A Comparative Study on Part-of-Speech Taggers’ Performance on Examination Questions Classification According to Bloom’s Taxonomy
Journal of Physics: Conference Series(2022)
摘要
Abstract Examination questions classification according to Bloom’s Taxonomy uses Natural Language Processing (NLP) approach, a series of text processing approach that generally can divided into the keywords identification stage and then the identified keywords classification to Bloom’s Taxonomy levels stage. Since this NLP approach is a pipeline processes, the keywords identification stage’s performance in term of accuracy is affecting the subsequent stage - the identified keywords classification and subsequently limits the final accuracy performance of the questions classification. The keywords identification stage’s performance is mainly depending on the effectiveness of Part-Of-Speech (POS) tagging. Thus, this paper aims to identify the best performing POS tagger in keywords identification stage and enhance the tagger’s performance with rule-based approach to achieve high accuracy performance and benefit the subsequent keyword classification and then the questions classification accuracy. The Perceptron tagger and the Stanford POS tagger are selected to be evaluated their performance in identifying the keywords of the randomly selected 200 examination questions from STEM subjects. This paper has observed the Stanford POS tagger is the best performing tagger in POS tagging with accuracy of 80.5%. Some rules are applied to the POS tagging to improve the accuracy further to 91.5%.
更多查看译文
AI 理解论文
溯源树
样例
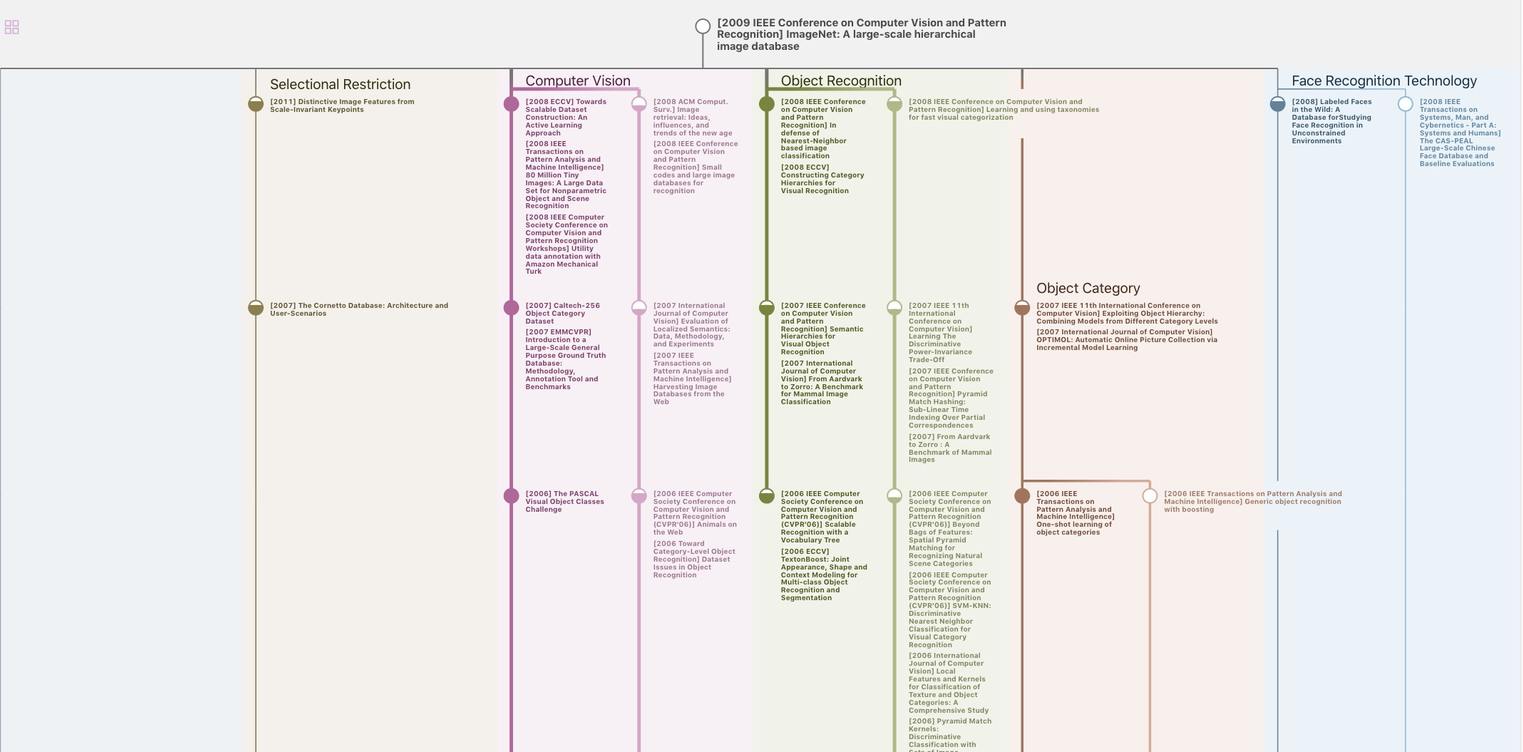
生成溯源树,研究论文发展脉络
Chat Paper
正在生成论文摘要