Using subgroup discovery and latent growth curve modeling to identify unusual developmental trajectories
Handbook of Computational Social Science, Volume 2(2021)
摘要
Latent growth curve models are frequently used statistical models to analyze longitudinal data and to gain insights into interindividual differences in growth trajectories. A key research question in this context is whether certain subgroups with unusual developmental trajectories exist. In this chapter, we introduce a new framework that integrates the modeling of growth curves with state-of-the art pattern mining techniques, that is, subgroup discovery and exceptional model mining, as an alternative to previous approaches such as growth mixture modeling (GMM) and structural equation model trees (SEM trees). This allows for flexibly and efficiently finding the interpretable subgroups of interest in large data sets. This exploratory data mining approach can help researchers to better understand heterogeneity in growth trajectories and to generate hypotheses for future research. We apply this approach in an empirical example to find subgroups of university students that show unusual developmental trajectories of dropout intentions.
更多查看译文
关键词
latent growth curve,subgroup discovery,modeling
AI 理解论文
溯源树
样例
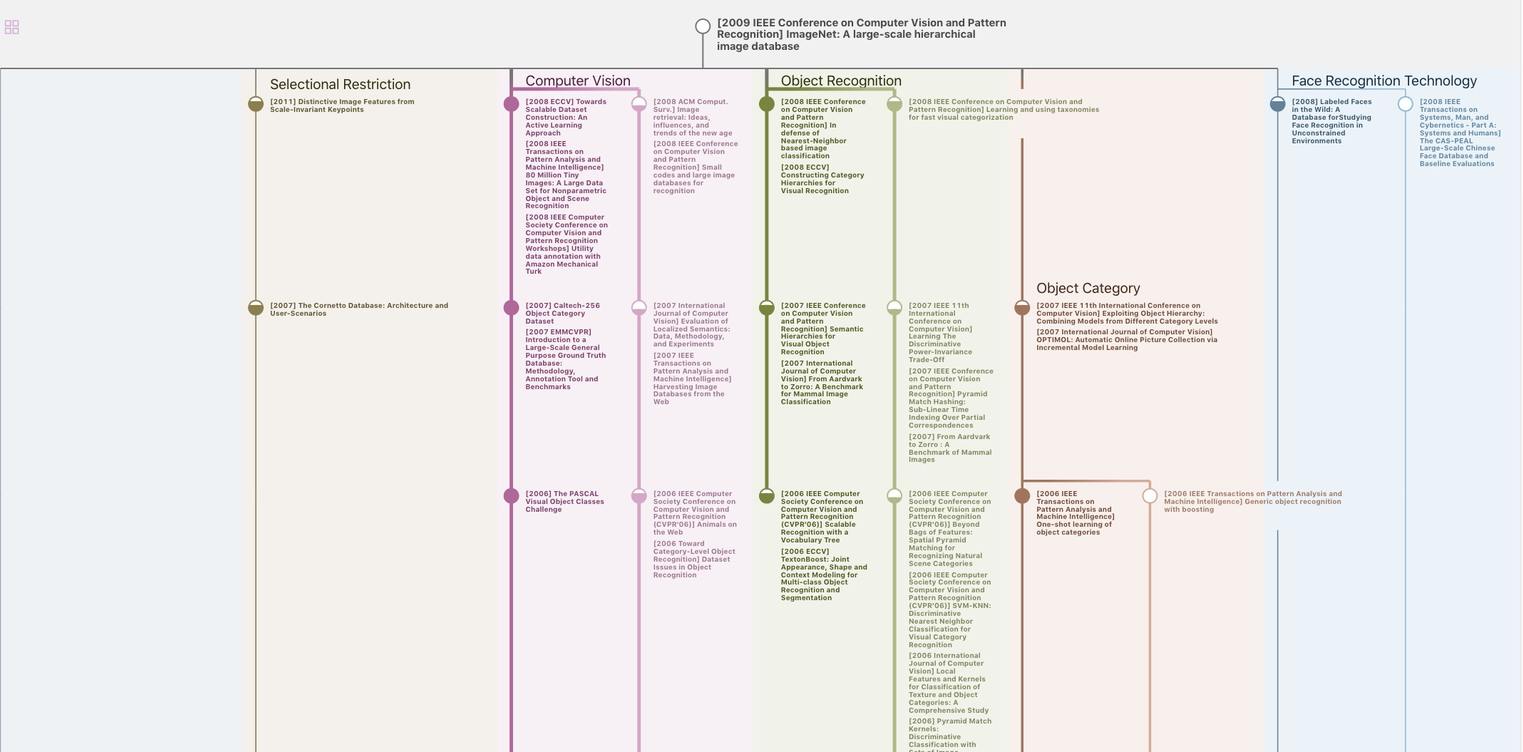
生成溯源树,研究论文发展脉络
Chat Paper
正在生成论文摘要