Cross-Platform Multimodal Misinformation: Taxonomy, Characteristics and Detection for Textual Posts and Videos
International Conference on Web and Social Media (ICWSM)(2022)
Abstract
Social media posts that direct users to YouTube videos are one of the most effective techniques for spreading misinformation. However, it has been observed that such posts rarely get deleted or flagged. Since multi-modal misinformation that leads to compelling videos has more impact than using just textual content, it is important to characterize and detect such textual post and video pairs to prevent users from becoming victims of misinformation. To address this gap, we build a taxonomy of how links to YouTube videos are used on social media platforms. We then use pairs of posts and videos annotated with this taxonomy to test several classification models built using cross-platform features. Our work reveals several characteristics of post-video pairs, in terms of how posts and videos are related to each other, the type of content they share, and their collective outcome. In addition, we find that traditional approaches to misinformation detection that rely only on text from posts miss a significant number of post-video pairs that contain misinformation. More importantly, we find that to reduce the spread of misinformation via post-video pairs, classifiers would be more effective if they are designed to use data and features from multiple diverse platforms.
MoreTranslated text
Key words
textual posts,videos,cross-platform
AI Read Science
Must-Reading Tree
Example
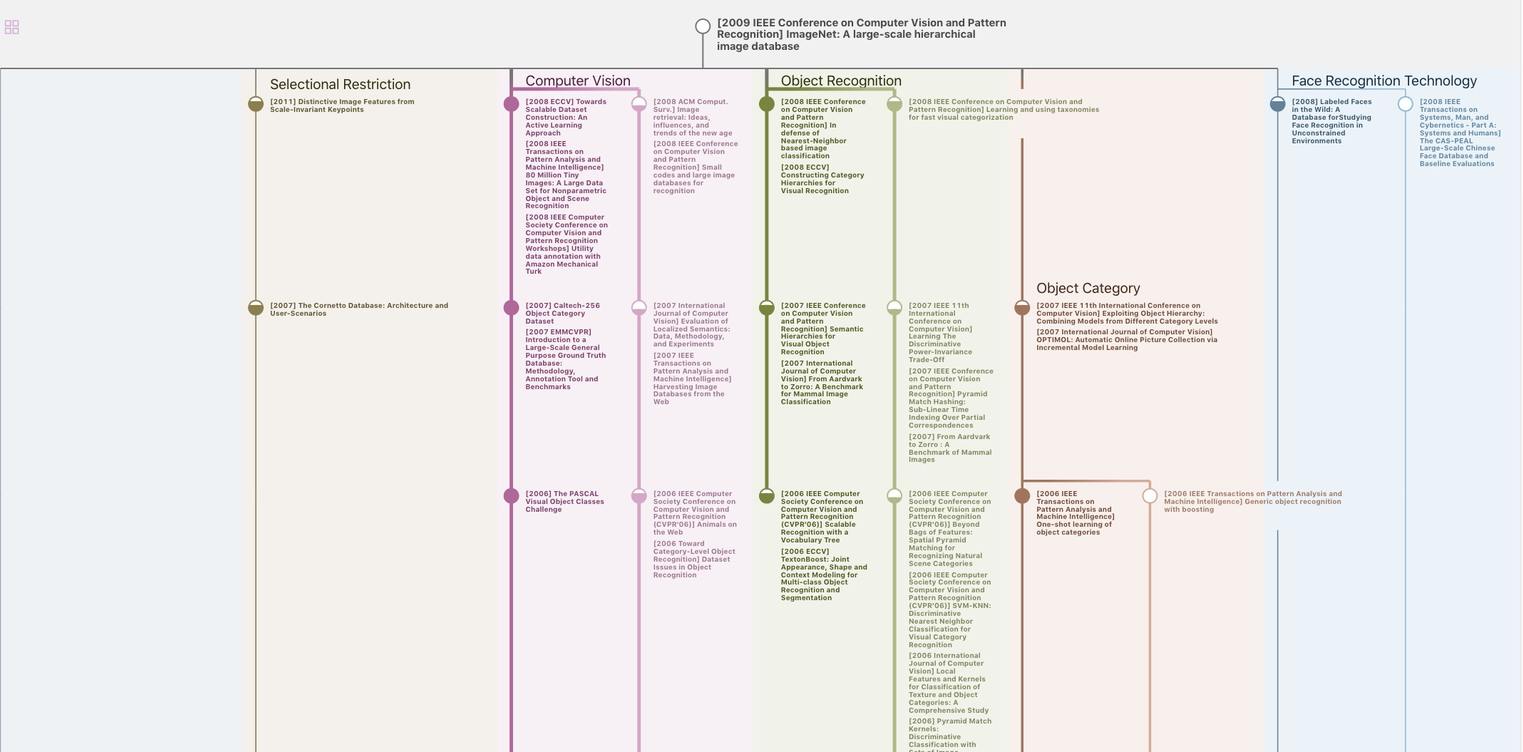
Generate MRT to find the research sequence of this paper
Chat Paper
Summary is being generated by the instructions you defined