Probabilistic Attribute Tree Structured Convolutional Neural Networks for Facial Expression Recognition in the Wild
IEEE Transactions on Affective Computing(2023)
摘要
Very recent work has demonstrated tremendous improvements in facial expression recognition (FER) on laboratory-controlled datasets. However, recognizing facial expressions under in-the-wild conditions still remains challenging, especially on unseen subjects due to high inter-subject variations. In this paper, we propose a novel Probabilistic Attribute Tree Convolutional Neural Network (PAT-CNN) to explicitly deal with large intra-class variations caused by identity-related attributes, e.g., age, race, and gender. Specifically, a PAT module with an associated PAT loss is proposed to learn features in a hierarchical tree structure organized according to identity-related attributes, where the final features are less affected by the attributes. Then, expression-related features are extracted from leaf nodes. Samples are probabilistically assigned to tree nodes at different levels such that expression-related features can be learned from all samples weighted by probabilities. Furthermore, the proposed PAT-CNN can be learned from limited attribute-annotated samples to make the best use of available data. Experimental results on four spontaneous facial expression datasets, i.e., RAF-DB, SFEW, ExpW, and FER-2013, have demonstrated that the proposed PAT-CNN achieves the best performance when compared to state-of-the-art methods by explicitly modeling attributes. Impressively, a single model PAT-CNN achieves the best performance on the SFEW test dataset when compared to the state-of-the-art methods using an ensemble of hundreds of CNNs.
更多查看译文
关键词
Convolutional neural network,facial expression recognition in the wild,probabilistic attribute tree
AI 理解论文
溯源树
样例
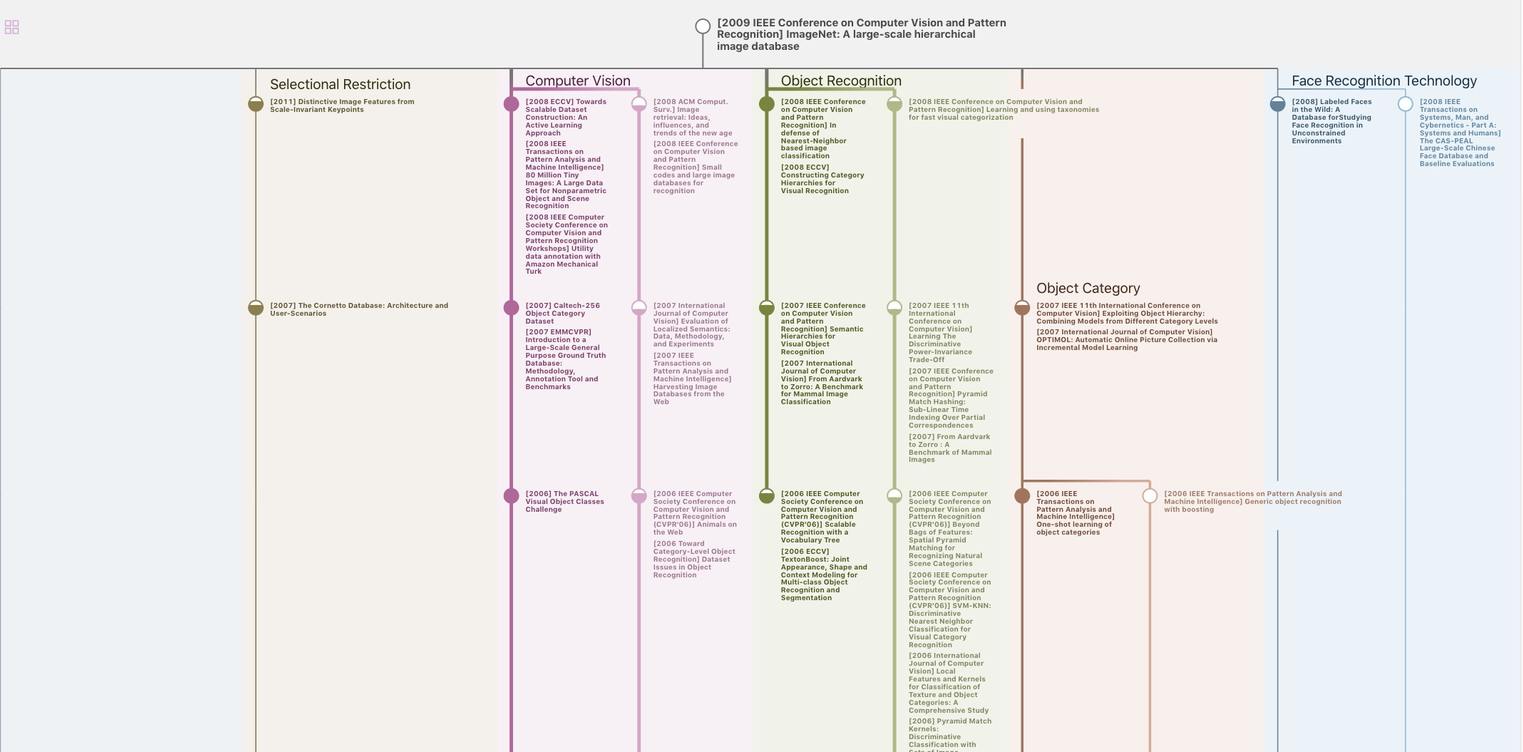
生成溯源树,研究论文发展脉络
Chat Paper
正在生成论文摘要