Automatic identification of oncology patients with unsupervised phenotyping and AutoML.
Journal of Clinical Oncology(2022)
摘要
e13547 Background: Recent studies suggest ICD codes often cannot characterise patients with specific diseases like oncology accurately in real-world clinical practice due to a high probability of miscoding, while manual inspection of millions of patients is not feasible as it is highly costly and slow. Therefore, we present an AI driven workflow, which leverages structured discrete values and unstructured textual data from Electronic Health Records (EHRs), to automatically identify cancer patients especially those who might be miscoded or undiagnosed. Methods: The proposed AI driven workflow is trained on 29637 and tested on 29339 patients from MIMIC-III database. First, the workflow builds patient profiles by leveraging the state-of-the-art unsupervised Natural Language Processing (NLP) method to extract phenotypic features from textual discharge summaries. Second, the workflow classifies if a patient has the risk of ovarian cancer (ICD-9: 183.0) or lung cancer (ICD-9: all subcodes of 162) based on their profiles using an Automated Machine Learning (AutoML) framework. Third, we improve the workflow through 100 gold-labelled patients out of the train set who are labelled as positive and negative ovarian (lung) cancer cases by three clinicians with consensus and we subsequently measure its performance against another 100 gold-labelled patients out of the test set and the entire test set with 29339 patients. Results: For ovarian cancer, on the 100 gold-labelled patients (52 ovarian cancer cases) out of the test set, the workflow achieves 0.877 AUCROC with precision 84.7% and sensitivity 96.2% whereas ICD codes have precision 94.6% and sensitivity 74.1%. On the entire test set with 29339 patients, the workflow identifies 111 ovarian cancer patients while ICD codes find only 38 patients which lead to 192% lifting. Similarly, for lung cancer, on the 100 gold-labelled patients (66 lung cancer cases) out of the test set, the workflow achieves 0.814 AUCROC with precision 88.7% and sensitivity 83.3% while ICD codes have precision 96.0% and sensitivity 72.7%. On the entire test set with 29399 patients, the workflow identifies 926 lung cancer patients while ICD codes find 585 patients which lead to 58% lifting. Conclusions: This study shows the AI driven workflow on textual clinical notes can help find more cancer patients with high accuracy especially those who are miscoded and undiagnosed by ICD codes. Since ICD codes are primarily used for billing purposes, this workflow also shows the opportunity to reconcile patient characteristics with ICD codes through the application of such novel AI at scale. Moreover, the results of this study have shown this approach can be scaled across other disease areas within oncology and beyond to help find new intelligence to characterise hard to diagnose conditions and improve patient outcomes through a significant improvement in speed and accuracy of screening and early diagnosis of patients.
更多查看译文
关键词
unsupervised phenotyping,oncology patients,automatic identification,automl
AI 理解论文
溯源树
样例
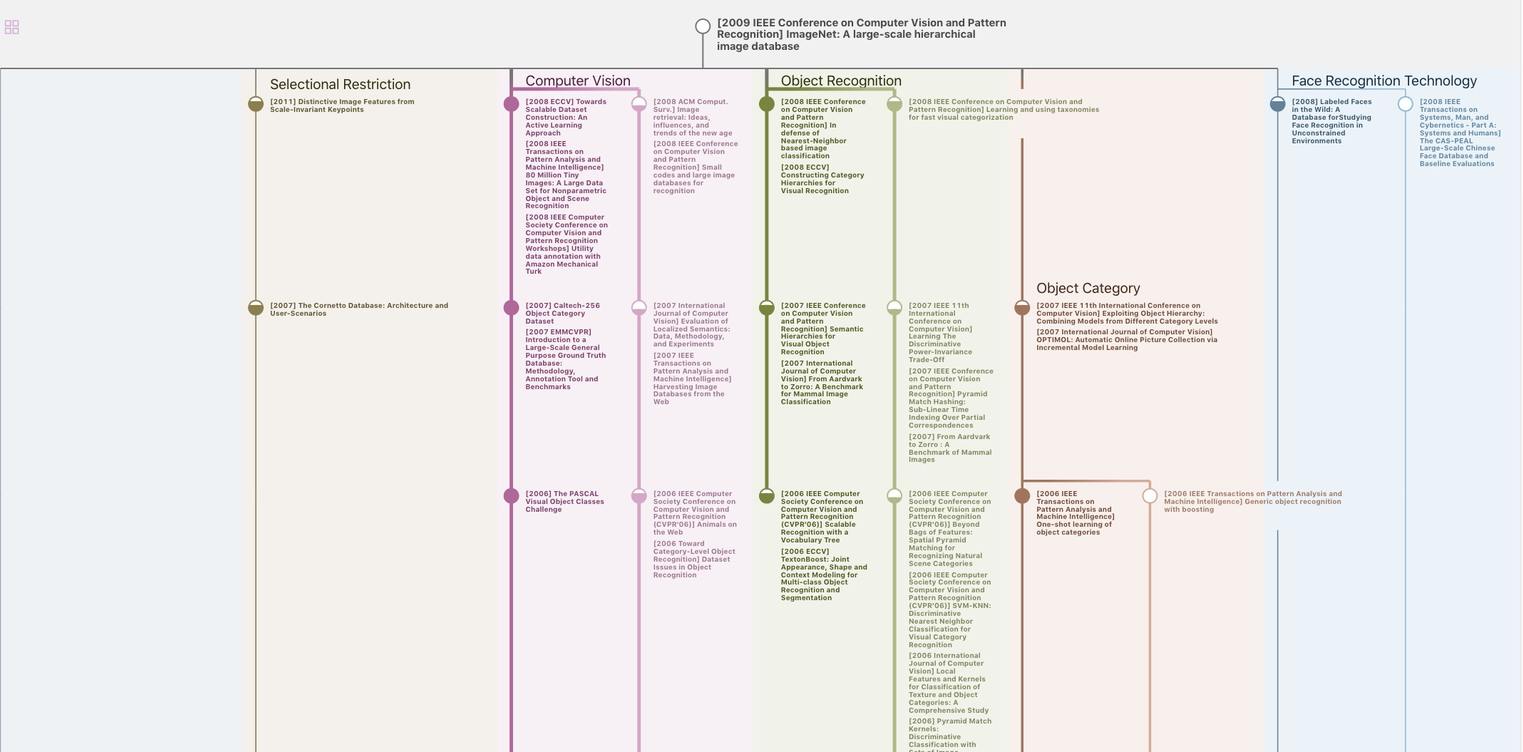
生成溯源树,研究论文发展脉络
Chat Paper
正在生成论文摘要