Mean-Field Learning for Edge Computing in Mobile Blockchain Networks
IEEE TRANSACTIONS ON MOBILE COMPUTING(2023)
Abstract
Blockchain has been leveraged to secure transactions for the m-commerce. However, the intensive computation in the mining process restricts the participation of mobile devices. Currently, some studies have deployed edge computing services to support the mining process, where edge servers managed by one Service Provider (SP) are considered. This paper investigates a more practical scenario with multiple SPs, where servers managed by different SPs have distinct capacities and prices, making miners' offloading decisions rather complicated. To tackle the above challenges, we consider task offloading, block propagation and miner mobility comprehensively to maximize utilities of miners. Specifically, we first formulate a Markov game, and then design a learning-based offloading algorithm for off-chain computation, where a novel learning model is constructed by integrating Deep Reinforcement Learning (DRL) and Mean Field Theory (MFT) to guarantee a Nash equilibrium. Different from existing studies, each miner merely needs to respond to the average effect from others in our system, insteading of knowing policies of others. Finally, both theoritical and performance results show that our designed algorithm has superiority on average miner utilities and algorithm convergence time compared with other representative algorithms.
MoreTranslated text
Key words
Mobile blockchain,deep reinforcement learning,mean field theory,multiple service providers
AI Read Science
Must-Reading Tree
Example
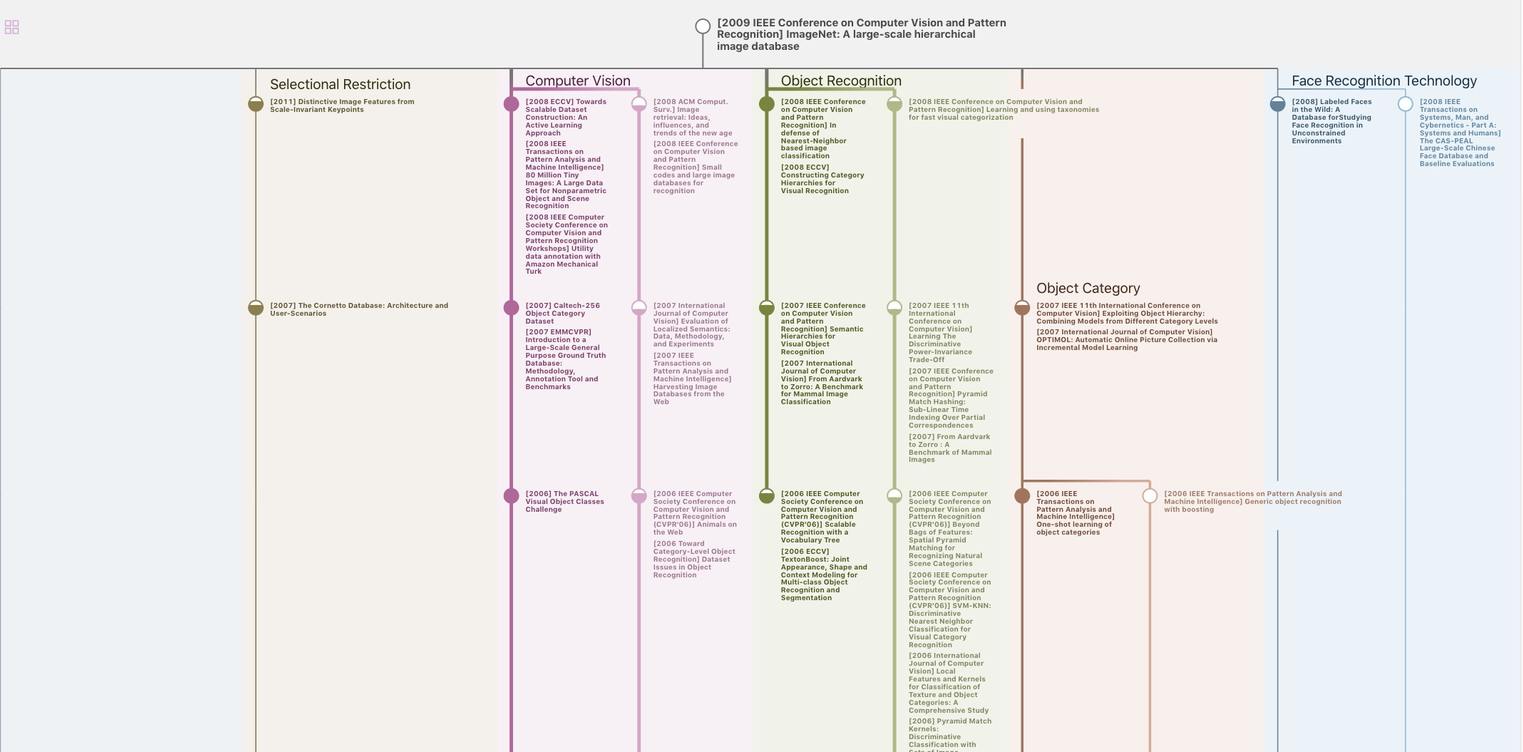
Generate MRT to find the research sequence of this paper
Chat Paper
Summary is being generated by the instructions you defined