Explicit Message-Passing Heterogeneous Graph Neural Network
IEEE Transactions on Knowledge and Data Engineering(2023)
摘要
Graph neural network (GNN) has shown its prominent performance in representation learning of graphs but it has not been fully considered for heterogeneous graphs which contain more complex structures and rich semantics. The rich semantic information of heterogeneous graph can be usually revealed by meta-paths. Therefore, most of the existing GNN models designed for heterogeneous graphs utilize the meta-path based neighborhood sampler to divide a heterogeneous graph into multiple homogeneous subgraphs according to various meta-paths so that the homogeneous GNN can be applied to investigate heterogeneous graphs. Nevertheless, the way of embedding semantic information of meta-paths into multiple homogeneous graphs is implicit and ineffective, which cannot accurately capture the semantics of heterogeneous graphs. In this paper, we propose a novel semi-supervised GNN model named Explicit Message-Passing Heterogeneous Graph Neural Network (EMP), which executes the process of explicit message-passing along the meta-paths. Besides, we also propose a split method for meta-paths and consider mutual effect between various meta-paths in advance in the proposed model, so that the semantic information of the whole set of meta-paths can be captured accurately. Extensive experiments conducted on three real-world datasets demonstrate the superiority of the proposed model.
更多查看译文
关键词
Graph neural network,node representation learning,heterogeneous graphs,meta-path
AI 理解论文
溯源树
样例
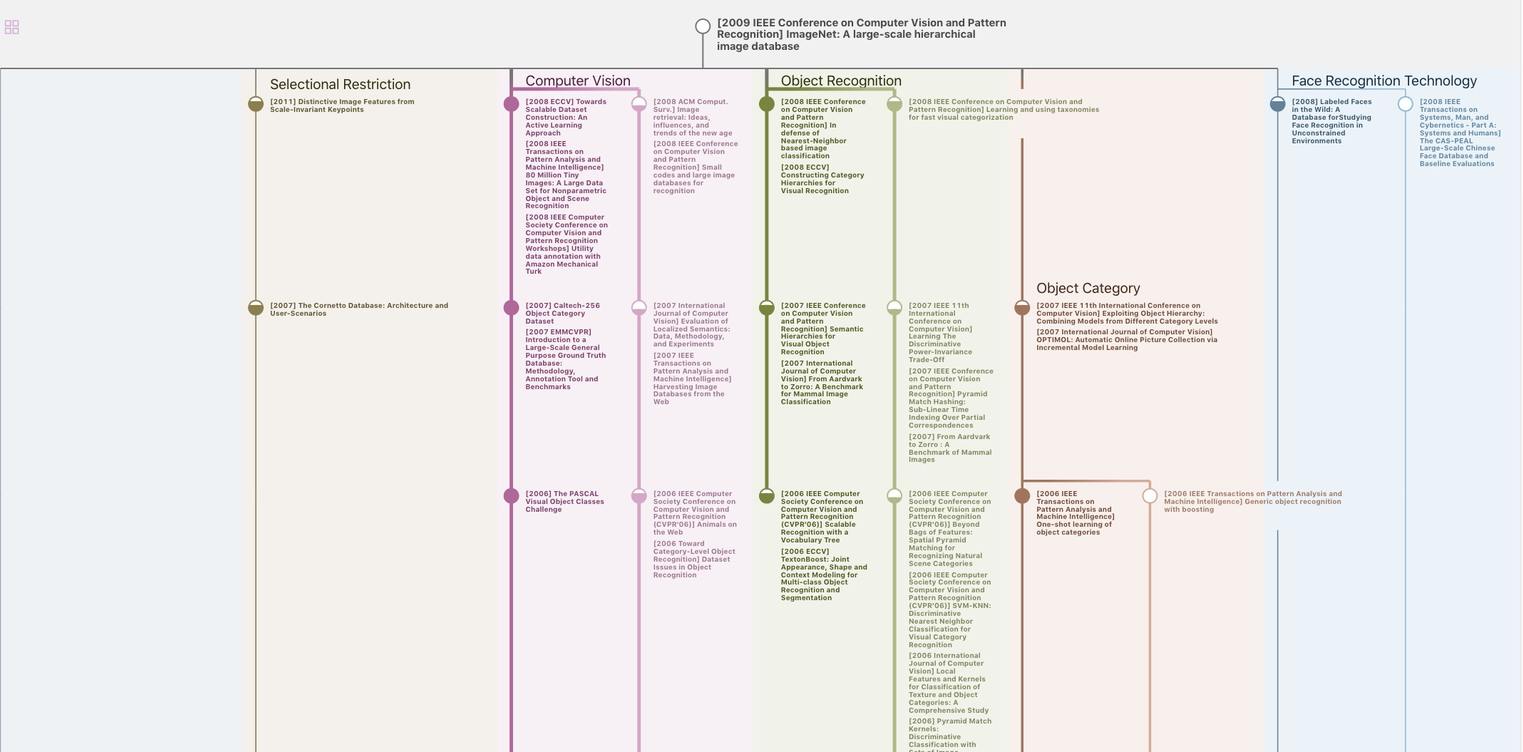
生成溯源树,研究论文发展脉络
Chat Paper
正在生成论文摘要