High-Performance UAV Crowdsensing: A Deep Reinforcement Learning Approach
IEEE Internet of Things Journal(2022)
摘要
Path planning is critical to realizing a high-performance unmanned aerial vehicle (UAV) crowdsensing system, which can be deployed to carry out large-scale tasks in the physical world, especially in emergency scenarios, such as earthquakes and mudslides. Deep reinforcement learning (DRL) has recently proven its superiority in path design. However, it is often applied under the assumption that the entire status of the target region is available, which is hard to achieve in practice. Instead, efforts should be made to ensure the efficient flight of several UAVs in order to collect data with incomplete observations in specified places. In this work, we set out to create a high-performance UAV crowdsensing system by combining DRL with partial observations. We present a novel DRL-based path-planning algorithm called DRL-PP. Specifically, we integrate an attention mechanism into the actor–critic technique to assist UAV swarm collaboration to collect data. We also design an incentive mechanism to ease the problem of sparse reward. Furthermore, we provide a dilemma detection system to prevent the generation of overlapping flight paths. Experimental results from extensive simulations prove that compared with the state-of-the-art approaches, the proposed DRL-PP can significantly improve the efficiency of data collection.
更多查看译文
关键词
Crowdsensing,deep reinforcement learning (DRL),Markov decision process (MDP),unmanned aerial vehicle (UAV)
AI 理解论文
溯源树
样例
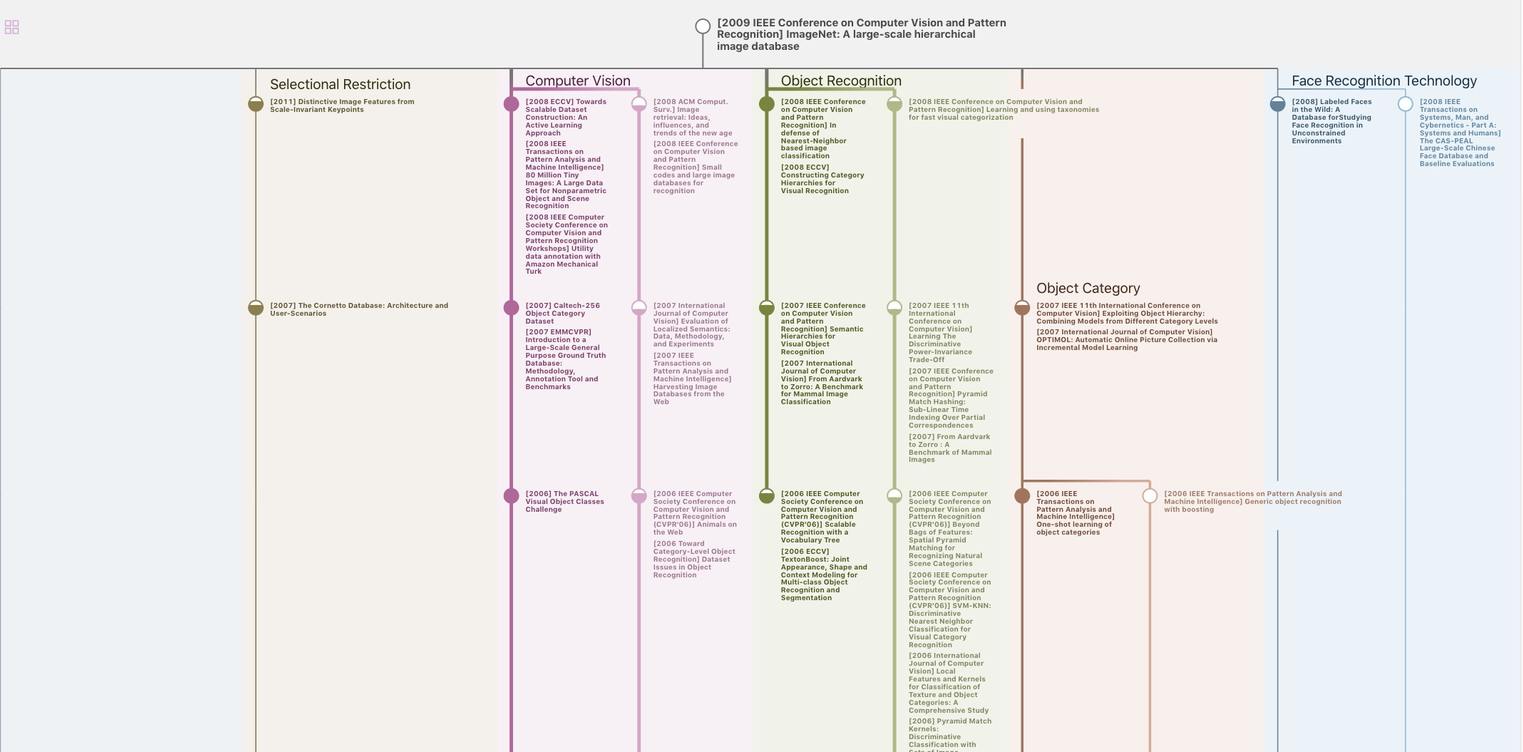
生成溯源树,研究论文发展脉络
Chat Paper
正在生成论文摘要