On the interpretation of deep learning models in bearing vibration diagnostics
Proceedings of the Annual Conference of the Prognostics and Health Management Society(2021)
摘要
In recent years, data-driven techniques such as deep learning (DL), have been widely represented in the literature in the field of bearing vibration condition monitoring. While these approaches achieve excellent performance in detecting bearing faults on controlled laboratory datasets, there is little information available on their applicability to more realistic working conditions. One challenge of these data-driven approaches is that they can learn non-classical features unrelated to the physical defect, making their generalizability debatable. To overcome the challenge of generalizability in DL models, we aim to first understand the underlying representation that the network uses to classify different bearing defects. Having an interpretable DL model may give us hints on how to increase its applicability by, e.g., data augmentation, changing input representations or adapting model architectures. To benefit from advances in interpretability in DL methods from computer vision, we first transform the vibration signal into an image. We evaluate a common input transformation, namely the spectrogram. Subsequently, the representations that the network has learnt are evaluated. We use the Grad-CAM algorithm together with signal modifications to evaluate which parts of the input signal contribute to class attribution. Our results show that the network learns signal features related to the transfer path, the physical properties of the test setup, rather than picking up classical features having a physical relation with the defect. Given that a transfer path is very machine specific, this could be an explanation for the lack of scalability of DL methods. To improve the generalizability of DL methods on bearing vibration analysis, the competing dominant machine specific features should be eliminated from the input representation. These results highlight the importance of combining domain expertise with data-driven approaches.
更多查看译文
AI 理解论文
溯源树
样例
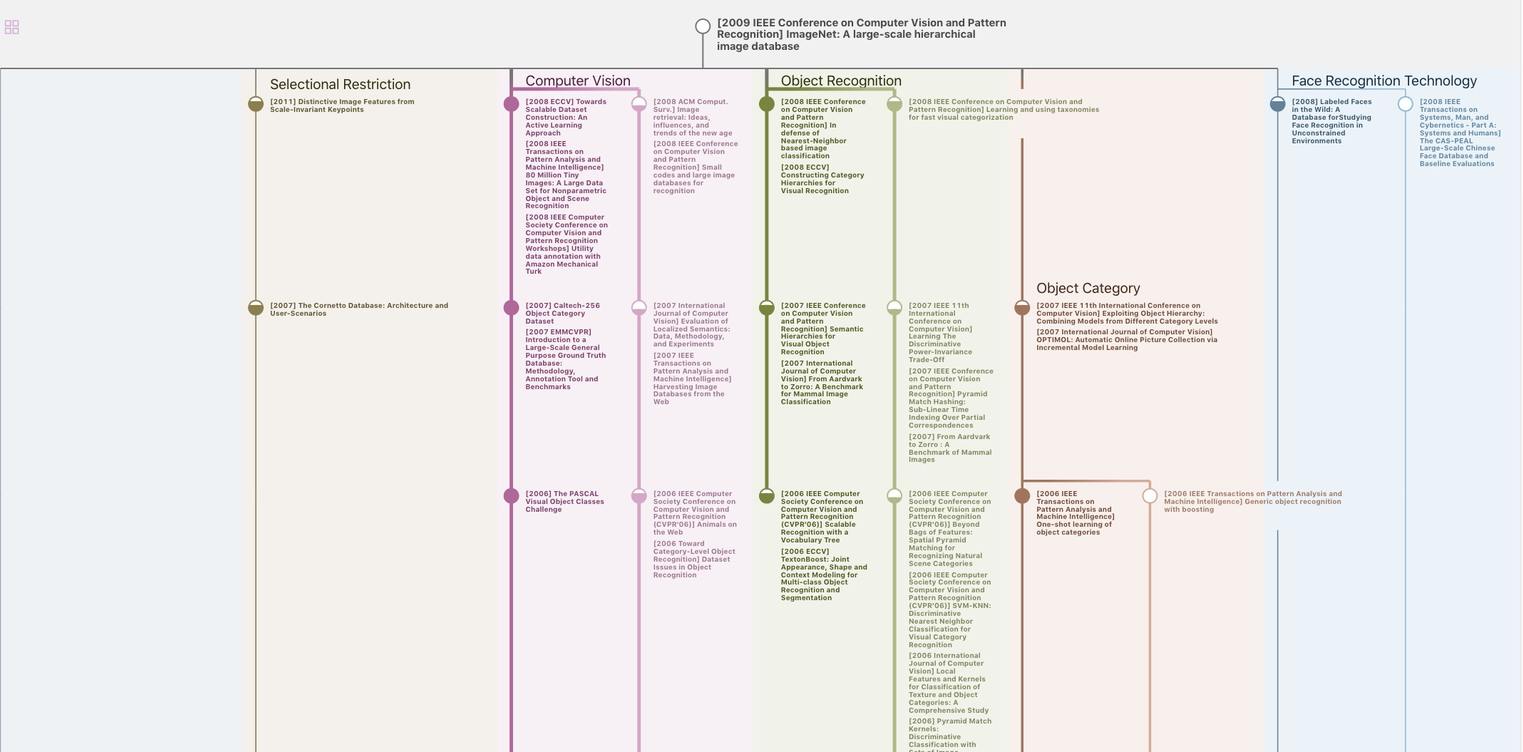
生成溯源树,研究论文发展脉络
Chat Paper
正在生成论文摘要