On the Importance of Application-Grounded Experimental Design for Evaluating Explainable ML Methods
AAAI 2024(2024)
摘要
Most existing evaluations of explainable machine learning (ML) methods rely on simplifying assumptions or proxies that do not reflect real-world use cases; the handful of more robust evaluations on real-world settings have shortcomings in their design, generally leading to overestimation of methods' real-world utility. In this work, we seek to address this by conducting a study that evaluates post-hoc explainable ML methods in a setting consistent with the application context and provide a template for future evaluation studies. We modify and improve a prior study on e-commerce fraud detection by relaxing the original work's simplifying assumptions that departed from the deployment context. Our study finds no evidence for the utility of the tested explainable ML methods in the context, which is a drastically different conclusion from the earlier work. This highlights how seemingly trivial experimental design choices can yield misleading conclusions about method utility. In addition, our work carries lessons about the necessity of not only evaluating explainable ML methods using tasks, data, users, and metrics grounded in the intended application context but also developing methods tailored to specific applications, moving beyond general-purpose explainable ML methods.
更多查看译文
关键词
General
AI 理解论文
溯源树
样例
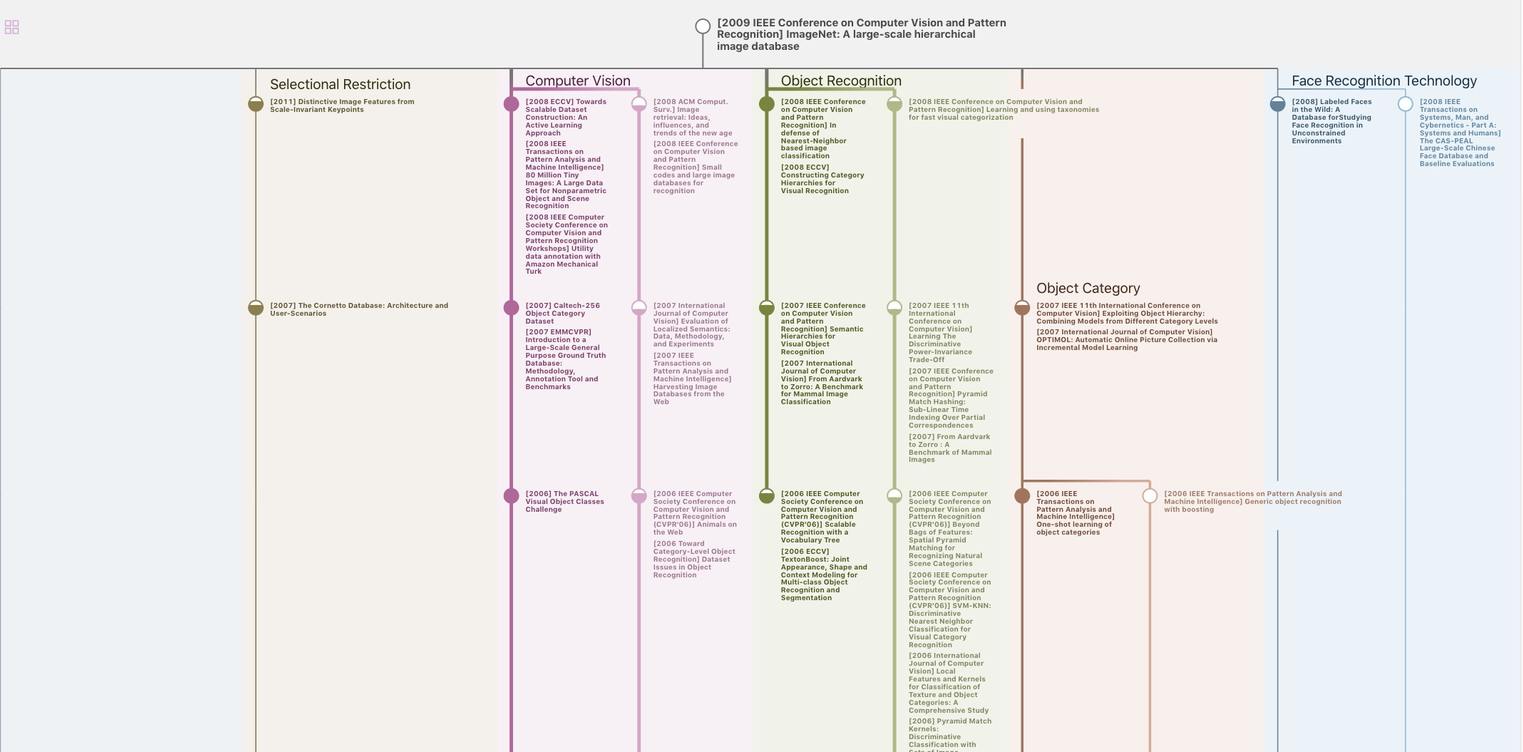
生成溯源树,研究论文发展脉络
Chat Paper
正在生成论文摘要