Quality Evaluation of Triples in Knowledge Graph by Incorporating Internal With External Consistency
IEEE TRANSACTIONS ON NEURAL NETWORKS AND LEARNING SYSTEMS(2024)
摘要
The evaluation of knowledge quality (KQ) in multisource knowledge graphs (KGs) is an essential step for many applications, such as fragmented knowledge fusion and knowledge base construction. Many existing quality evaluation methods for multisource knowledge are based on validation from high-quality knowledge bases or statistical analysis of knowledge related to a specific fact from multiple sources, named external consistency (EC)-based methods. However, high-quality KGs are difficult to obtain, and there might exist incorrect knowledge in multisource KGs interfering with KQ evaluation. To address the issue, this article refers to the internal structure of a KG to evaluate the degree to which the contained triples conform to the overall semantic pattern of the KG, such as KG embedding and logic inference-based approaches, defined as internal consistency (IC) evaluation. The IC is integrated with the EC to identify possible incorrect triples and reduce their influences on the KQ evaluation, thus alleviating the interference of incorrect knowledge. The proposed method is verified with multiple datasets, and the results demonstrate that the proposed method could significantly reduce wrong evaluations caused by incorrect knowledge and effectively improve the quality evaluation of triples.
更多查看译文
关键词
Integrated circuits,Measurement,Knowledge engineering,Iterative methods,Interference,Artificial neural networks,Statistical analysis,Consistency,knowledge graph (KG),knowledge quality (KQ),quality control
AI 理解论文
溯源树
样例
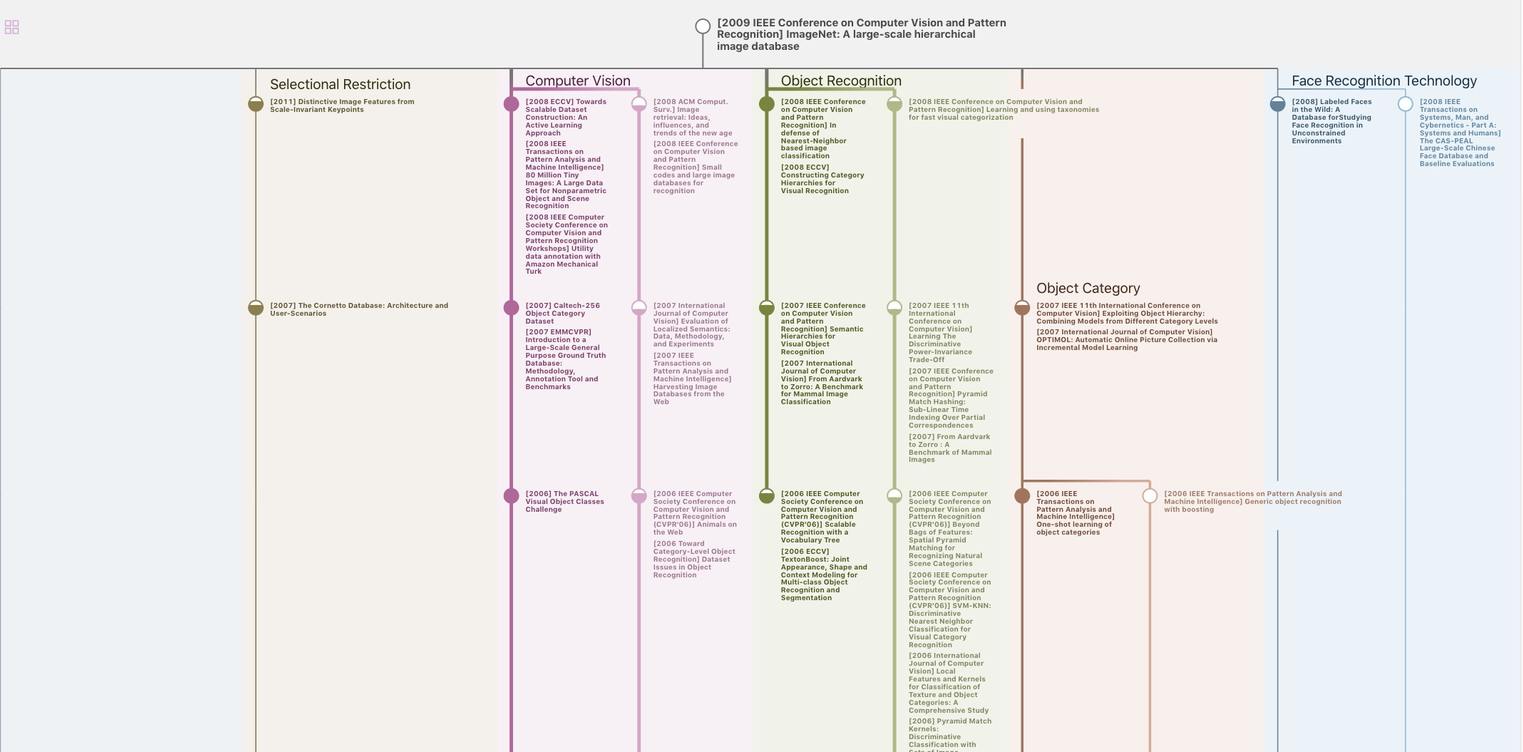
生成溯源树,研究论文发展脉络
Chat Paper
正在生成论文摘要