Sufficient Dimension Reduction with Deep Neural Networks for Phenotype Prediction
Proceedings of the 3rd International Conference on Statistics: Theory and ApplicationsInternational Conference on Statistics: Theory and Applications(2021)
摘要
Phenotype prediction with genome-wide SNPs or biomarkers is a difficult problem in biomedical research due to many issues, such as nonlinearity of the underlying genetic mapping, high-dimensionality of SNP data, and insufficiency of training samples.To tackle this difficulty, we propose a split-and-merge deep neural network (SM-DNN) method, which employs the split-and-merge technique on deep neural networks to obtain nonlinear sufficient dimension reduction of the input data and then learn a deep neural network on the dimension reduced data.We show that the DNN-based dimension reduction is sufficient, which retains all information on response contained in the explanatory data.Our numerical experiments indicate that the SM-DNN method can lead to significant improvement in phenotype prediction for a variety of real data examples.In particular, with only rare variants, we achieved a remarkable prediction accuracy of over 74% for the Early-Onset Myocardial Infarction (EOMI) exome sequence data.
更多查看译文
关键词
sufficient dimension reduction,deep neural networks,neural networks,prediction
AI 理解论文
溯源树
样例
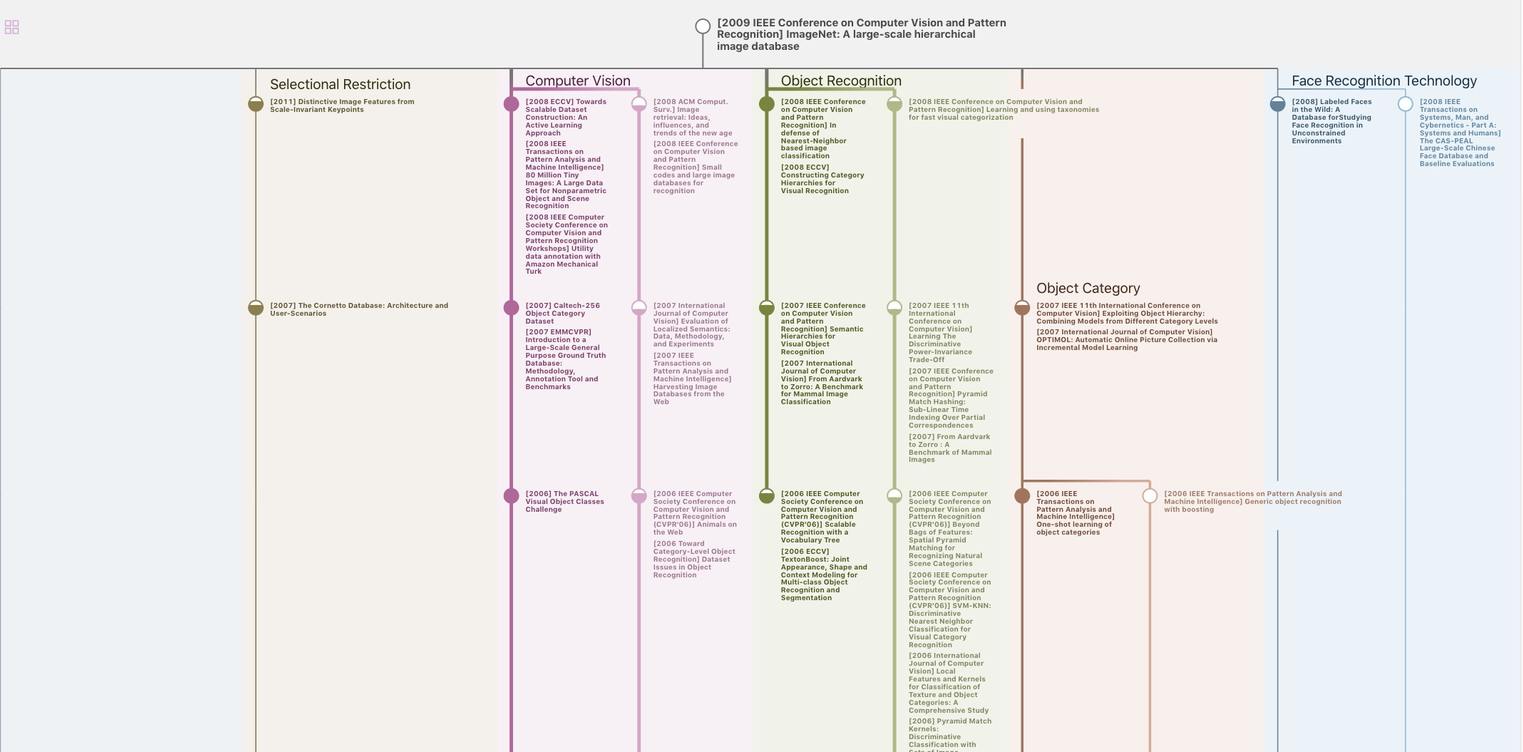
生成溯源树,研究论文发展脉络
Chat Paper
正在生成论文摘要