Point Cloud Video Super-Resolution via Partial Point Coupling and Graph Smoothness
IEEE TRANSACTIONS ON IMAGE PROCESSING(2022)
摘要
Point cloud (PC) is a collection of discrete geometric samples of a physical object in 3D space. A PC video consists of temporal frames evenly spaced in time, each containing a static PC at one time instant. PCs in adjacent frames typically do not have point-to-point (P2P) correspondence, and thus exploiting temporal redundancy for PC restoration across frames is difficult. In this paper, we focus on the super-resolution (SR) problem for PC video: increase point density of PCs in video frames while preserving salient geometric features consistently across time. We accomplish this with two ideas. First, we establish partial P2P coupling between PCs of adjacent frames by interpolating interior points in a low-resolution PC patch in frame t and translating them to a corresponding patch in frame t + 1, via a motion model computed by iterative closest point (ICP). Second, we promote piecewise smoothness in 3D geometry in each patch using feature graph Laplacian regularizer (FGLR) in an easily computable quadratic form. The two ideas translate to an unconstrained quadratic programming (QP) problem with a system of linear equations as solution-one where we ensure the numerical stability by upper-bounding the condition number of the coefficient matrix. Finally, to improve the accuracy of the ICP motion model, we re-sample points in a super-resolved patch at time t to better match a low-resolution patch at time t + 1 via bipartite graph matching after each SR iteration. Experimental results show temporally consistent super-resolved PC videos generated by our scheme, outperforming SR competitors that optimized on a per-frame basis, in two established PC metrics.
更多查看译文
关键词
3D point cloud, graph signal processing, super resolution, numerical linear algebra
AI 理解论文
溯源树
样例
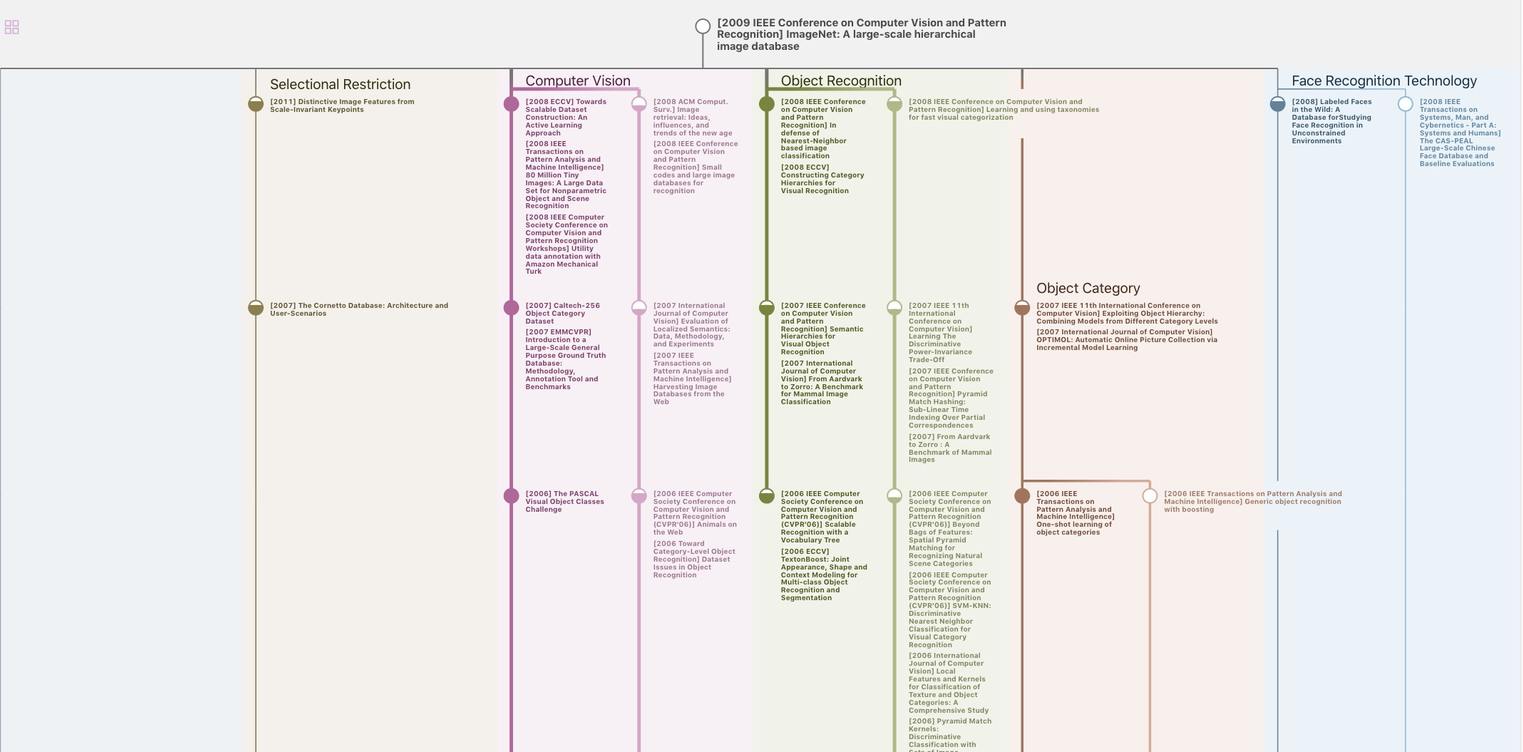
生成溯源树,研究论文发展脉络
Chat Paper
正在生成论文摘要