Anticipatory transport system with hybrid linear and nonlinear forecasting using streaming wafer process data
Applied Soft Computing(2022)
摘要
In semiconductor plants, autonomous vehicle systems (AVSs) are designed to transfer wafer lots using several hundreds of vehicles. To minimize the idleness of production machines, vehicles must be quickly assigned and sent to the required location in advance. Currently, owing to uncertainties that exit in production processing, simple deterministic heuristic approaches are the ones that are predominantly used when making decisions regarding lot discharging and recharging of production machines. However, to obtain better solutions that reflect the near-future states of production machines and AVSs, more sophisticated approaches are required. To address this, we propose a hybrid predictive algorithm using which a delivery vehicle can be sent in advance to minimize the production machine idle time. To predict the remaining processing time, we combine a latent variable regression and an artificial neural network to model and generalize the linear and the nonlinear patterns of wafer takt times. We then use dynamic time warping to identify the best matching pattern for the wafers being processed, thereby accurately estimating the remaining processing time. The experimental results demonstrated the superior performance of the proposed method in comparison to the existing ones in terms of accuracy. Finally, based on the results of field applications, we found that the proposed method can improve the operational efficiency of AVSs by allowing fast wafer transfer and maximize production throughput by reducing machine idle time.
更多查看译文
关键词
Anticipatory transport system,Automated material handling systems,Autonomous vehicle system,Dynamic time warping,Hybrid forecasting,Time series
AI 理解论文
溯源树
样例
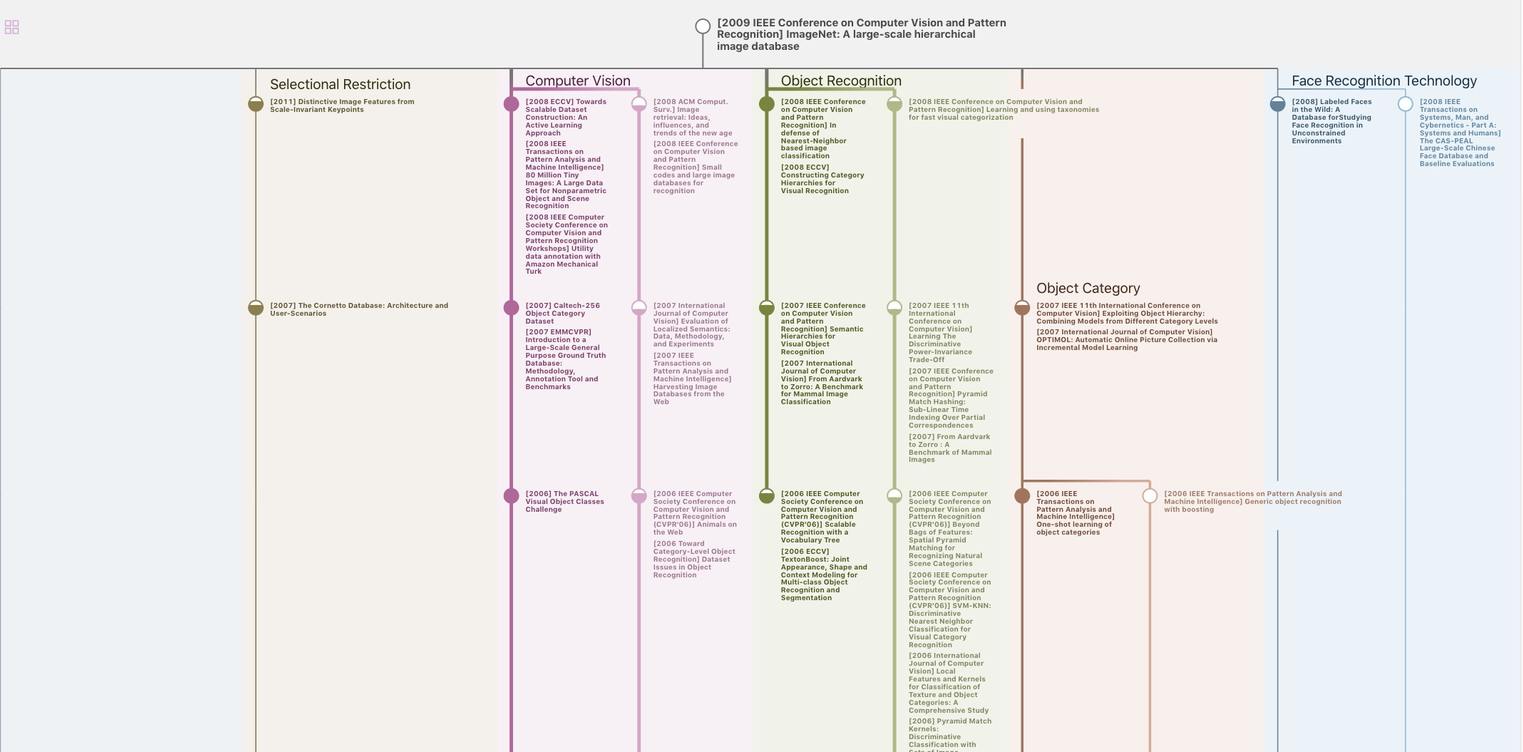
生成溯源树,研究论文发展脉络
Chat Paper
正在生成论文摘要