Dataset Similarity to Assess Semisupervised Learning Under Distribution Mismatch Between the Labeled and Unlabeled Datasets.
IEEE Transactions on Artificial Intelligence(2023)
摘要
Semisupervised deep learning (SSDL) is a popular strategy to leverage unlabeled data for machine learning when labeled data is not readily available. In real-world scenarios, different unlabeled data sources are usually available, with varying degrees of distribution mismatch regarding the labeled datasets. It begs the question, which unlabeled dataset to choose for good SSDL outcomes. Oftentimes, semantic heuristics are used to match unlabeled data with labeled data. However, a quantitative and systematic approach to this selection problem would be preferable. In this work, we first test the SSDL MixMatch algorithm under various distribution mismatch configurations to study the impact on SSDL accuracy. Then, we propose a quantitative unlabeled dataset selection heuristic based on dataset dissimilarity measures. These are designed to systematically assess how distribution mismatch between the labeled and unlabeled datasets affects MixMatch performance. We refer to our proposed method as deep dataset dissimilarity measures (DeDiMs), designed to compare labeled and unlabeled datasets. They use the feature space of a generic Wide-ResNet, which can be applied prior to learning, are quick to evaluate, and model agnostic. The strong correlation in our tests between MixMatch accuracy and the proposed DeDiMs suggests that this approach can be a good fit for quantitatively ranking different unlabeled datasets prior to SSDL training.
更多查看译文
关键词
Dataset similarity,deep learning,distribution mismatch,MixMatch,out of distribution data,semisupervised deep learning
AI 理解论文
溯源树
样例
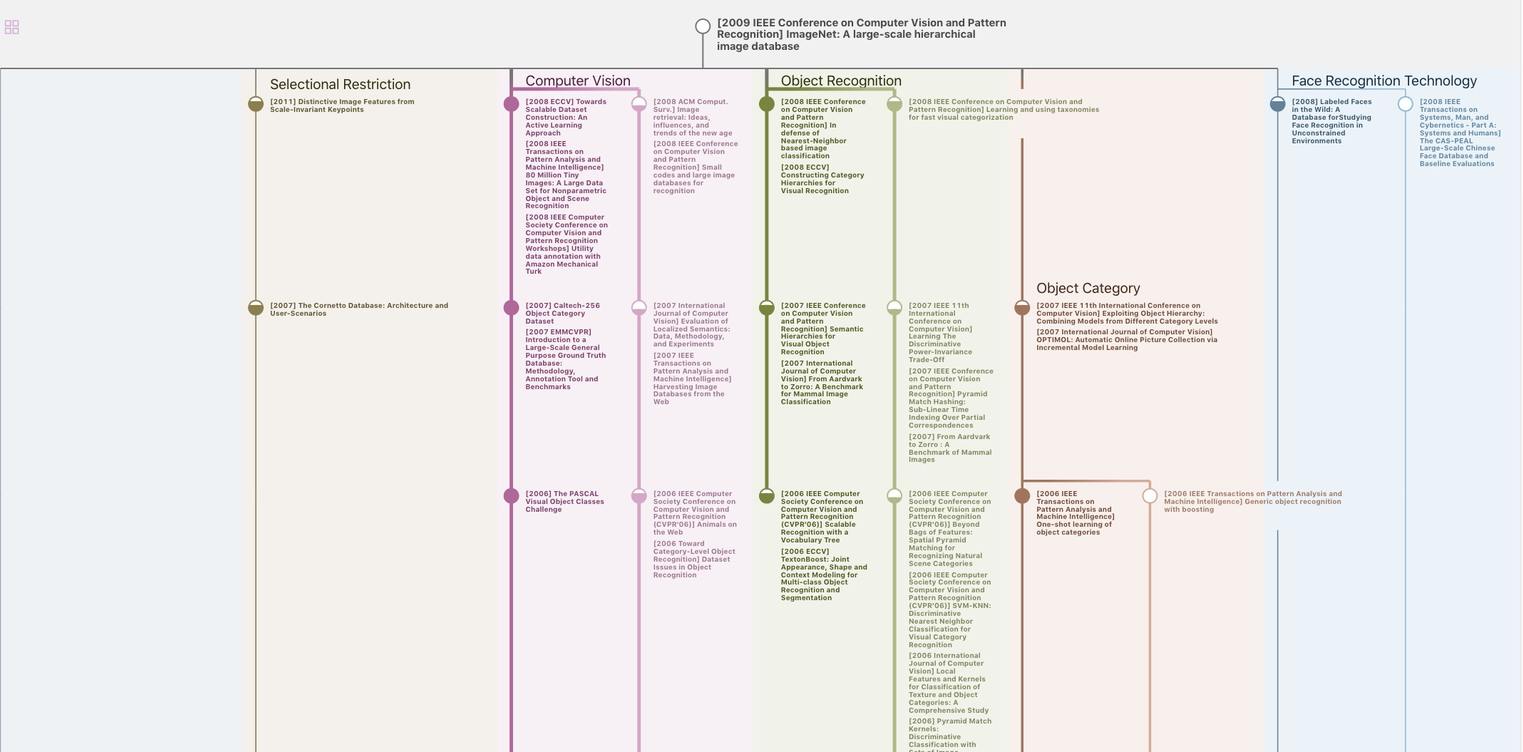
生成溯源树,研究论文发展脉络
Chat Paper
正在生成论文摘要