Open Challenges in Developing Generalizable Large Scale Machine Learning Models for Catalyst Discovery
ACS CATALYSIS(2022)
摘要
The development of machine learned potentials for catalyst discovery has predominantly been focused on very specific chemistries and material compositions. While effective in interpolating between available materials, these approaches struggle to generalize across chemical space. The recent curation of large-scale catalyst datasets has offered the opportunity to build a universal machine learning potential, spanning chemical and composition space. If accomplished, said potential could accelerate the catalyst discovery process across a variety of applications (CO2 reduction, NH3 production, etc.) without additional specialized training efforts that are currently required. The release of the Open Catalyst 2020 (OC20) has begun just that, pushing the heterogeneous catalysis and machine learning communities towards building more accurate and robust models. In this perspective, we discuss some of the challenges and findings of recent developments on OC20. We examine the performance of current models across different materials and adsorbates to identify notably underperforming subsets. We then discuss some of the modeling efforts surrounding energy-conservation, approaches to finding and evaluating the local minima, and augmentation of off-equilibrium data. To complement the community's ongoing developments, we end with an outlook to some of the important challenges that have yet to be thoroughly explored for large-scale catalyst discovery.
更多查看译文
关键词
machine-learning potentials,graph neural networks,catalyst discovery,high-throughput screening,renewable energy
AI 理解论文
溯源树
样例
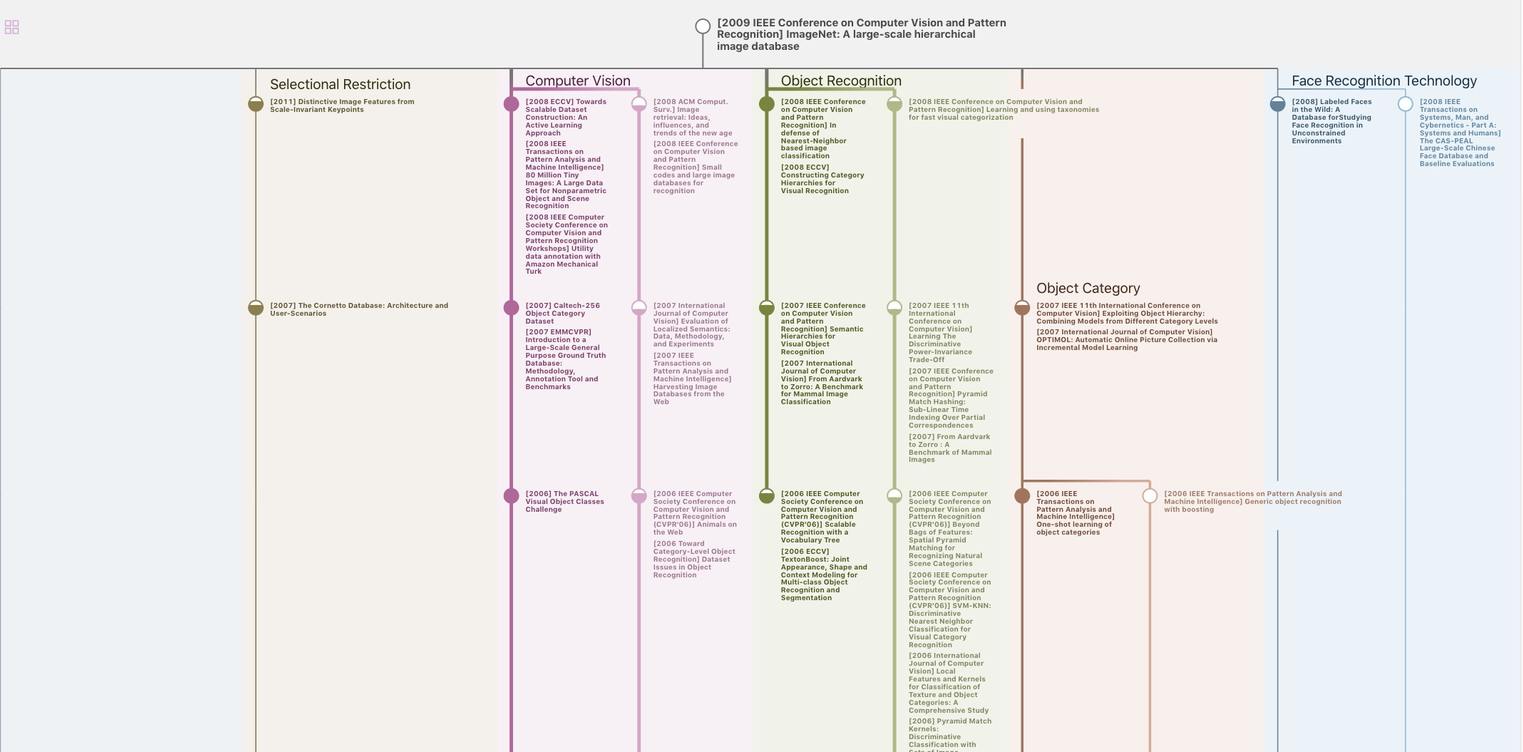
生成溯源树,研究论文发展脉络
Chat Paper
正在生成论文摘要