Ensemble of Convolution Neural Networks for Improving Automatic Modulation Classification Performance
Journal of Science and Technology Issue on Information and Communications Technology(2022)
摘要
This paper investigates convolutional neural networks (CNN) to classify 26 types of signal modulation under the influence of five different fading channels and Gausian noise with SNR from -20 dB to +18 dB. Specifically, five CNN models, including ResNet18, SqueezeNet, GoogleNet, MobileNet, and RepVGG, are taken into account for a accuracy competition to discover the best one. As a result, the SqueezeNet model achieves the highest accuracy of 97.5% for the SNR value of +8~dB. Based on the evaluation results of the single models, we propose an ensemble learning approach, which integrate some robust networks to improve classification accuracy. The numerical results show that ensemble learning can improve the automatic modulation classification accuracy compared to those single models. Specifically, the ensemble learning model gains the accuracy of 52.7% at the SNR of -20 dB and 77% at the SNR of -2 dB. In addition, three types of ensemble methods are considered for analysis and comparison. Consequently, the weighted ensemble provides a better performance in terms of accuracy than unweighted one.
更多查看译文
关键词
modulation,convolution neural networks,classification,neural networks
AI 理解论文
溯源树
样例
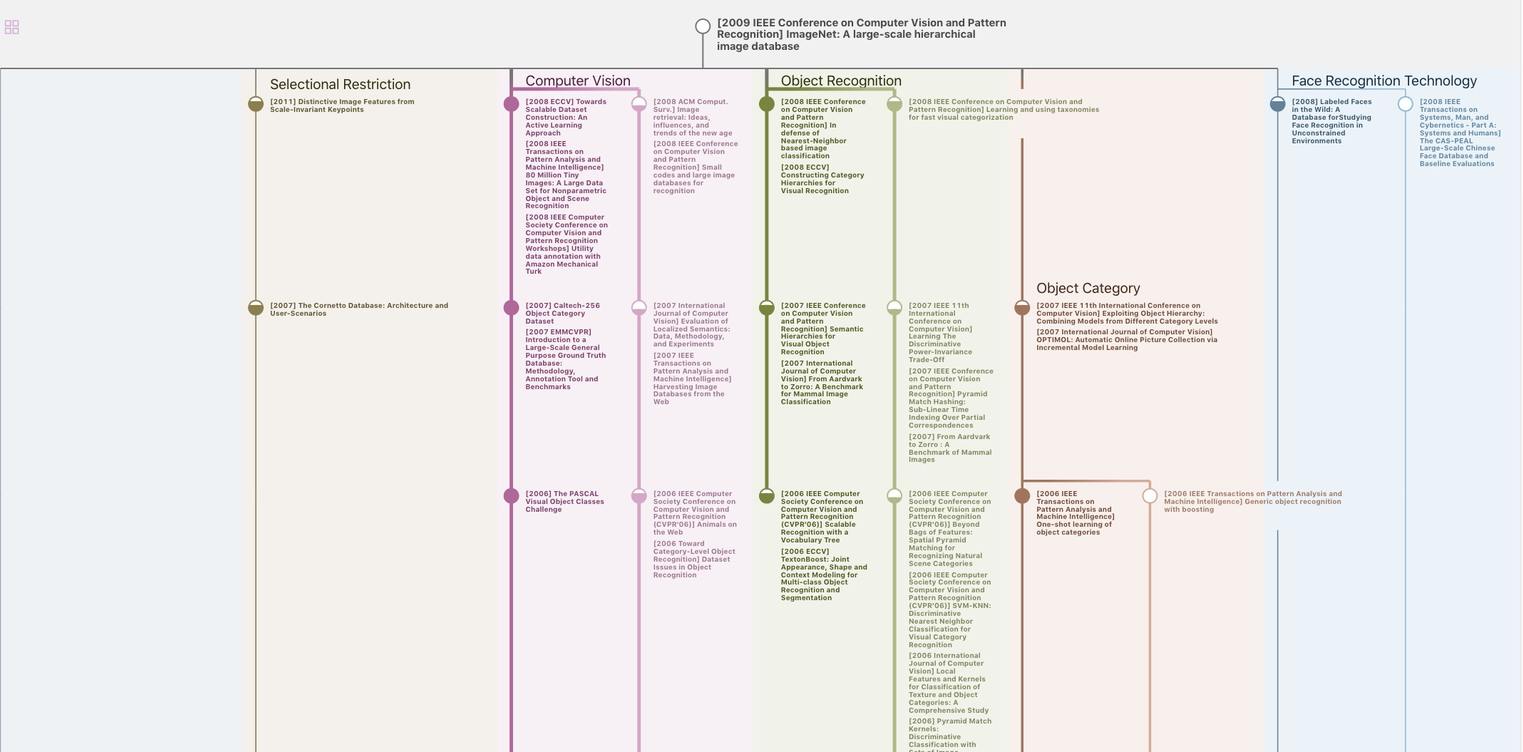
生成溯源树,研究论文发展脉络
Chat Paper
正在生成论文摘要