ATF: An Alternating Training Framework for Weakly Supervised Face Alignment
IEEE Transactions on Multimedia(2023)
摘要
In recent years, various face-landmark datasets have been published. Intuitively, it is significant to integrate multiple labeled datasets to achieve higher performance. Due to the different annotation schemes of datasets, it is hard to directly train models using them together. Although numerous efforts have been made in the joint use of datasets, there remain three shortages in previous methods,
i.e.
, additional computation, limitation of the markups scheme, and limited support for the regression method. To solve the above issues, we proposed a novel
Alternating Training Framework
(ATF), which leverages the similarity and diversity across multiple datasets for a more robust detector. ATF mainly contains two sub-modules:
Alternating Training with Decreasing Proportions
(ATDP) and
Mixed Branch Loss
(
$\mathcal {L}_{MB}$
). In particular, ATDP trains multiple datasets simultaneously via a weakly supervised way to take advantage of the diversity among them, and
$\mathcal {L}_{MB}$
utilizes similar landmark pairs to constrain different branches of the corresponding datasets. Besides, we extend the framework to easily handle three situations: single target detector, joint detector, and novel detector. Extensive experiments demonstrate the effectiveness of our framework for both heatmap-based and direct coordinate regression. Moreover, we have achieved a joint detector that outperforms state-of-the-art methods on each benchmark.
更多查看译文
关键词
Face alignment,multi-task learning,weakly supervised
AI 理解论文
溯源树
样例
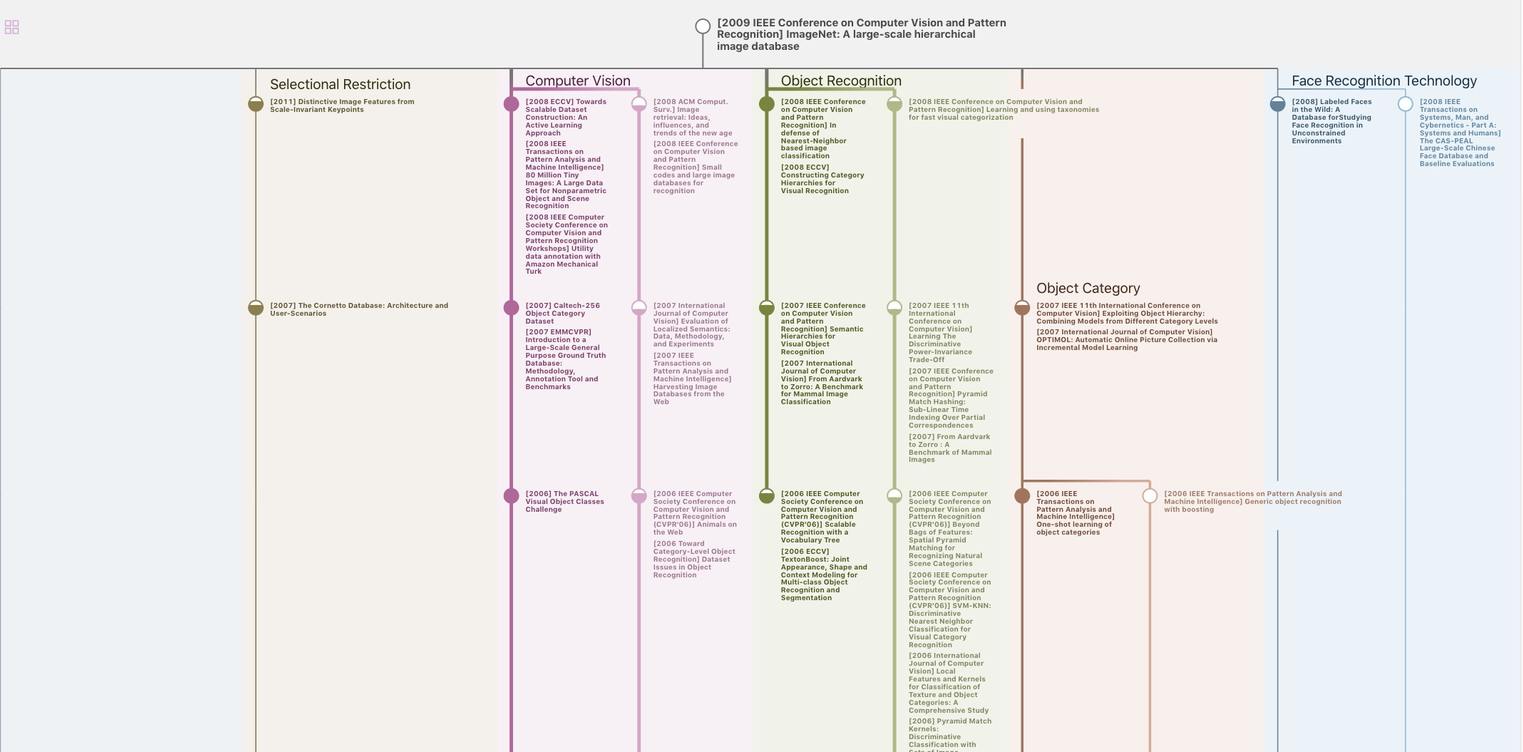
生成溯源树,研究论文发展脉络
Chat Paper
正在生成论文摘要