STRUCTURAL EQUATION MODELLING TO IMPROVE KIDNEY FUNCTION ASSESSMENT IN THE GENERAL POPULATION
Nephrology Dialysis Transplantation(2022)
摘要
Abstract BACKGROUND AND AIMS In order to identify behavioral, environmental, biological or genetic determinants of kidney function, population-based epidemiological studies rely on approximate estimates of the glomerular filtration rate (eGFR) based on measured markers such as serum creatinine, cystatin C, and a few other [1,2]. Since none of these markers is the exclusive reflection of the kidney function, results should always be interpreted with caution to disentangle determinants of kidney function from those that are specific to the marker metabolism. A structural equation modelling (SEM) approach could overcome these limitations allowing to estimate the unobservable kidney function based on a larger number of markers, improving the possibilities of conducting more specific inferential studies [3]. We implemented SEM to identify a latent kidney function trait in a general population sample and validated this derived biomarker against 10-year incidence of kidney disease using an independent dataset. METHOD In the population-based MICROS study (South Tyrol, Italy) [4], we measured serum creatinine (SCr), uric acid (UA), blood urea nitrogen (BUN), cystatin C (CysC), and serum albumin in 1317 study participants. We split the sample into a model-building (n = 647) and a validation set (n = 670). The model-building set was used for SEM analysis and estimation of factor loadings for each biomarker, using the R package ‘lavaan’. Participants in the validation set were submitted a 10-year follow-up interview on kidney disease within the Cooperative Health Research In South Tyrol (CHRIS) study [5]. We fitted logistic regression models to assess the predictivity of the estimated latent kidney function trait versus each individual marker, CKD-EPI eGFR estimates based on serum creatinine and cystatin C (CKD-EPI 2009; CKD-EPI 2021; CKD-EPI Cys; and CKD-EPI CreCys), as well as MDRD-4 and MDRD-6. We estimated odds ratios (ORs) for 10-year kidney disease incidence per standard deviation (SD) change in each marker. RESULTS In the model-building set (mean age 49 years, SD = 19; 58% females; mean eGFRCKD-EPI 2021 = 85.9, SD = 20.9), we identified factor loadings for SCr, UA, BUN, and CysC (Figure 1) achieving acceptable goodness of fit (Chi-square = 14.7; CFI = 0.98; TLI = 0.93; and RMSEA = 0.099). The validation set (baseline mean age = 40 years, SD = 13; 55% females; mean eGFRCKD-EPI 2021 = 100.8, SD = 16.1) had a 10-year kidney disease incidence of 7.8%. For the latent kidney function trait, we observed an OR of 2.1 (95% CI: 1.4–3.2) for incidence per each SD change. This was lower than the ORs (95% CI) associated with the eGFRMDRD4 (2.3, 1.4–4.1) and eGFRMDRD6 (2.5, 1.5–4.2), but larger than the ORs (95% CI) observed for all other markers (SCr: 1.8, 1.3–2.6; UA: 1.2, 0.9–1.8; BUN: 1.3, 0.9–1.8; and CysC: 1.3, 1.0–1.7) or eGFR estimates (eGFRCKD-EPI2009: 1.8, 1.2–2.7; eGFRCKD-EPI2021: 1.7, 1.2–2.5; eGFRCKD-EPI Cys: 1.4, 1.0–1.9; and eGFRCKD-EPI CreCys: 1.8, 1.2–2.6). CONCLUSION A latent kidney function trait derived from SEM performed similarly and often better than conventional eGFR estimates in a 10-year kidney disease incidence prediction. The method warrants validation in independent general population samples with large sample size.
更多查看译文
AI 理解论文
溯源树
样例
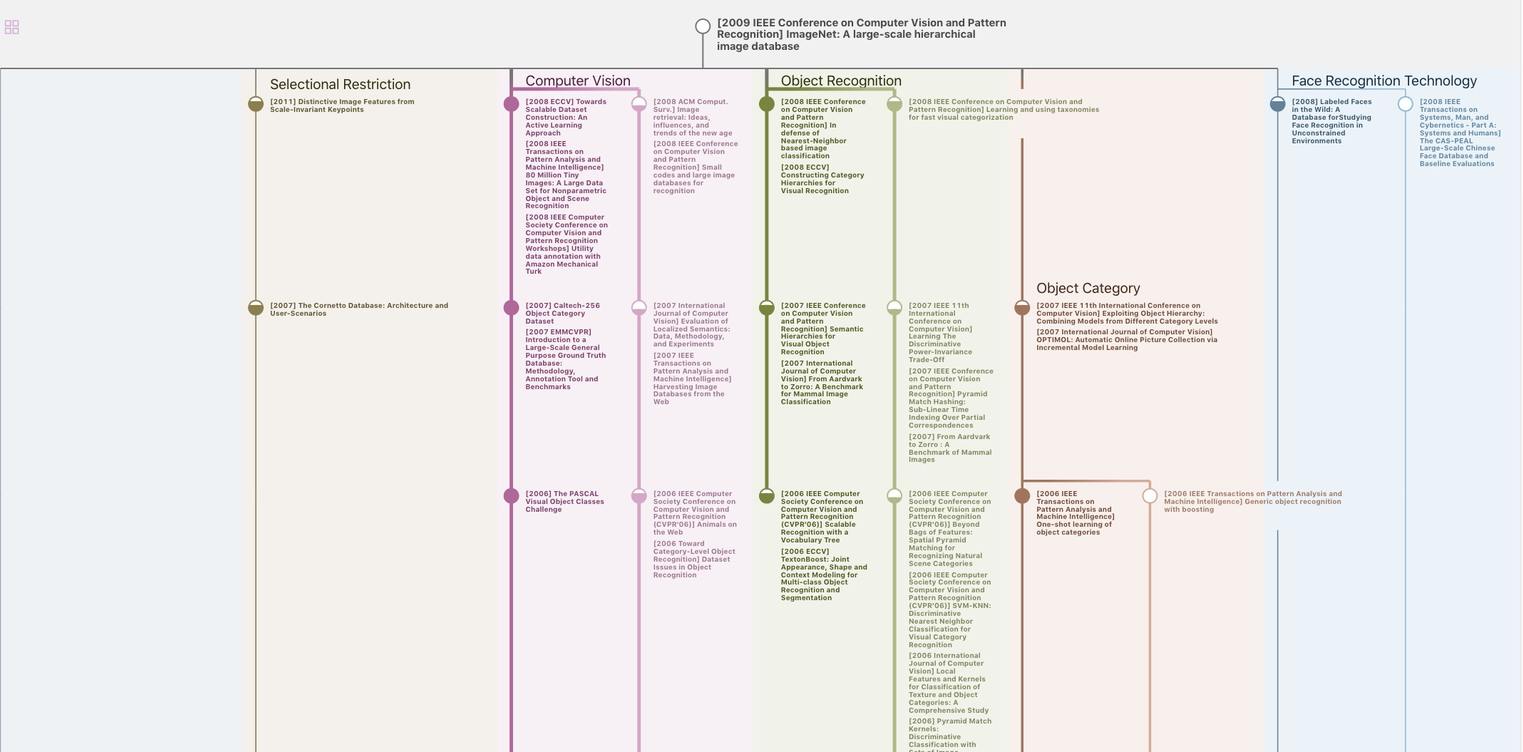
生成溯源树,研究论文发展脉络
Chat Paper
正在生成论文摘要