Offline RL for Natural Language Generation with Implicit Language Q Learning
ICLR 2023(2022)
摘要
Large language models distill broad knowledge from text corpora. However, they can be inconsistent when it comes to completing user specified tasks. This issue can be addressed by finetuning such models via supervised learning on curated datasets, or via reinforcement learning. In this work, we propose a novel offline RL motivated method, implicit language Q-learning (ILQL), designed for use on language models, that combines both the flexible utility optimization framework of traditional RL algorithms with supervised learning's ability to leverage existing data and its simplicity and stability. Our method, based on dynamic programming, employs a blend of value conservatism alongside an implicit dataset support constraint in learning value functions, which are then used to guide language model generations towards maximizing utility. In addition to empirically validating ILQL, we present a detailed empirical analysis of situations where offline RL can be useful in natural language generation settings, demonstrating how it can be a more effective utility optimizer than prior approaches for end-to-end dialogue, and how it can effectively optimize high variance reward functions based on subjective judgement, such as whether to label a comment as an example of toxic speech or not.
更多查看译文
关键词
offline reinforcement learning,natural language processing,dialogue,controlled generation
AI 理解论文
溯源树
样例
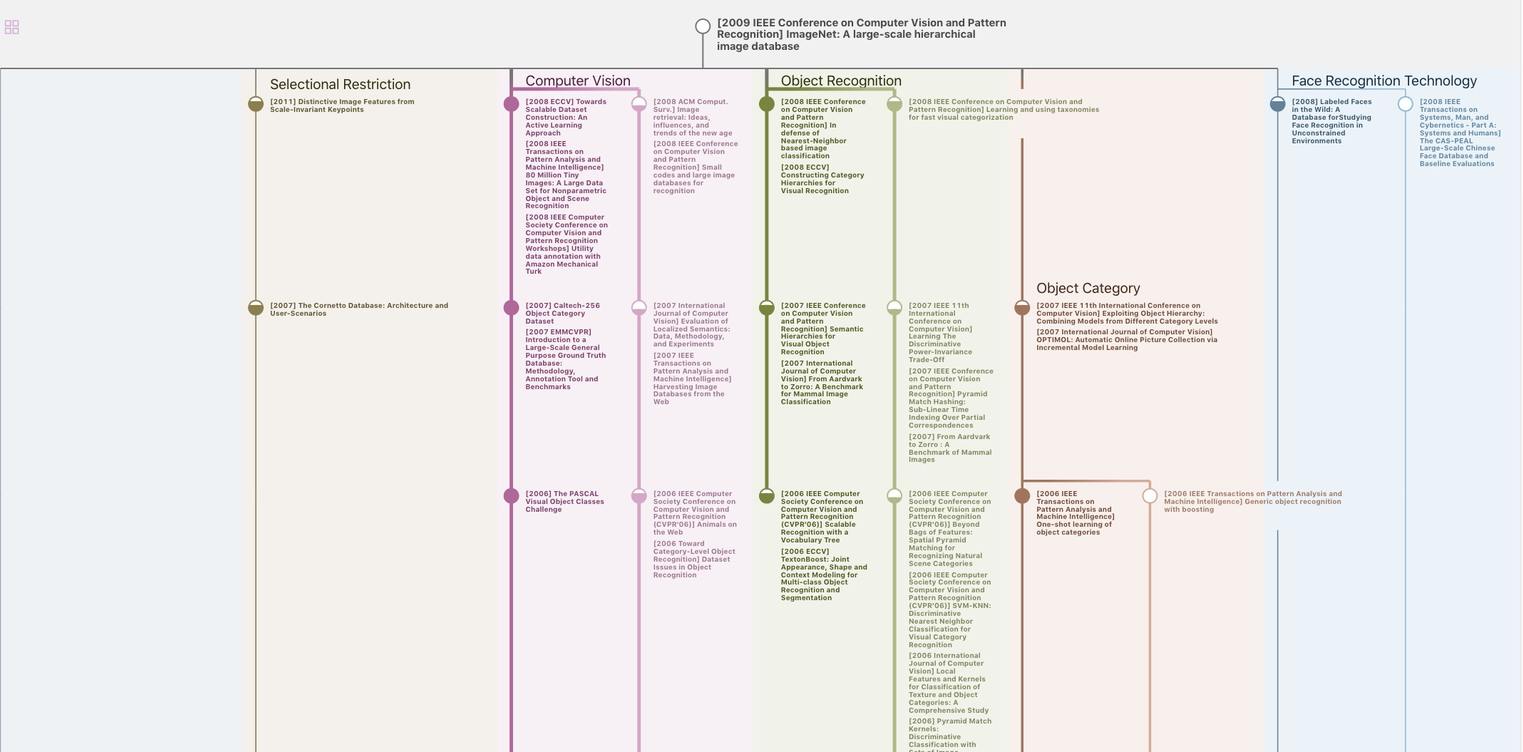
生成溯源树,研究论文发展脉络
Chat Paper
正在生成论文摘要