P &GGD: A Joint-Way Model Optimization Strategy Based on Filter Pruning and Filter Grafting For Tea Leaves Classification
NEURAL PROCESSING LETTERS(2022)
摘要
In the automatic processing of tea, the category of fresh tea leaves determines the processing parameters for the automatic control systems. The automatic classification of fresh tea leaves is a necessity. Previous works used deep and wide architectures of CNNs to classify the tea leaves, which have a limitation on the model deployment. Despite the filter pruning achieve superior performance about network compression and acceleration, the pruning-based approaches have to trade accuracy for low computational cost and model size. we propose a novel joint-way model optimization strategy, named Filter Pruning and Grafting via Geometric Distance(P GGD), to solve the above problems. Specifically, we utilize the filter pruning method to compress models and uses the filter grafting method to boost accuracy. To graft pruned models effectively, we develop the grafting criteria based on the geometric distance to measure the information of layers. Extensive experiments validate our approach on the fresh-tea-leaves dataset and two image classification benchmarks. For example, with the fresh-tea-leaves dataset, we achieve 96.296 % top-1 accuracy, with more than 45 % FLOPs-reduction, even outperforms the non-compress MobileNetV2 by 2.395 % accuracy. Our proposed method can provide more accurate, more compact, and faster models. Thus, it can promote the deployment of the model at the edge of the automatic production line.
更多查看译文
关键词
Image classification,Filter pruning,Filter grafting,Lightweight convolution neural networks
AI 理解论文
溯源树
样例
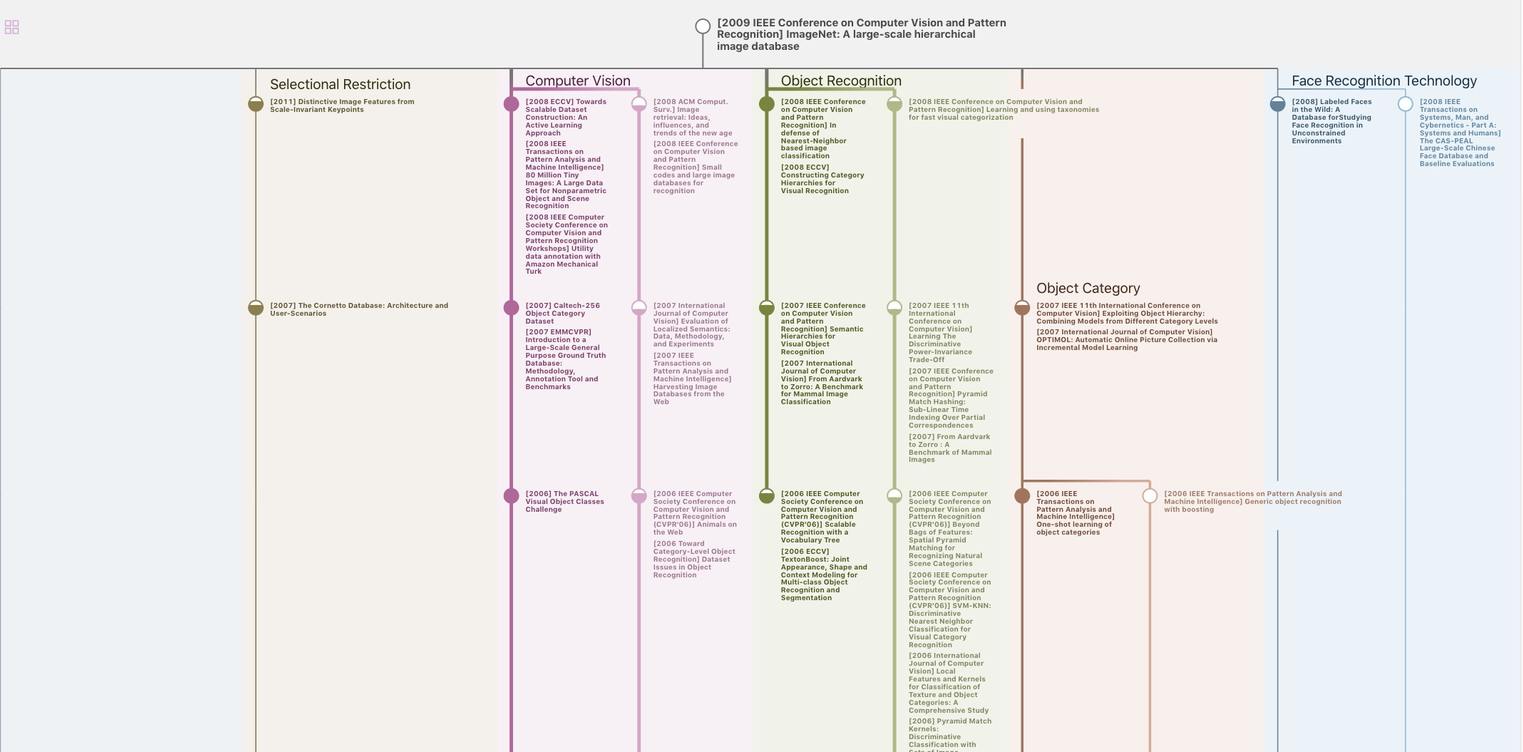
生成溯源树,研究论文发展脉络
Chat Paper
正在生成论文摘要