Fictitious Play and Best-Response Dynamics in Identical Interest and Zero-Sum Stochastic Games.
International Conference on Machine Learning(2022)
摘要
This paper proposes an extension of a popular decentralized discrete-time learning procedure when repeating a static game called fictitious play (FP) (Brown, 1951; Robinson, 1951) to a dynamic model called discounted stochastic game (Shapley, 1953). Our family of discrete-time FP procedures is proven to converge to the set of stationary Nash equilibria in identical interest discounted stochastic games. This extends similar convergence results for static games (Monderer & Shapley, 1996a). We then analyze the continuous-time counterpart of our FP procedures, which include as a particular case the best-response dynamic introduced and studied by Leslie et al. (2020) in the context of zero-sum stochastic games. We prove the converge of this dynamics to stationary Nash equilibria in identical-interest and zero-sum discounted stochastic games. Thanks to stochastic approximations, we can infer from the continuous-time convergence some discrete time results such as the convergence to stationary equilibria in zero-sum and team stochastic games (Holler, 2020).
更多查看译文
关键词
zero sum stochastic games,fictitious play,best-response
AI 理解论文
溯源树
样例
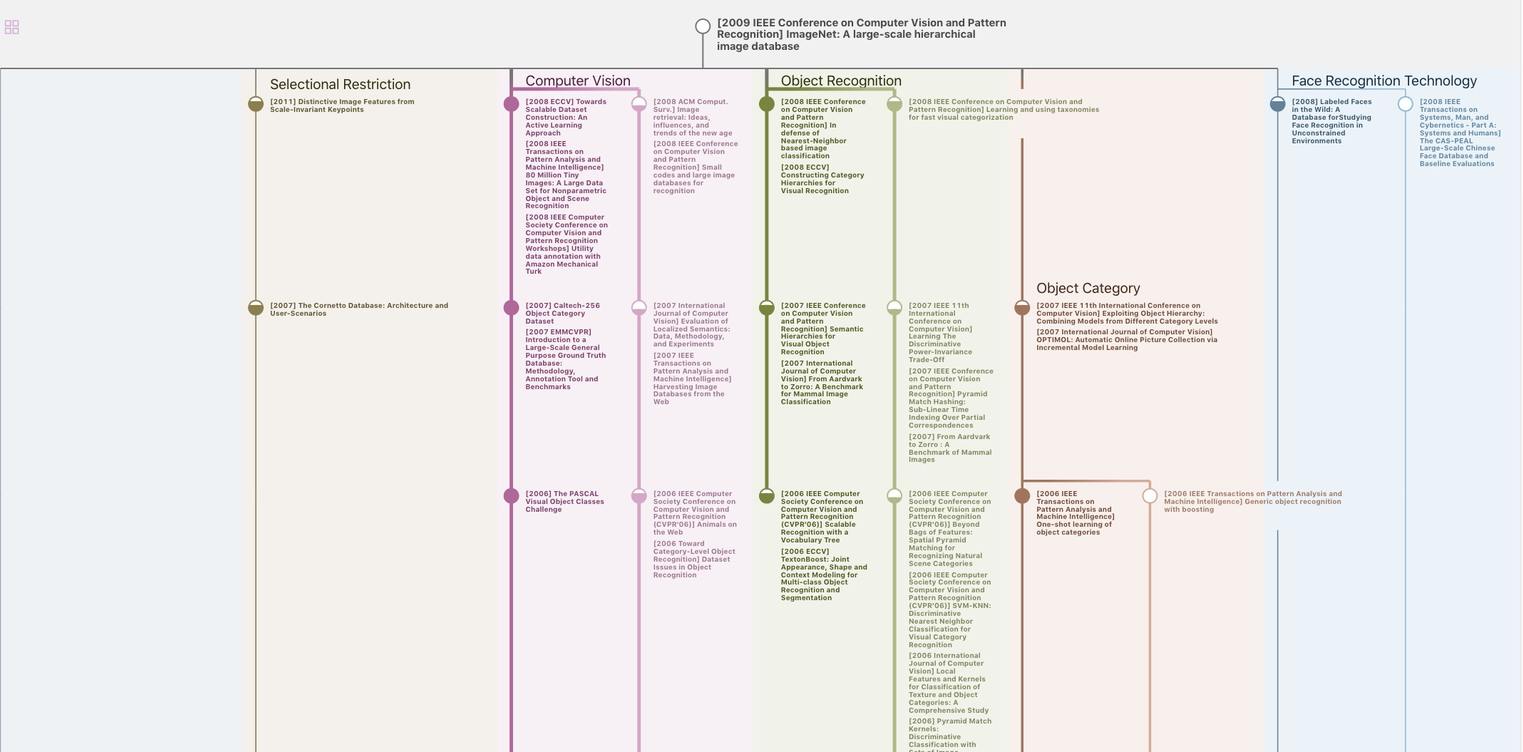
生成溯源树,研究论文发展脉络
Chat Paper
正在生成论文摘要