Safe Building HVAC Control via Batch Reinforcement Learning
IEEE transactions on sustainable computing(2022)
摘要
In this paper, we study safe building HVAC control via batch reinforcement learning. Random exploration in building HVAC control is infeasible due to safety considerations. However, diverse states are necessary for RL algorithms to learn useful policies. To enable \emph{safety} during exploration, we propose guided exploration by adding a Gaussian noise to a hand-crafted rule-based controller. Adjusting the variance of the noise provides a tradeoff between the \emph{diversity} of the dataset and the \emph{safety}. We apply Conservative Q Learning (CQL) to learn a policy. CQL ensures that the trained policy stays within the policy distribution used to collect the dataset, thereby guarantees safety at deployment. To select the optimal policy during the offline training, we apply model-based performance evaluation. We use the widely adopted CityLearn testbed to evaluate the performance of our proposed method. Compared with a rule-based controller, our approach obtains $12\%\sim35\%$ reduction in ramping, $3\%\sim10\%$ reduction in 1-load factor, $3\%\sim8\%$ reduction in daily peak at deployment with less than $10\%$ performance degradation during the exploration. On the contrary, the performance degradation of the state-of-the-art online reinforcement learning algorithm during exploration is around $8\%\sim 18\%$ . It also fails to surpass the performance of the rule-based controller at deployment.
更多查看译文
关键词
Batch reinforcement learning,safe building HVAC control,model-based offline performance evaluation
AI 理解论文
溯源树
样例
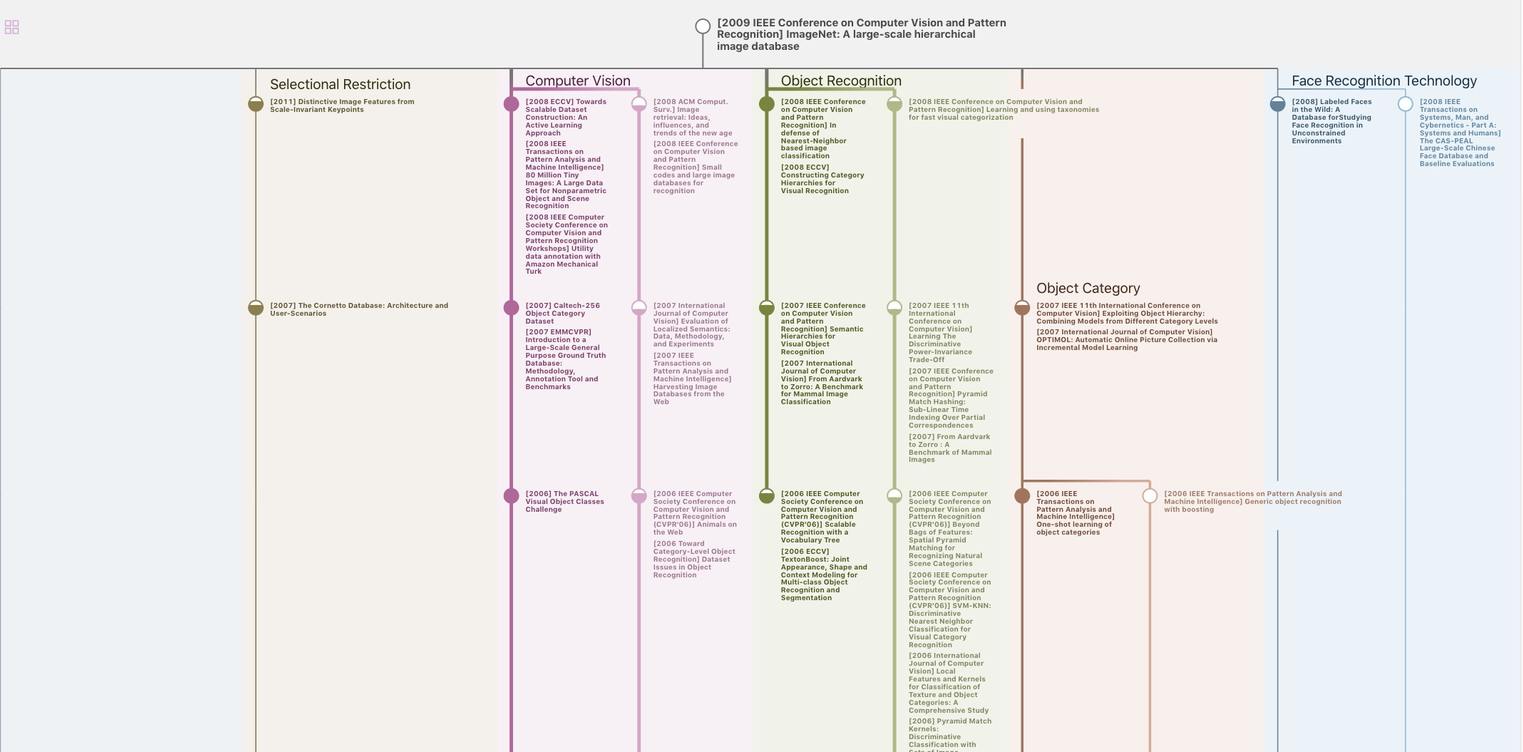
生成溯源树,研究论文发展脉络
Chat Paper
正在生成论文摘要