Form Follows Function
Informatik aktuellBildverarbeitung für die Medizin 2022(2022)
摘要
In this work, we construct and train a learning-based neural network pipeline for retinal vessel segmentation on fundus images and transfer the preprocessing module directly onto OCTA en face projections. Without additional fine-tuning, the transferred module retains the edge-preserving denoising functionality, as our smart network design enables zero-shot domain adaptation. Compared to a denoising network trained on OCTA data, the transferred preprocessing module is superior with regard to performance, generalization ability, and numerical stability. In addition, Frangi’s vessel segmentation of the preprocessed images outperforms the predictions using pretrained full segmentation networks. The selection of fitting Gaussian scales of the Frangi filter in the target domain can bypass the over/under-segmentation problem caused by the vessel diameter mismatch between the two domains. The contribution of this work is two-fold: we provide an exemplary evidence that smart design of network pipelines allows for flexible functional module reuse across different imaging modalities, i.e. zero-shot domain adaptation; and we discover a deep learning-based vessel segmentation pipeline for OCTA en face images which reaches an AUC score of 0.9418 without additional training.
更多查看译文
关键词
form,function
AI 理解论文
溯源树
样例
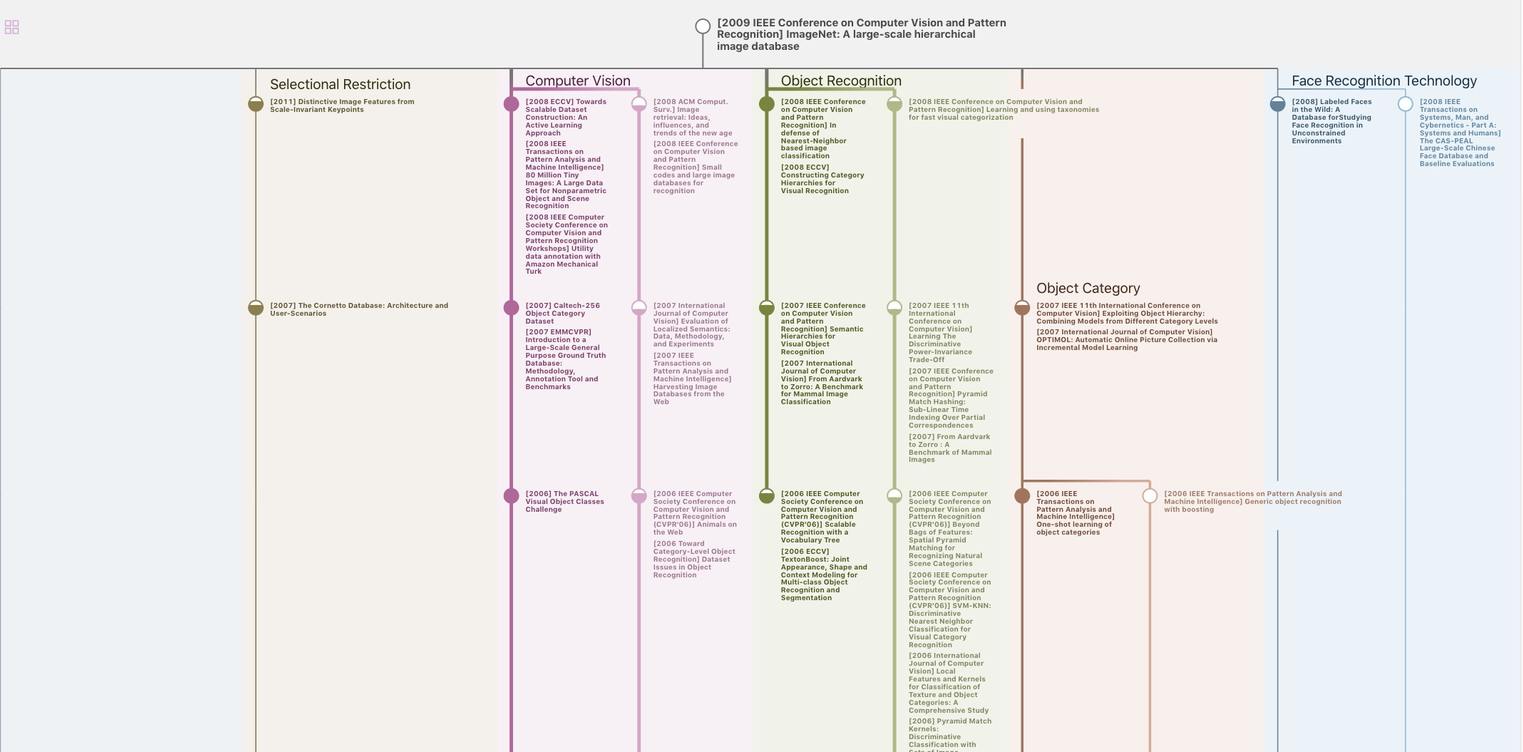
生成溯源树,研究论文发展脉络
Chat Paper
正在生成论文摘要