Generation of Black-box Audio Adversarial Examples Based on Gradient Approximation and Autoencoders
ACM Journal on Emerging Technologies in Computing Systems(2022)
摘要
Deep Neural Network (DNN) is gaining popularity thanks to its ability to attain high accuracy and performance in various security-crucial scenarios. However, recent research shows that DNN based Automatic Speech Recognition (ASR) systems are vulnerable to adversarial attacks. Specifically, these attacks mainly focus on formulating a process of adversarial example generation as iterative, optimization-based attacks. Although these attacks make significant progress, they still take large generation time to produce adversarial examples, which makes them difficult to be launched in real-world scenarios. In this paper, we propose a real-time attack framework that utilizes the neural network trained by the gradient approximation method to generate adversarial examples on Keyword Spotting (KWS) systems. The experimental results show that these generated adversarial examples can easily fool a black-box KWS system to output incorrect results with only one inference. In comparison to previous works, our attack can achieve a higher success rate with less than 0.004 seconds. We also extend our work by presenting a novel ensemble audio adversarial attack and testing the attack on KWS systems equipped with existing defense mechanisms. The efficacy of the proposed attack is well supported by promising experimental results.
更多查看译文
关键词
Adversarial examples,deep neural network,automatic speech recognition
AI 理解论文
溯源树
样例
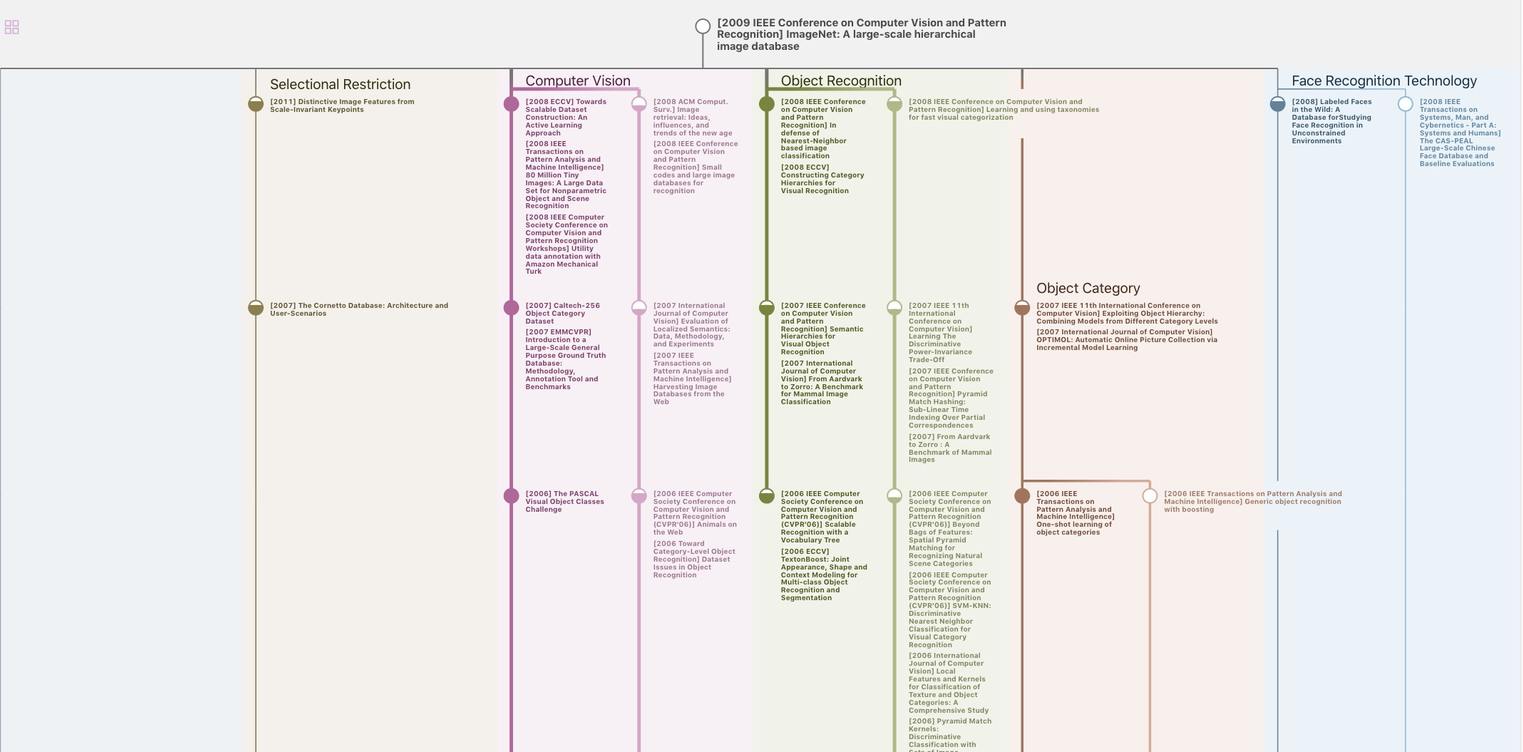
生成溯源树,研究论文发展脉络
Chat Paper
正在生成论文摘要