An ultra-sensitive assay using cell-free DNA fragmentomics for multi-cancer early detection.
Journal of Clinical Oncology(2022)
摘要
3037 Background: Although most cancer can benefit from early detection by more effective treatments and better prognosis, current screening programs are only limited to tumor-specific tests for a subset of common cancers. To benefit a broader population and outweigh the risks of single-cancer tests, an effective and affordable multi-cancer test should be developed for sensitive early detection of multiple cancers and accurate prediction of cancer tissue of origin simultaneously. Methods: In this study, we enrolled 971 cancer patients of the most prevalent and lethal cancer types, including primary liver cancer (PLC, N= 381), colorectal adenocarcinoma (CRC, N= 298), and lung adenocarcinoma (LUAD, N= 292), as well as 243 healthy controls. The participants were randomly divided into a training cohort and a test cohort in a 1:1 ratio. Five fragmentomic features representing cfDNA fragmentation size, motif sequence, and copy number variation were extracted from processed whole-genome sequencing (WGS) data of the participants to build the base models. Each base model implemented five machine learning algorithms for model training, and the optimal base models were used to create the final multi-dimensional model through ensemble stacked machine learning. The integrated multi-cancer model is composed of the first-level binary cancer detection model and the second-level multi-classification cancer origin model. The training cohort was used to train the models with 10-fold cross-validation. The test cohort remained untouched during model construction and was solely used for performance evaluation. Results: Our cancer samples are highlighted by mostly early-stage diseases (early-stage PLC: 88.5%; CRC: 100.0%; LUAD: 100.0%). The cancer detection model reached an area under the curve (AUC) of 0.983 for differentiating cancer patients from healthy individuals in the test cohort. At 95.0% specificity, the sensitivity of detecting all cancer is 95.5%, and 100.0%, 94.6%, and 90.4% for PLC, CRC, and LUAD, respectively. Its sensitivity is consistently high for early-stage, small-size tumors. The cancer origin model demonstrated an overall 93.1% accuracy for predicting tissue of origin in the test cohort (97.4%, 94.3%, and 85.6% for PLC, CRC, and LUAD, respectively). Furthermore, the model's cancer detection and origin classification performance remained robust when reducing sequencing depth to 1× (cancer detection: ≥ 91.5% sensitivity at 95.0% specificity; cancer origin: ≥ 91.6% accuracy). Conclusions: We utilized multiple plasma cfDNA fragmentomic features to build an ensemble stacked machine learning model. The assay reached ultrasensitivity and accuracy for multi-cancer early detection, shedding light on leveraging cfDNA fragmentomics for early screening in clinical practice.
更多查看译文
AI 理解论文
溯源树
样例
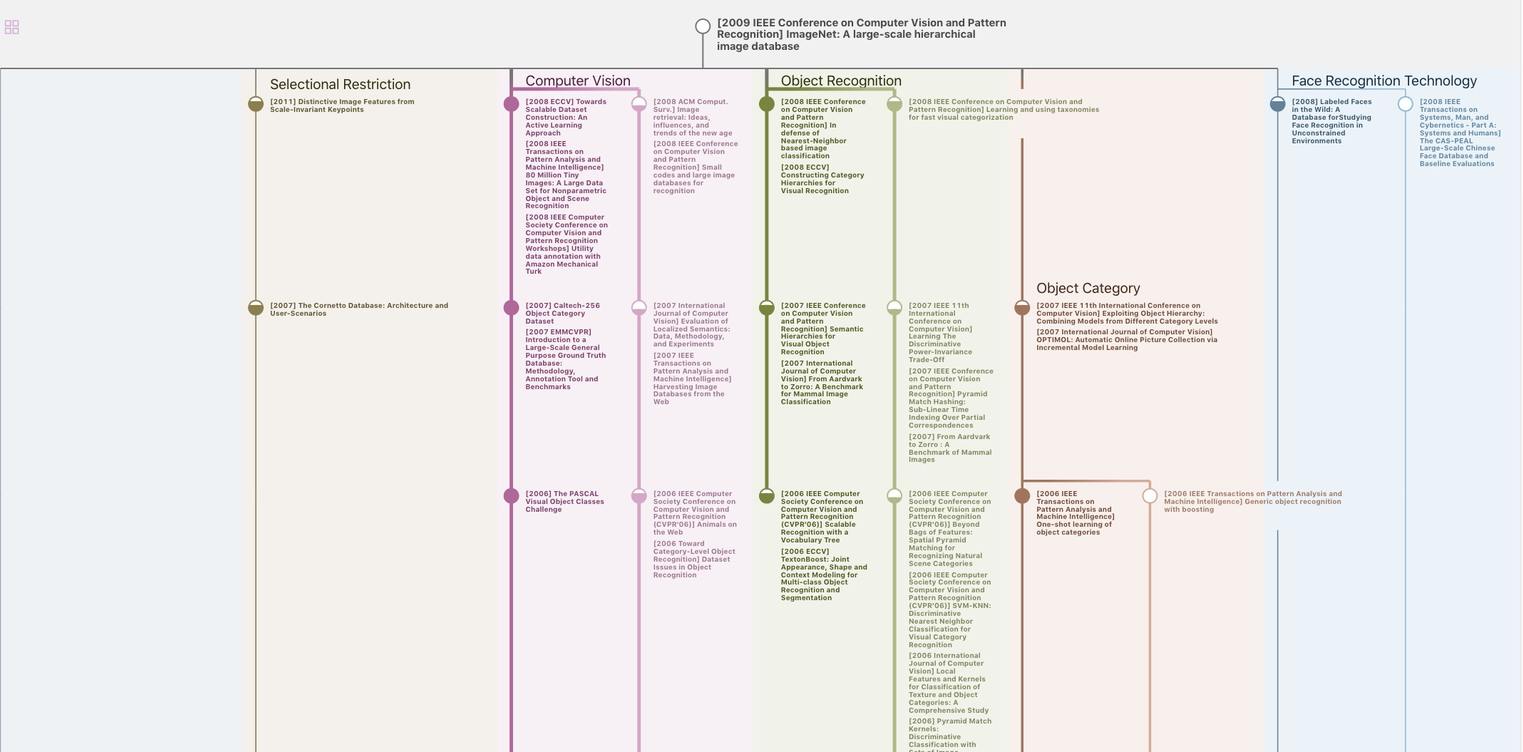
生成溯源树,研究论文发展脉络
Chat Paper
正在生成论文摘要