Category-Stitch Learning for Union Domain Generalization.
ACM Trans. Multim. Comput. Commun. Appl.(2023)
摘要
Domain generalization aims to generalize the network trained on multiple domains to unknown but related domains. Under the assumption that different domains share the same classes, previous works can build relationships across domains. However, in realistic scenarios, the change of domains is always followed by the change of categories, which raises a difficulty for collecting sufficient aligned categories across domains. Bearing this in mind, this paper introduces union domain generalization as a new domain generalization scenario, in which the label space varies across domains, and the categories in unknown domains belong to the union of all given domain categories. The absence of categories in given domains is the main obstacle to aligning different domain distributions and obtaining domain-invariant information. To address this problem, we propose category-stitch learning (CSL), which aims to jointly learn the domain-invariant information and complete missing categories in all domains through an improved variational autoencoder and generators. The domain-invariant information extraction and sample generation cross-promote each other to better generalizability. Additionally, we decouple category and domain information and propose explicitly regularizing the semantic information by the classification loss with transferred samples. Thus our method can breakthrough the category limit and generate samples of missing categories in each domain. Extensive experiments and visualizations are conducted on MNIST, VLCS, PACS, Office-Home, and DomainNet datasets to demonstrate the effectiveness of our proposed method.
更多查看译文
关键词
Domain generalization,variational autoencoder,generator
AI 理解论文
溯源树
样例
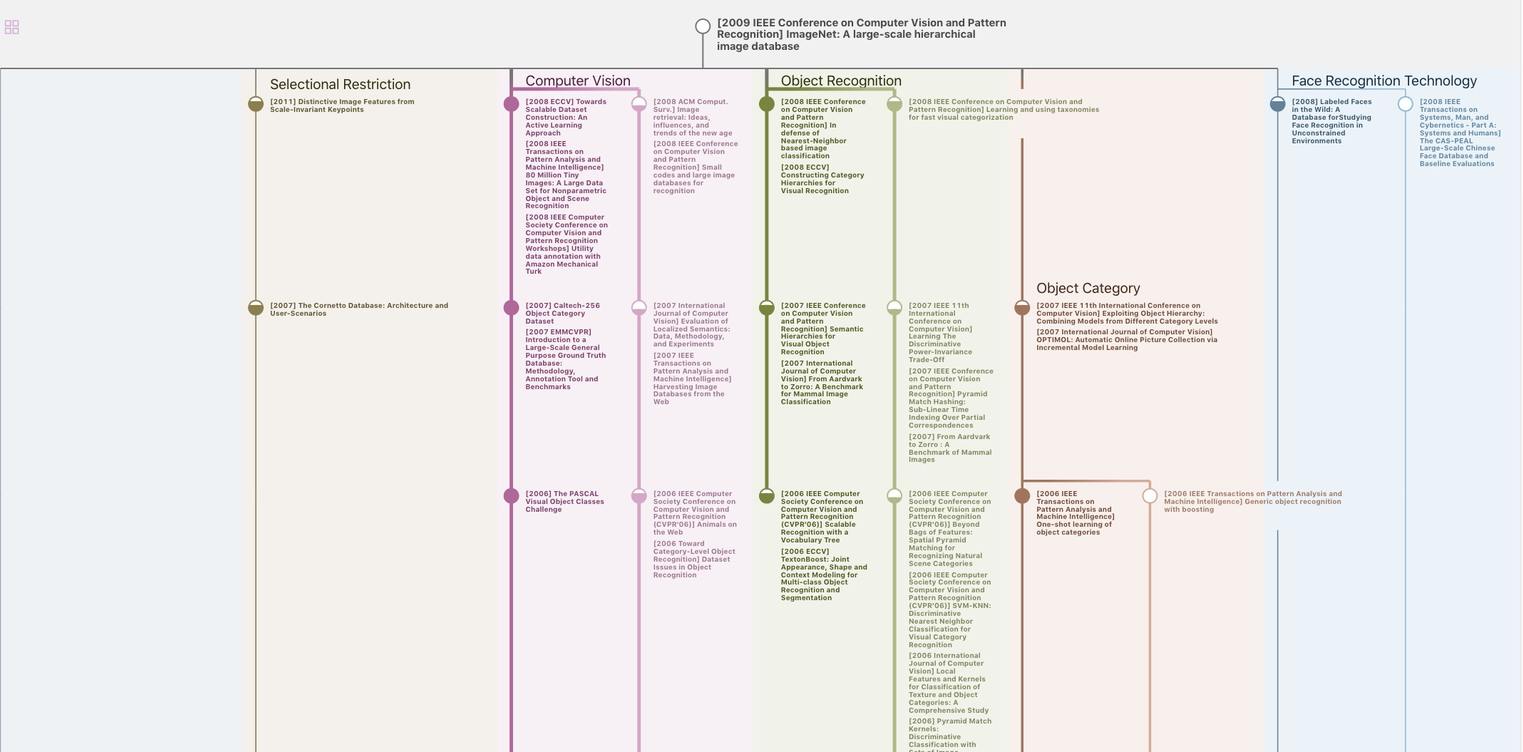
生成溯源树,研究论文发展脉络
Chat Paper
正在生成论文摘要