A dual-interpolator method for improving parallel MRI reconstruction
Magnetic Resonance Imaging(2022)
摘要
Autocalibration signal is acquired in the k-space-based parallel MRI reconstruction for estimating interpolation coefficients and reconstructing missing unacquired data. Many ACS lines can suppress aliasing artifacts and noise by covering the low-frequency signal region. However, more ACS lines will delay the data acquisition process and therefore elongate the scan time. Furthermore, a single interpolator is often used for recovering missing k-space data, and model error may exist if the single interpolator size is not selected appropriately. In this work, based on the idea of the disagreement-based semi-supervised learning, a dual-interpolator strategy is proposed to collaboratively reconstruct missing k-space data. Two interpolators with different sizes are alternatively applied to estimate and re-estimate missing data in k-space. The disagreement between two interpolators is converged and real missing values are co-estimated from two views. The experimental results show that the proposed method outperforms GRAPPA, SPIRiT, and Nonlinear GRAPPA methods using relatively low number of ACS data, and reduces aliasing artifacts and noise in reconstructed images.
更多查看译文
关键词
Parallel MRI reconstruction,Co-training,Disagreement-based semi-supervised learning,Infinite impulse response filter
AI 理解论文
溯源树
样例
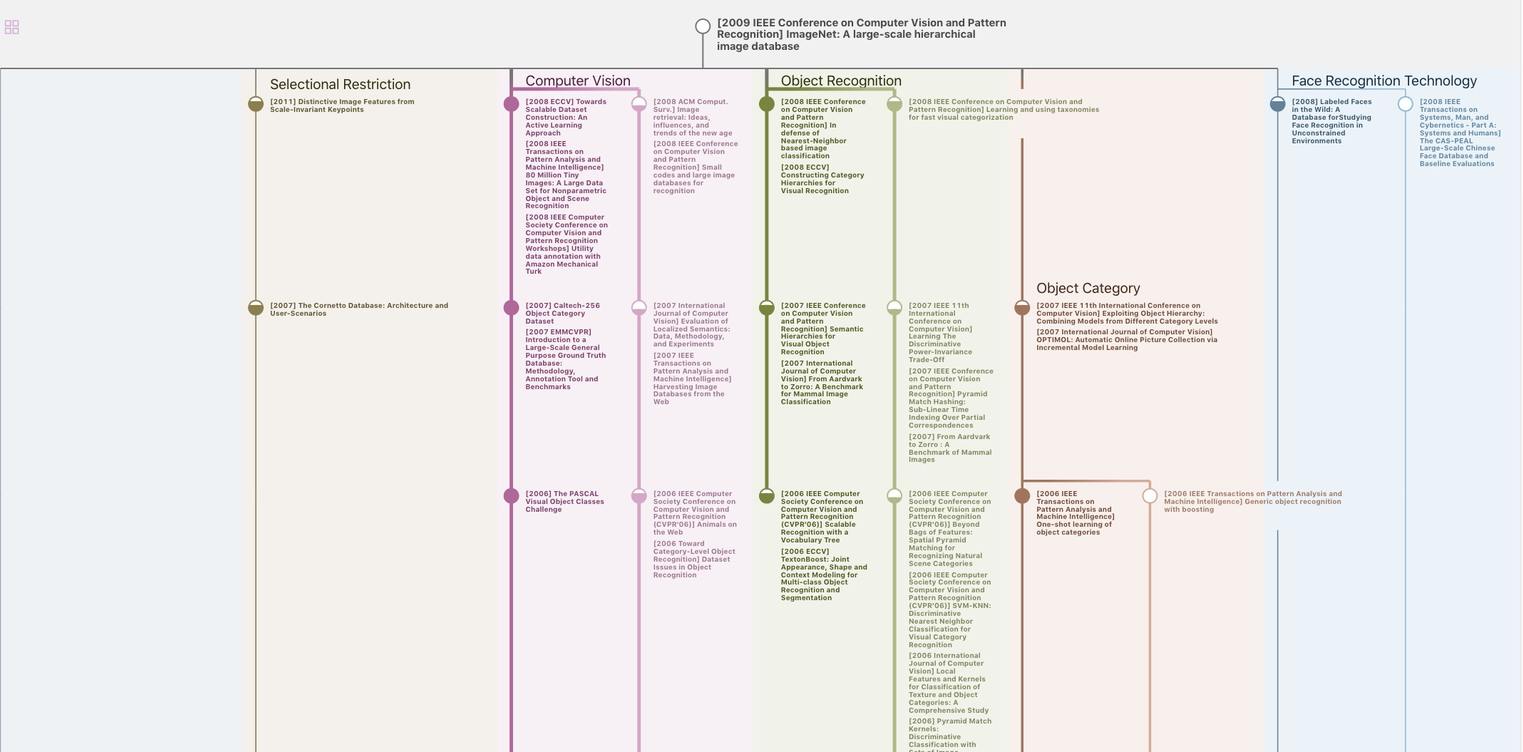
生成溯源树,研究论文发展脉络
Chat Paper
正在生成论文摘要