Ensemble-Based Feature Selection With Long Short-Term Memory for Classification of Network Intrusion
Advances in Social Networking and Online CommunitiesE-Collaboration Technologies and Strategies for Competitive Advantage Amid Challenging Times(2021)
摘要
This chapter presents an ensemble-based feature selection with long short-term memory (LSTM) model. A deep recurrent learning model is proposed for classifying network intrusion. This model uses ensemble-based feature selection (EFS) for selecting the appropriate features from the dataset and long short-term memory for the classification of network intrusions. The EFS combines five feature selection techniques, namely information gain, gain ratio, chi-square, correlation-based feature selection, and symmetric uncertainty-based feature selection. The experiments were conducted using the standard benchmark NSL-KDD dataset and implemented using tensor flow and python. The proposed model is evaluated using the classification performance metrics and also compared with all the 41 features without any feature selection as well as with each individual feature selection technique and classified using LSTM. The performance study showed that the proposed model performs better, with 99.8% accuracy, with a higher detection and lower false alarm rates.
更多查看译文
关键词
feature selection,classification,ensemble-based,short-term
AI 理解论文
溯源树
样例
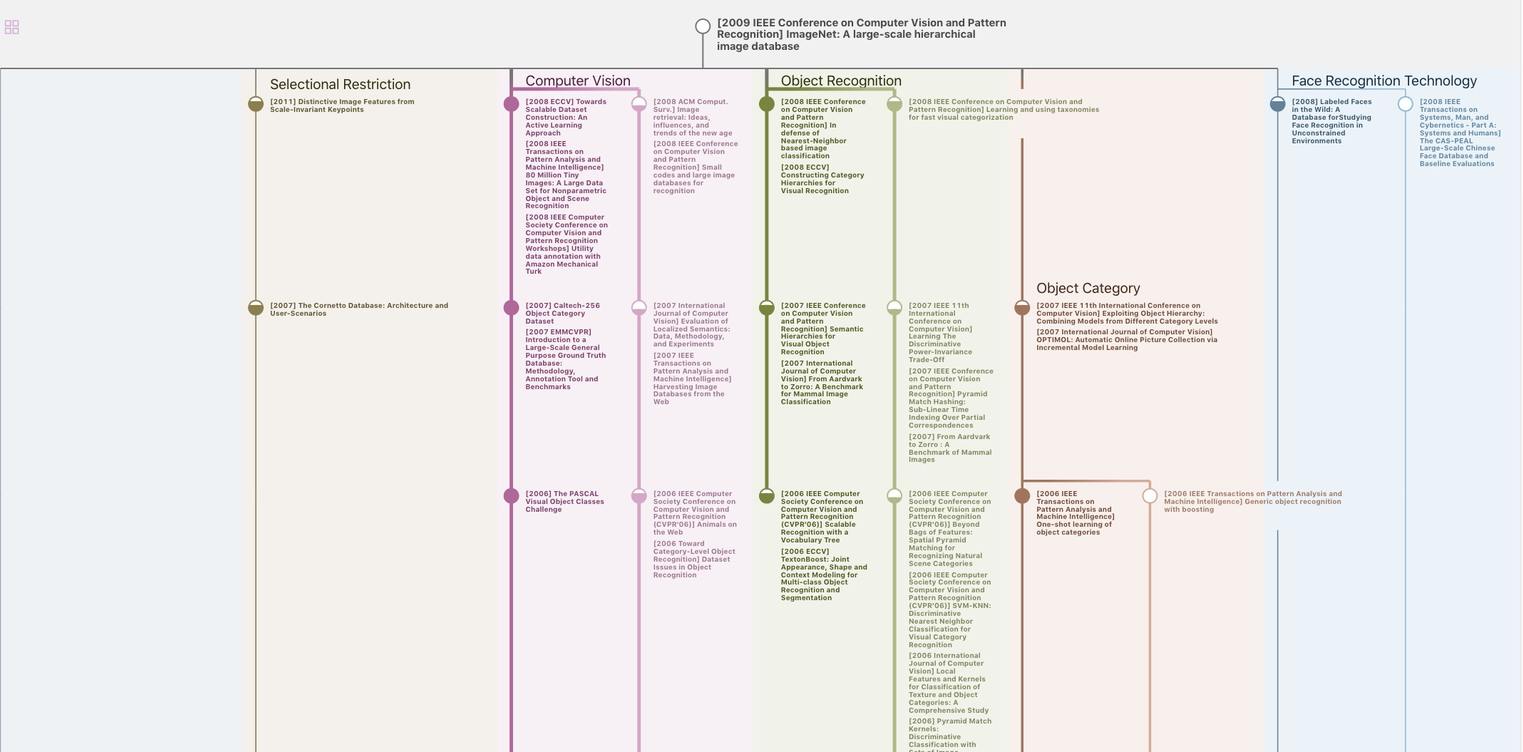
生成溯源树,研究论文发展脉络
Chat Paper
正在生成论文摘要