Segmentation of waterbodies in remote sensing images using deep stacked ensemble model
Applied Soft Computing(2022)
摘要
Identifying surface water resources is considered as one of the principal applications of remote sensing image analysis that plays a crucial role in controlling optimal use of these resources, and preventing floods and crises such as drought. Traditional machine learning methods for extracting waterbodies require complex spectral analysis and selection of features based on previous knowledge. Although applying deep learning-based approaches, which has been considered in recent years, has eliminated the necessity of extracting manual features, they require too many training data and computational resources to achieve high performance. Consequently, each presented deep architecture can detect some of the existing patterns in the predefined conditions. This paper trains and optimizes three robust deep architectures, presented in various fields, using surface water data, and combines their results to achieve a robust model for detecting surface water. To this end, a deep hybrid architecture called ”deep stacked ensemble model” is employed on the outputs of three independent deep sub-models and extracts the final segmentation mask of the water areas more accurately. We evaluated our proposed model on a water body detection dataset provided by artificial intelligence crowd landsat (AIcrowd11https://www.aicrowd.com/challenges/ai-for-good-ai-blitz-3/problems/lndst/leaderboards. LNDST) challenge. The proposed technique improves the semantic segmentation performance and surpasses state-of-the-art results.
更多查看译文
关键词
Waterbody segmentation,Remote sensing image analysis,Deep stacked ensemble model,U-net,Deep learning
AI 理解论文
溯源树
样例
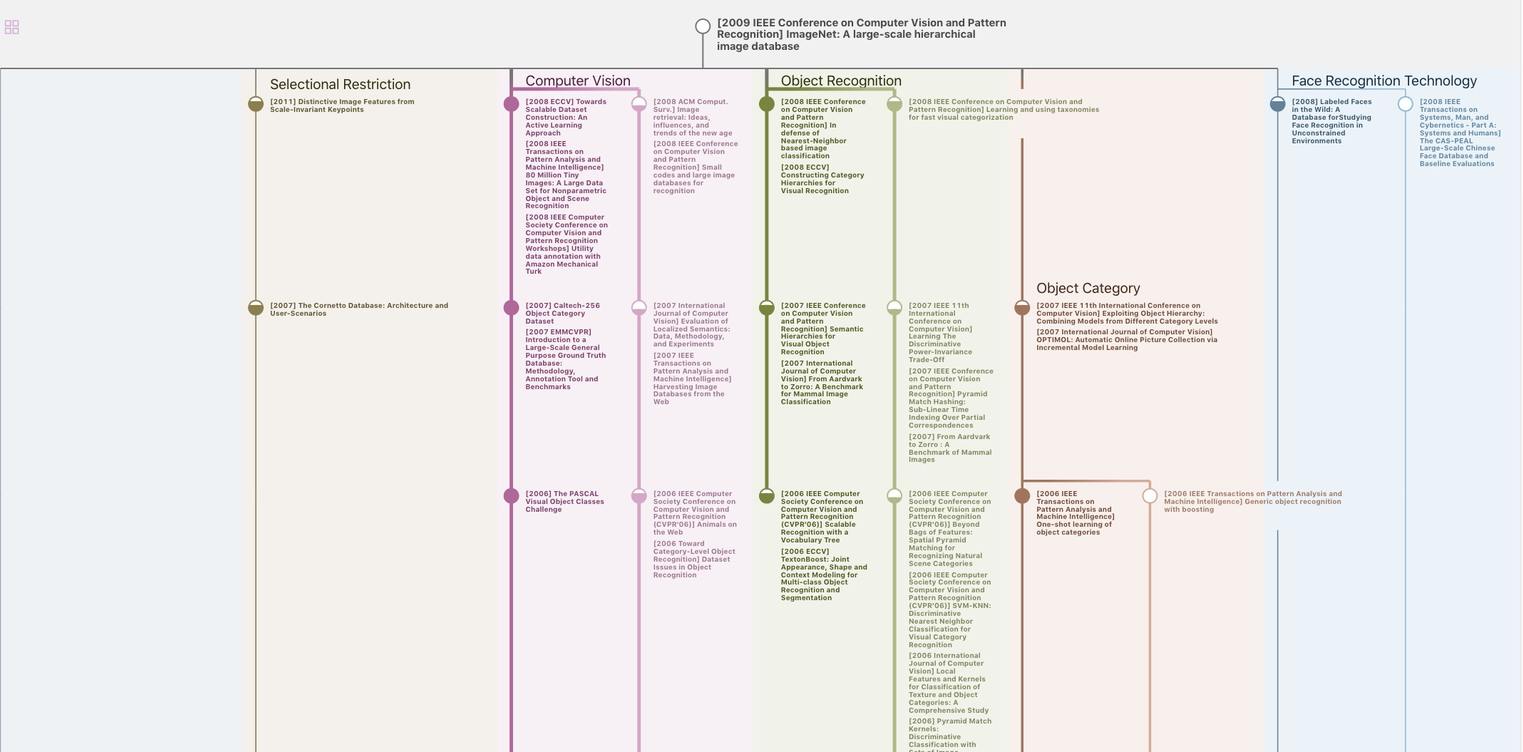
生成溯源树,研究论文发展脉络
Chat Paper
正在生成论文摘要