Revisiting ensemble adversarial attack
Signal Processing: Image Communication(2022)
摘要
Deep neural networks have shown vulnerability to adversarial attacks. Adversarial examples generated with an ensemble of source models can effectively attack unseen target models, posing a security threat to practical applications. In this paper, we investigate the manner of ensemble adversarial attacks from the viewpoint of network gradients with respect to inputs. We observe that most ensemble adversarial attacks simply average gradients of the source models, ignoring their different contributions in the ensemble. To remedy this problem, we propose two novel ensemble strategies, the Magnitude-Agnostic Bagging Ensemble (MABE) strategy and Gradient-Grouped Bagging And Stacking Ensemble (G2BASE) strategy. The former builds on a bagging ensemble and leverages a gradient normalization module to rebalance the ensemble weights. The latter divides diverse models into different groups according to the gradient magnitudes and combines an intragroup bagging ensemble with an intergroup stacking ensemble. Experimental results show that the proposed methods enhance the success rate in white-box attacks and further boost the transferability in black-box attacks.
更多查看译文
关键词
Adversarial attack,Ensemble strategies,Gradient-based methods,Deep neural networks,Image classification
AI 理解论文
溯源树
样例
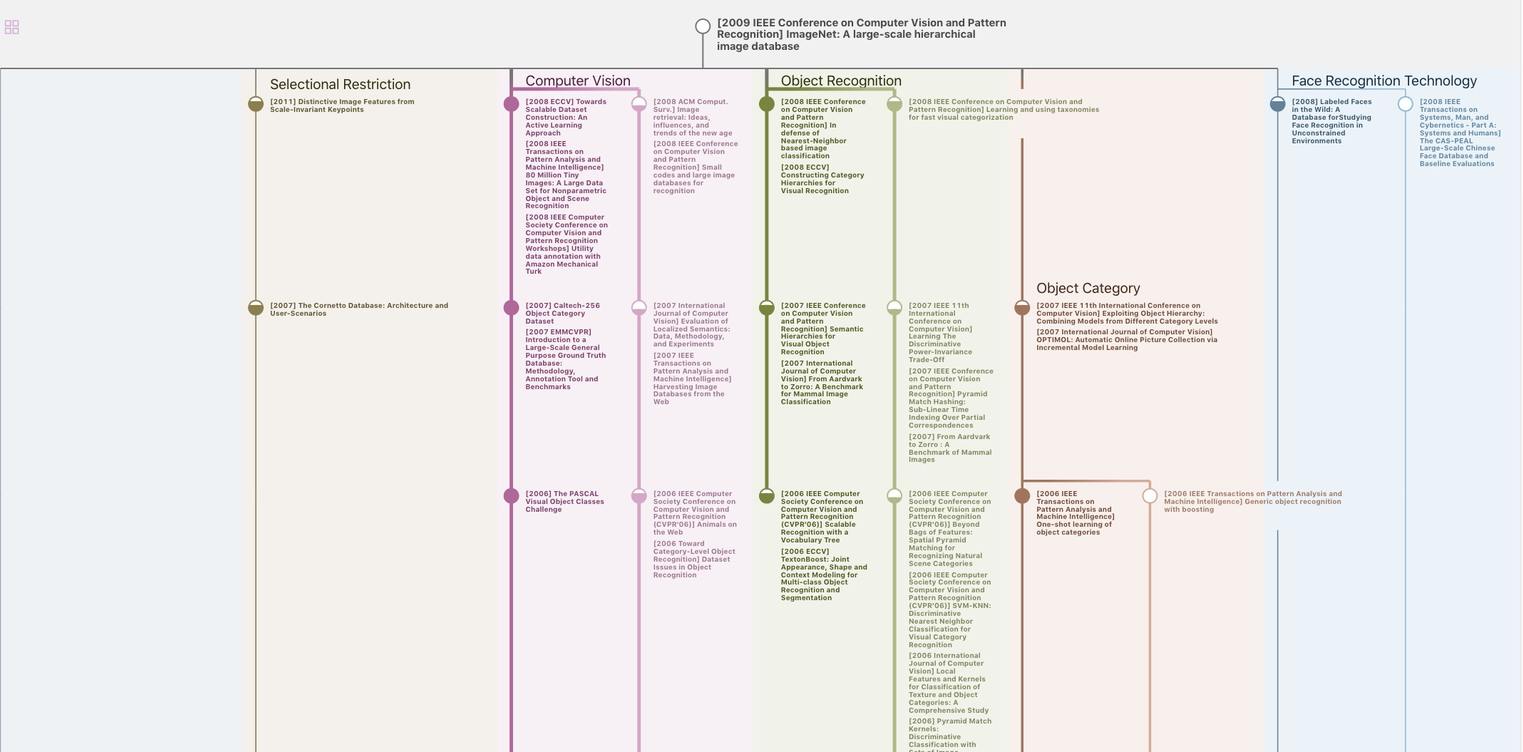
生成溯源树,研究论文发展脉络
Chat Paper
正在生成论文摘要