EntailSum: An Entailment Based Approach to Aspect-Based Text Summarization with Automated Aspect Adaptation
International Journal of Pattern Recognition and Artificial Intelligence(2022)
摘要
Aspect-based summarization differs from generic text-summarization in which the generated summary must be conditioned on a given topic. A fundamental challenge to the aspect-based summarization approach is the lack of labeled data for training models, which limits the usage of supervised methods. One approach to address this issue is to introduce human intervention to generate unique datasets per aspect. However, there is a large number of possible aspects to summarize which makes this option impossible to scale. This limits the use of typical modeling techniques, and requires methods which excel in few-shot, or ideally zero-shot regimes. Hence, in this research, we propose a modular, two-step approach that does not need any aspect-based supervision. This research combines recent advances in zero-shot text classification and generic summarization in a novel way. The backbone of the proposed approach is a transformer network trained for the task of textual entailment, which is used to reduce a document to the set of on topic sentences. In the experiments, our model achieves a new state of the art compared to other unsupervised models on the MA-News dataset (ROUGE-1 35.70 and ROUGE-2 15.52), and even outperforms fine-tuned models without any supervision of its own.
更多查看译文
关键词
Aspect-based summarization, entailment, zero-shot, natural language processing
AI 理解论文
溯源树
样例
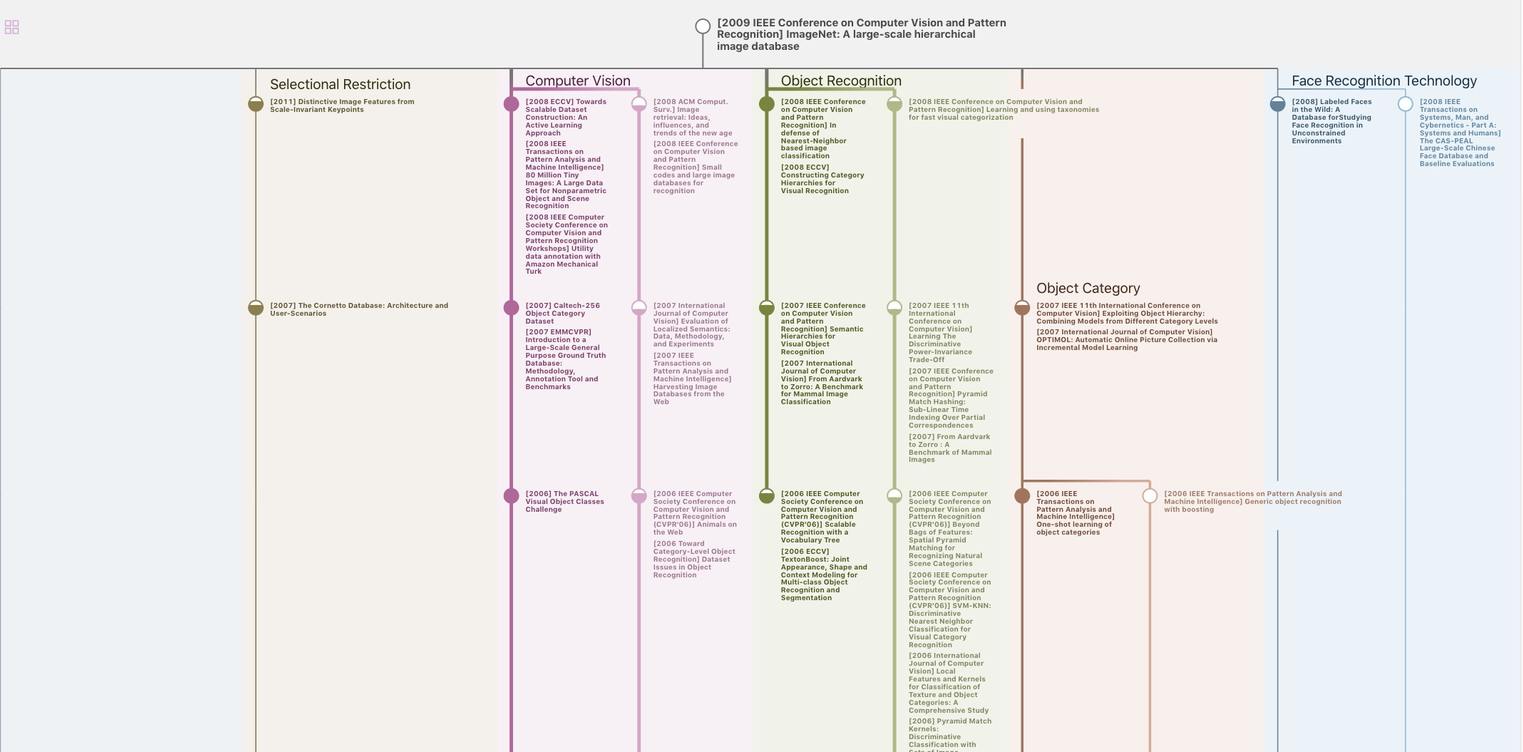
生成溯源树,研究论文发展脉络
Chat Paper
正在生成论文摘要