Interference Constrained Beam Alignment for Time-Varying Channels via Kernelized Bandits
2022 20th International Symposium on Modeling and Optimization in Mobile, Ad hoc, and Wireless Networks (WiOpt)(2022)
摘要
To fully utilize the abundant spectrum resources in millimeter wave (mmWave), Beam Alignment (BA) is necessary for large antenna arrays to achieve large array gains. In practical dynamic wireless environments, channel modeling is challenging due to time-varying and multipath effects. In this paper, we formulate the beam alignment problem as a nonstationary online learning problem with the objective to maximize the received signal strength under interference constraint. In particular, we employ the non-stationary kernelized bandit to leverage the correlation among beams and model the complex beamforming and multipath channel functions. Furthermore, to mitigate interference to other user equipment, we leverage the primal-dual method to design a constrained UCB-type kernelized bandit algorithm. Our theoretical analysis indicates that the proposed algorithm can adaptively adjust the beam in time-varying environments, such that both the cumulative regret of the received signal and constraint violations have sublinear bounds with respect to time. This result is of independent interest for applications such as adaptive pricing and news ranking. In addition, the algorithm assumes the channel is a black-box function and does not require any prior knowledge for dynamic channel modeling, and thus is applicable in a variety of scenarios. We further show that if the information about the channel variation is known, the algorithm will have better theoretical guarantees and performance. Finally, we conduct simulations to highlight the effectiveness of the proposed algorithm.
更多查看译文
关键词
mmWave beam alignment,Gaussian Process bandit,non-stationary bandit
AI 理解论文
溯源树
样例
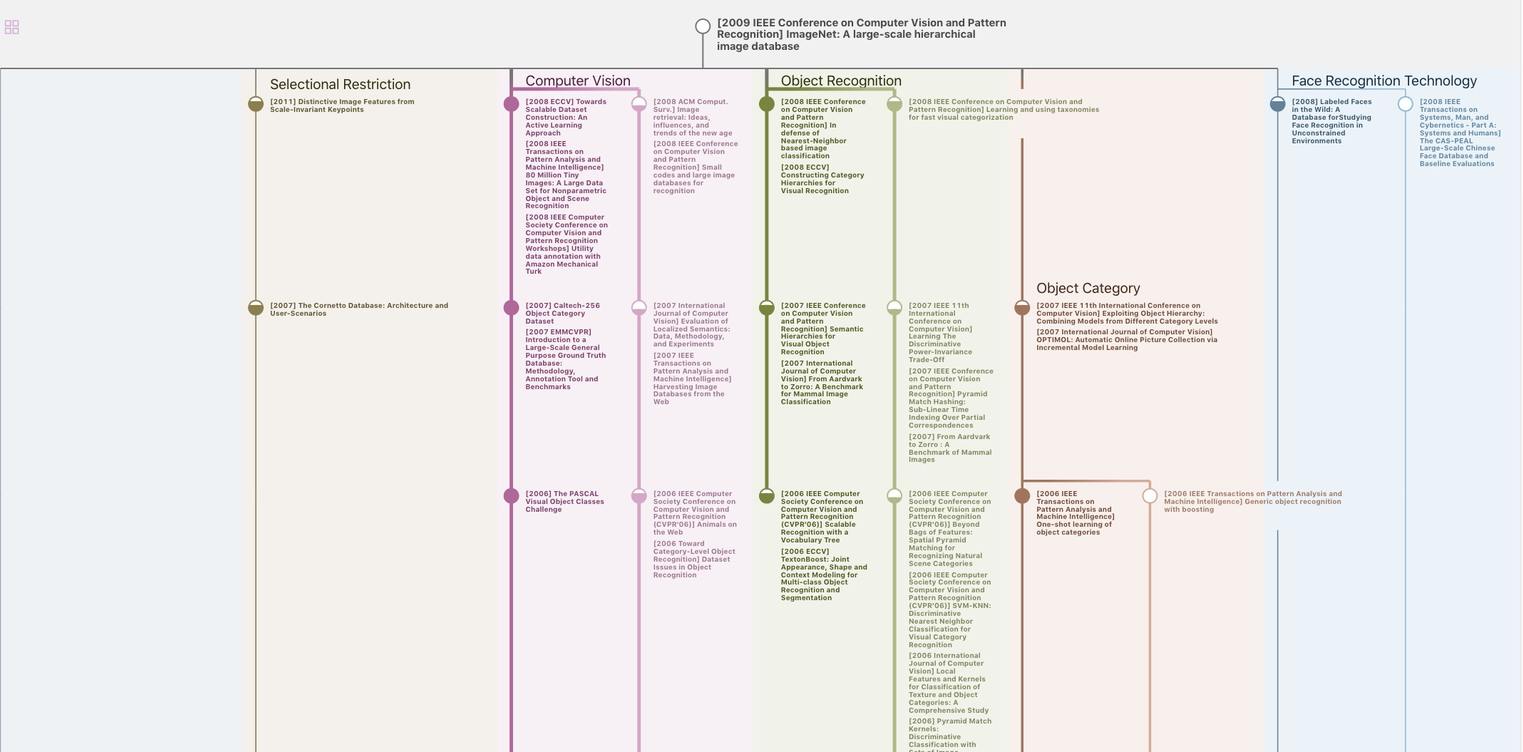
生成溯源树,研究论文发展脉络
Chat Paper
正在生成论文摘要