LaBaNI: Layer-based Noise Injection Attack on Convolutional Neural Networks
Proceedings of the Great Lakes Symposium on VLSI 2022(2022)
摘要
Hardware accelerator-based CNN inference improves the performance and latency but increases the time-to-market. As a result, CNN deployment on hardware is often outsourced to untrusted third parties (3Ps) with security risks, like hardware Trojans (HTs). Therefore, during the outsourcing, designers conceal the information about initial and final CNN layers from 3Ps. However, this paper shows that this solution is ineffective by proposing a hardware-intrinsic attack (HIA), Layer-based Noise Injection (LaBaNI), which successfully performs misclassification without knowing the initial and final layers. LaBaNi uses the statistical properties of feature maps of the CNN to design the trigger with a very low triggering probability and a payload for misclassification. To show the effectiveness of LaBaNI, we demonstrated it on LeNet and LeNet-3D CNN models deployed on Xilinx's PYNQ board. In the experimental results, the attack is successful, non-periodic, and random, hence difficult to detect. Results show that LaBaNI utilizes up to 4% extra LUTs, 5% extra DSPs, and 2% extra FFs, respectively.
更多查看译文
关键词
noise injection attack,convolutional neural networks,neural networks,layer-based
AI 理解论文
溯源树
样例
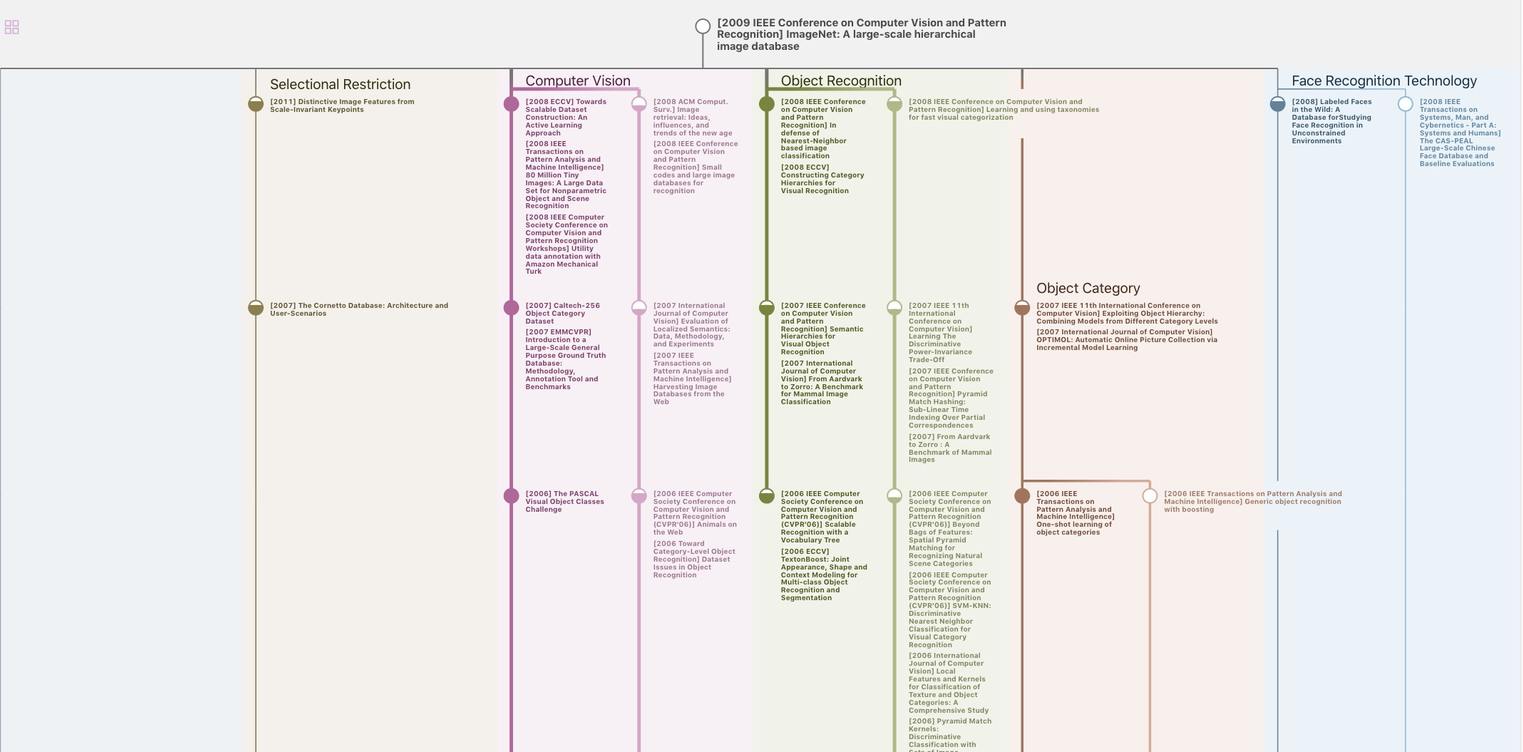
生成溯源树,研究论文发展脉络
Chat Paper
正在生成论文摘要