Challenges and Limitations of Geospatial Data and Analyses in the Context of COVID-19
Mapping COVID-19 in Space and TimeHuman Dynamics in Smart Cities(2021)
摘要
The global COVID-19 pandemic has infected public consciousness with a new appreciation of geospatial analyses in public health. Not since John Snow’s 1854 cholera map of London has a public health crisis brought so much attention to geospatial data, and their importance to understanding, predicting, and preventing the spread of disease. Although high profile models and visualization dashboards of COVID-19 have increased awareness of geospatial data and analytics in public health, they have also amplified concerns regarding data issues, including questions of reproducibility and replicability (R&R), provenance, scale of analysis, fitness for use, trust, privacy, and uncertainty. The following chapter sections discuss these data issues and their implications for decision making during the COVID-19 pandemic. R&R and provenance—Many complex geospatial R&R issues can be simplified by a focus on who has (or should have) access to associated geospatial provenance information which is here defined. Human mobility data—Non-traditional data sources, such as social media, can augment official reporting sources and provide insight into mobility and social distancing behaviors. We discuss how to incorporate human mobility data selectively, while maintaining anonymity, with official data sources during emergency situations. Scales of analysis—Although several models and geo-visualization tools are providing information about disease spread based on mobility, network connectivity, and/or socio-economic conditions, these models and tools rarely shed light about the impact of the spatial scale at which data are available and models are implemented. The widely adopted practice in the U.S. of mapping data to counties may provide false impressions to policy makers and the public regarding the locations at greatest and least risk. Given the dynamic nature of the disease, temporal scale is also crucial to understanding the implications of models. Understanding uncertainty in projection models—A key issue in infectious disease epidemiological models is the characterization and quantification of uncertainty in predictions. Uncertainties come from assumptions on model parameters, model estimation, and both systematic and random uncertainties from input data, including temporal lags in reporting. Model choice may add to overall uncertainties in the predicted numbers. Since these forecasts have an immense impact on public health policy and individual risk-based decision making, it is paramount to understand and convey the sources and nature of uncertainties.
更多查看译文
关键词
geospatial data
AI 理解论文
溯源树
样例
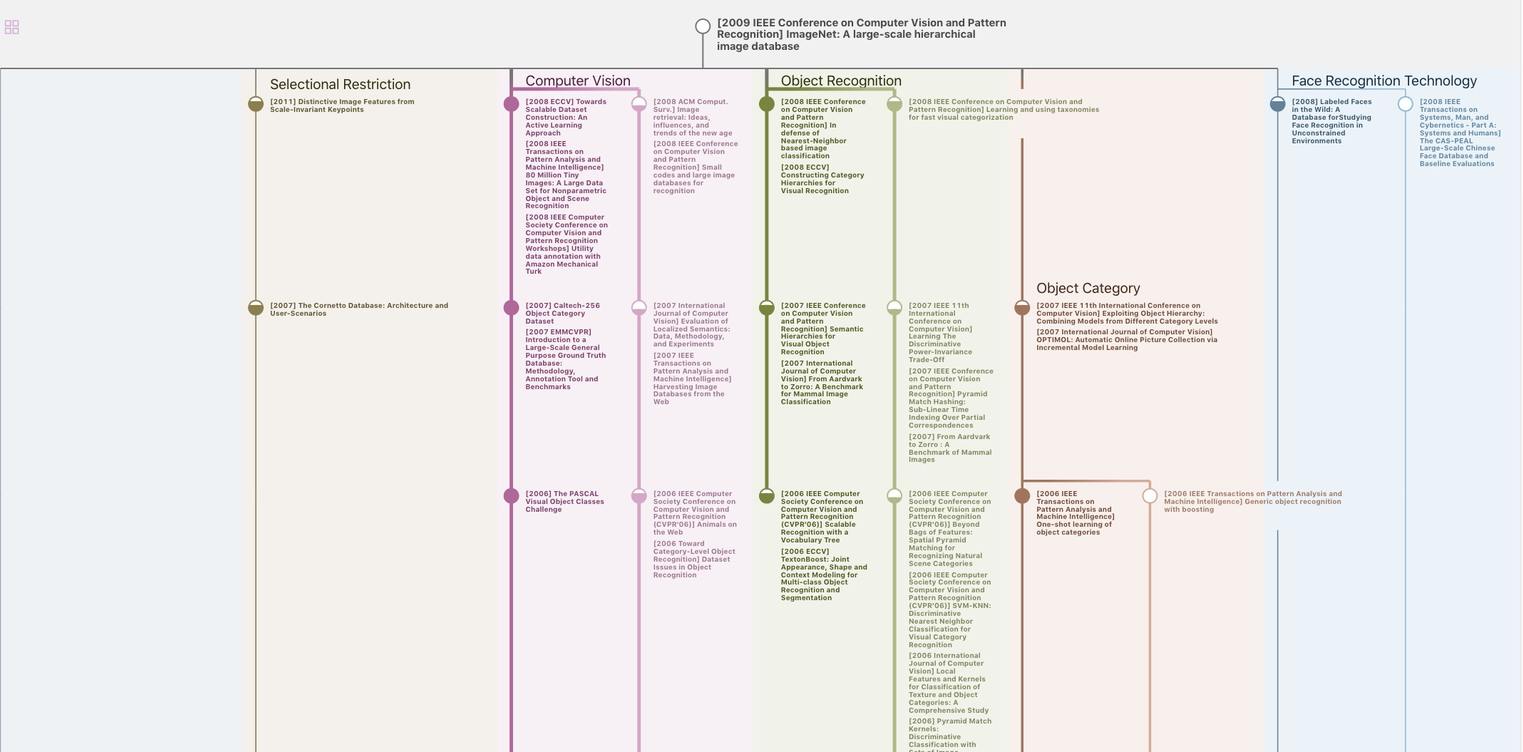
生成溯源树,研究论文发展脉络
Chat Paper
正在生成论文摘要