Multitask Particle Swarm Optimization With Dynamic On-Demand Allocation
IEEE Transactions on Evolutionary Computation(2023)
摘要
Multitask optimization aims to solve multiple optimization problems in parallel utilizing a single population. However, if the computing resources are limited, allocating the same computing resources to different tasks will cause resource waste and make complex tasks difficult to converge to the optimal solution. To address this issue, a multitask particle swarm optimization with a dynamic on-demand allocation strategy (MTPSO-DA) is proposed to dynamically allocate computing resources. First, a task complexity index, based on convergence rate and contribution rate, is designed to evaluate the difficulty of solving different tasks. Then, the complexity of different tasks can be evaluated in real time. Second, the skill factor of the particle is extended to a time-varying matrix according to the task complexity index. Then, the recently captured feedback is stored to determine the computational resource demands of the task. Third, an on-demand allocation strategy, based on the time-varying matrix, is developed to obtain the skill factor probability vector utilizing the attenuation accumulation method. Then, computing resources can be allocated dynamically among different tasks. Finally, some comparative experiments are conducted based on the benchmark problem to evaluate the superiority of the MTPSO-DA algorithm. The results indicate that the proposed MTPSO-DA algorithm can achieve dynamic resource allocation.
更多查看译文
关键词
Complexity,multitask optimization (MTO),resource allocation
AI 理解论文
溯源树
样例
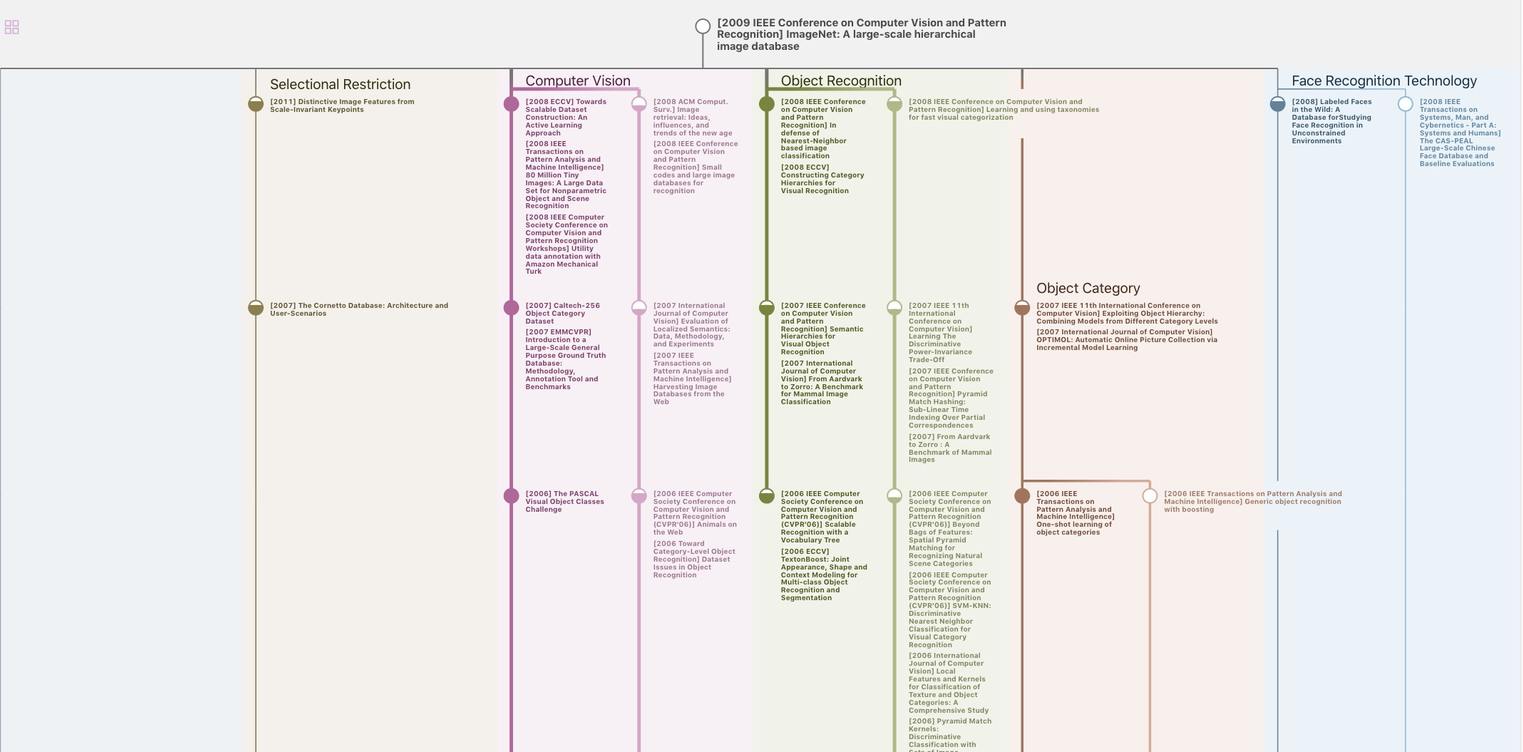
生成溯源树,研究论文发展脉络
Chat Paper
正在生成论文摘要