In Defense of Core-set: A Density-aware Core-set Selection for Active Learning
KDD '22: Proceedings of the 28th ACM SIGKDD Conference on Knowledge Discovery and Data Mining(2022)
摘要
Active learning enables the efficient construction of a labeled dataset by labeling informative samples from an unlabeled dataset. In a real-world active learning scenario, the use of diversity-based sampling is indispensable because there are many redundant or highly similar samples. Core-set approach is the promising diversity-based method selecting diverse samples by considering the distance between samples. However, the approach poorly performs compared to the uncertainty-based method that selects the most difficult samples where neural models reveal low confidence. In this work, we analyze the feature space through the lens of density and, interestingly, observe that locally sparse regions tend to have more informative samples than dense regions. Motivated by our analysis, we empower the core-set approach with the density-awareness and propose a density-aware core-set (DACS) which estimates the density of the unlabeled samples and selects diverse samples mainly from sparse regions which are treated as the informative regions. To reduce the computational bottlenecks in estimating the density, we introduce a new density approximation based on locality-sensitive hashing. Experimental results demonstrate the efficacy of DACS in both classification and regression tasks and specifically show that DACS can produce state-of-the-art performance in a practical scenario. Since DACS is weakly dependent on architectures, we also present a simple yet effective combination method to show that the existing methods can be beneficially combined with DACS.
更多查看译文
关键词
selection,learning,active,core-set,density-aware,core-set
AI 理解论文
溯源树
样例
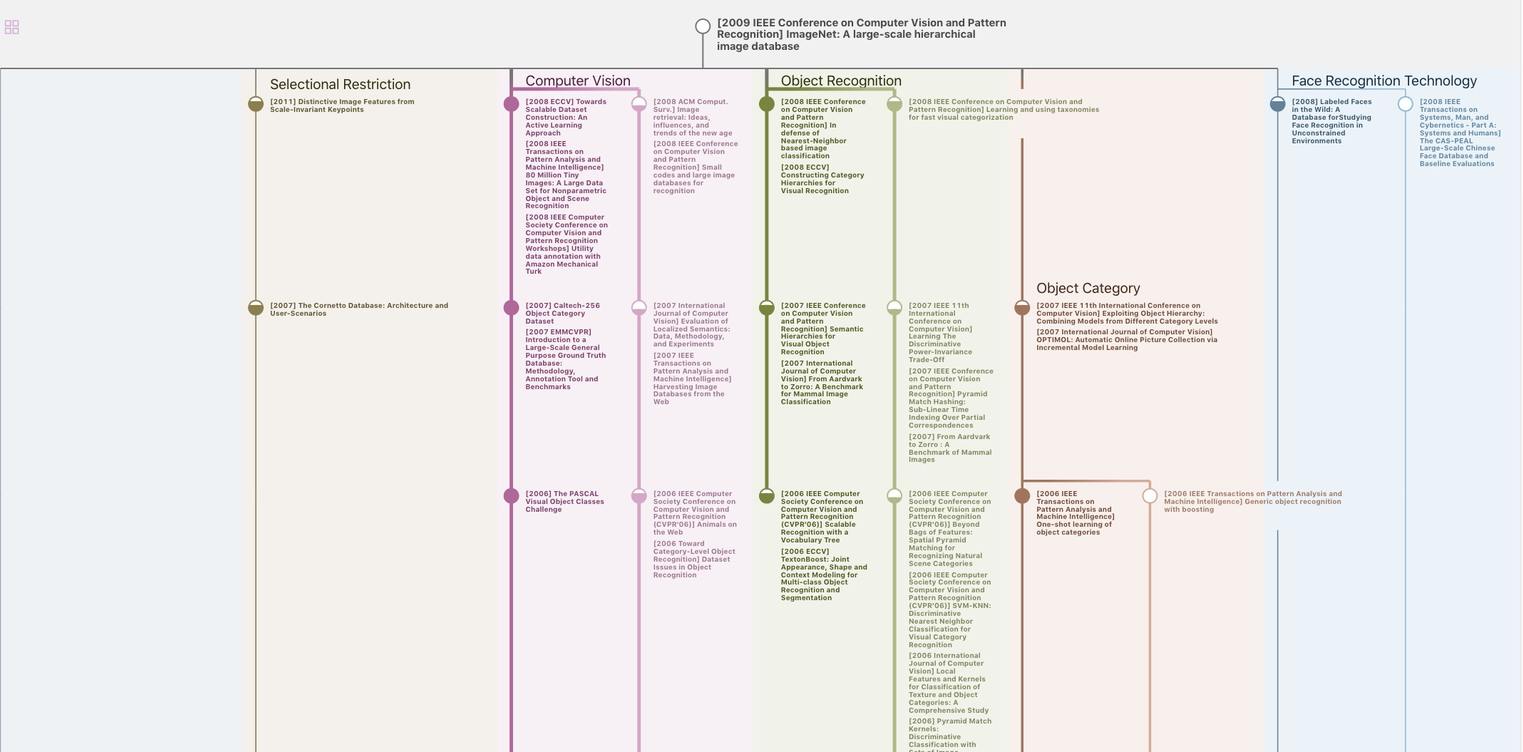
生成溯源树,研究论文发展脉络
Chat Paper
正在生成论文摘要