High spatial-temporal resolution land use regression models for ambient NO and NO2 concentrations in Accra Metropolis, Ghana
ISEE Conference Abstracts(2021)
摘要
BACKGROUND AND AIM: Increasing urban expansion and economic growth along with downward trend in household biomass use in sub-Saharan Africa (SSA) is shifting the dominant air pollution sources in cities from biomass to road traffic, with nitrogen oxides (NOx = NO + NO2) concentrations expected to be doubled by 2030 compared to 2012. Yet, there is scarcity of data on the spatial patterns and community distribution of NOx pollution in growing SSA cities. METHODS: In a measurement campaign between April 2019 to June 2020, we collected weekly integrated NOx and NO2 samples at 134 locations, consisting of yearlong and weeklong measurement sites across the Greater Accra Metropolitan Area (GAMA). The data were combined with land use and meteorological variables, providing both spatial and temporal information, to develop mixed-effect land use regression models (LUR) to predict NO and NO2 concentrations at 50m 50 m resolution across the GAMA. Stepwise forward approach was used to select the final models for the prediction. RESULTS:Our final models explained 80% and 88% of the variances (R2) in NO and NO2, respectively. The root-mean-square error (RMSE) and mean absolute error (MAE) following a 10-fold cross validation was 20.1 and 15.1 µg/m3 for NO, and 14.1 and 10.6 µg/m3 for NO2. Road traffic and meteorological variables were the most dominant predictors for both NO and NO2 models. CONCLUSIONS:Most of previous LUR models used solely spatial predictors and could only model annual concentrations for air pollutants. Our spatial-temporal integrated LUR models have really good performance for weekly NO and NOx concentrations with high spatial resolution. These models could provide high-resolution population exposure estimates of NO and NO2 for future study. KEYWORDS: nitrogen oxides, sub-Saharan Africa, traffic pollution, population exposure
更多查看译文
AI 理解论文
溯源树
样例
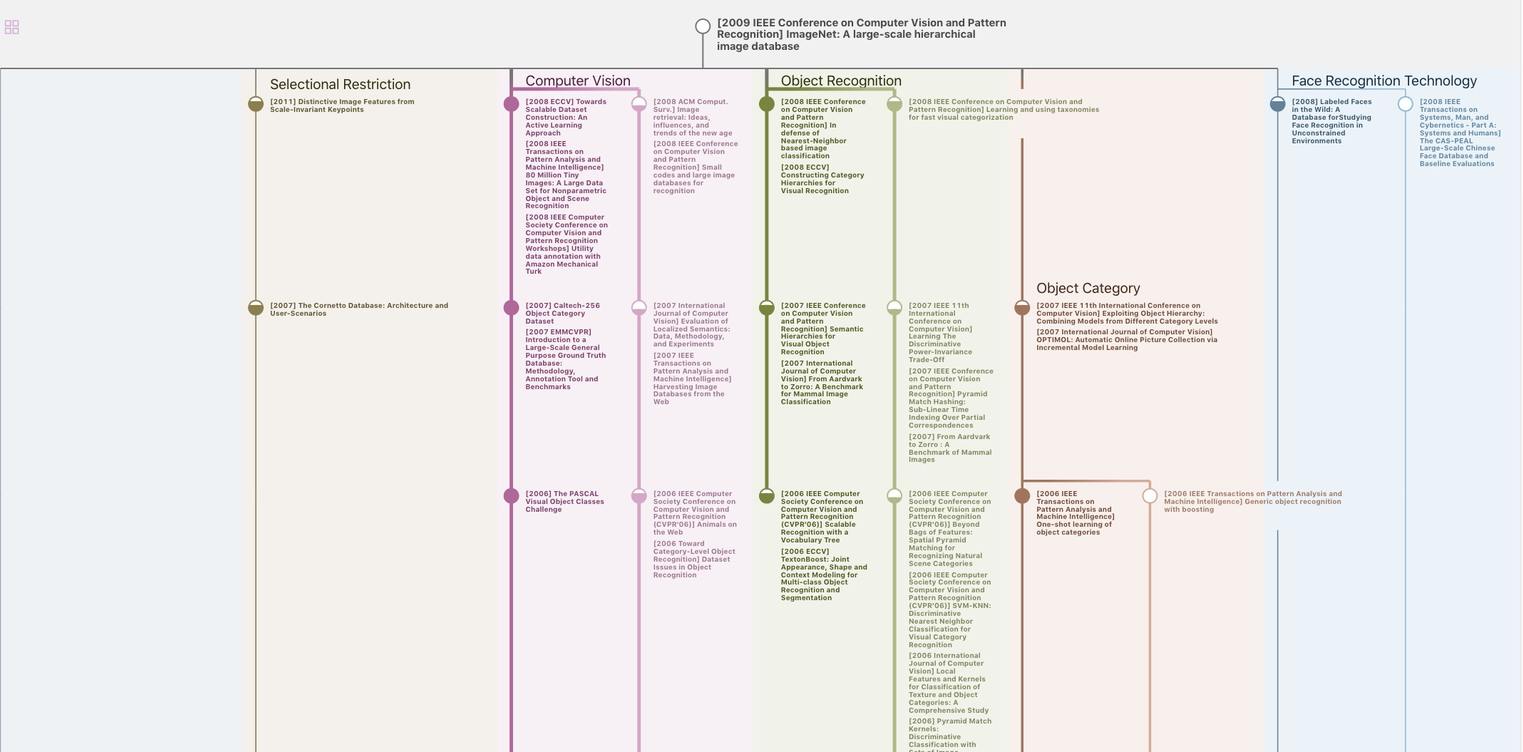
生成溯源树,研究论文发展脉络
Chat Paper
正在生成论文摘要