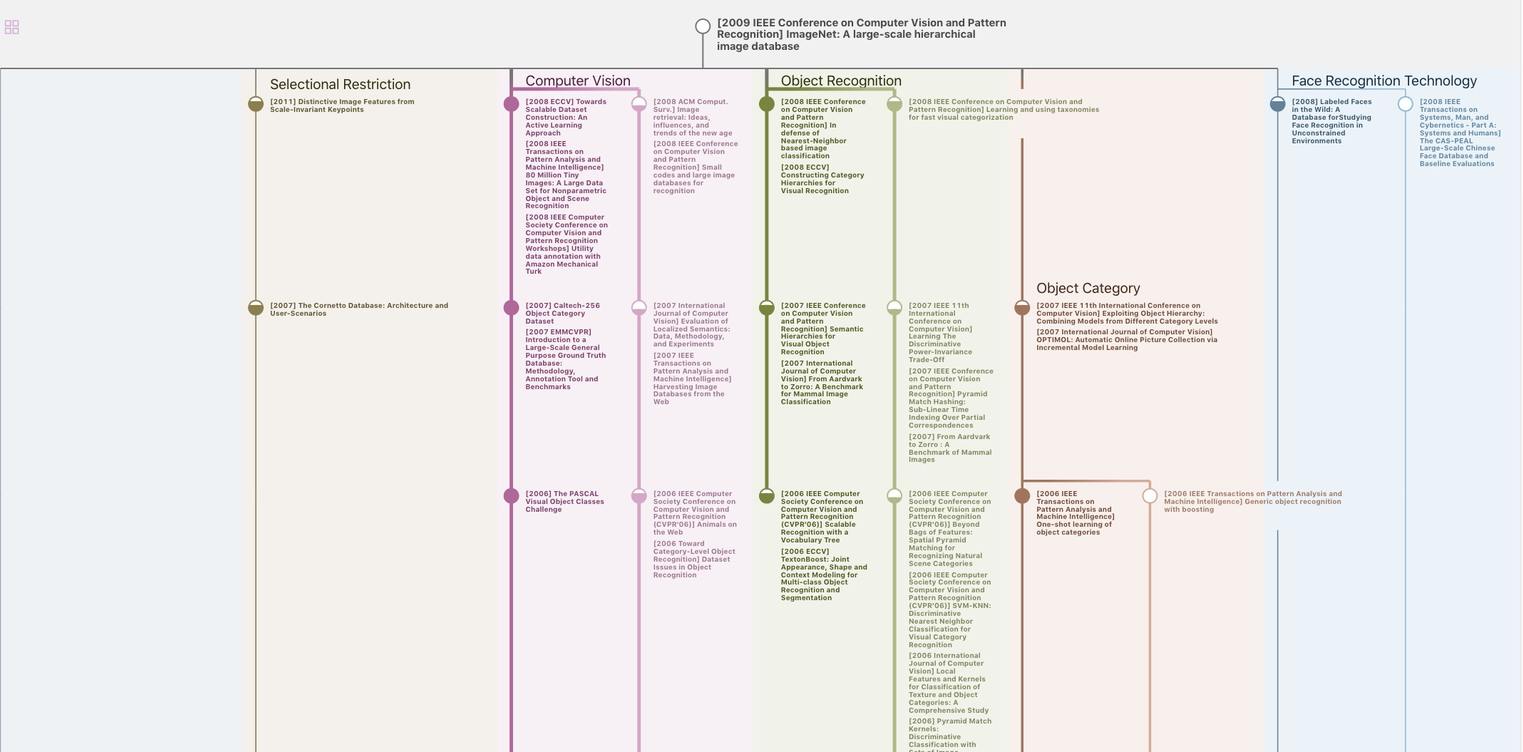
生成溯源树,研究论文发展脉络
Chat Paper
正在生成论文摘要